Artificial Intelligence Applications in Petroleum Supply and Management
A special issue of Energies (ISSN 1996-1073). This special issue belongs to the section "H1: Petroleum Engineering".
Deadline for manuscript submissions: closed (30 May 2023) | Viewed by 22834
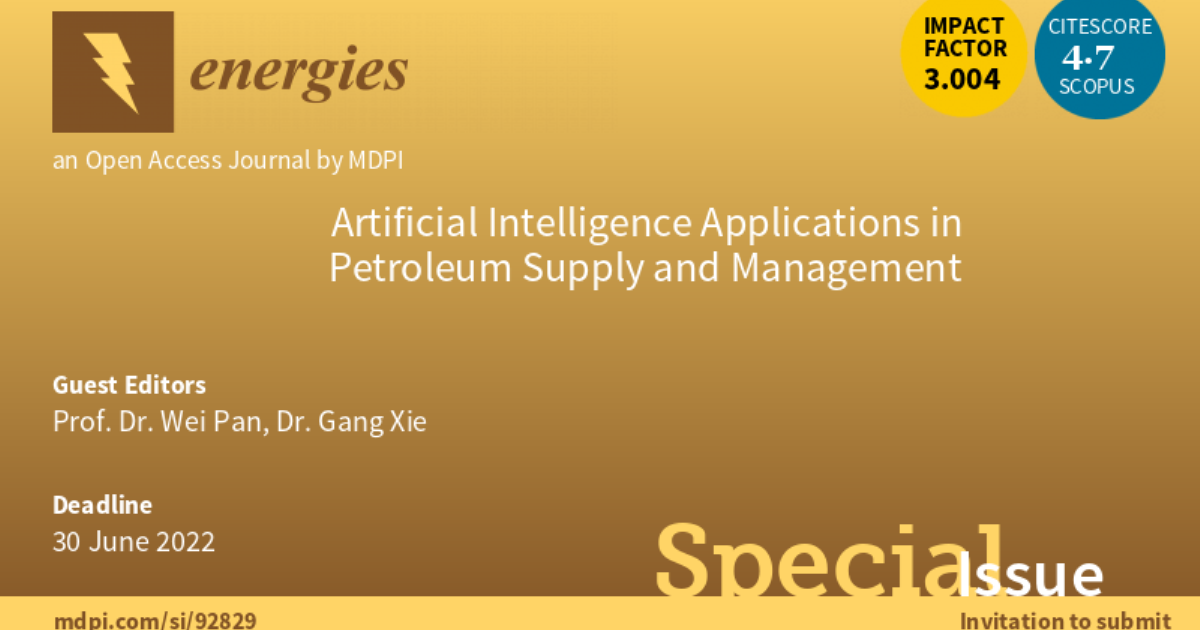
Special Issue Editors
Interests: artificial intelligence; digital economy; input–output theory; environment and health; risk management; energy optimization
Special Issues, Collections and Topics in MDPI journals
Interests: artificial intelligence; economic forecasting; sustainable supply chain management; risk management; energy optimization
Special Issues, Collections and Topics in MDPI journals
Special Issue Information
Dear Colleagues,
I would like to invite you to contribute to a Special Issue of Energies on “Artificial Intelligence Applications in Petroleum Supply and Management”.
At present, the application of artificial intelligence in the petroleum industry is far from extensive, as it is still in the primary stage of exploration. However, new technologies are introducing a new era in the field, such as integrated sensors, augmented reality (AR), virtual reality (VR), artificial intelligence (AI), and unmanned driving, which have brought great challenges and possibilities to the “very traditional” oil industry. For example, unmanned aerial vehicles (UAVs) have begun to be used to monitor and manage the safety and efficiency of oil equipment operations, while smart sensors and satellite imaging technology are helping operators to manage and operate remote well sites in real time, reducing the risk of long-term isolation.
Technologies such as COMOS and Walkinside are also helping to create 3D visualizable virtual oil platforms that digitally capture real-time information about each component, enabling operators to manage and monitor efficiently and accurately without ever having to step foot on the platform. These activities require relevant supporting technologies, such as the Internet of Things (IoT), information and communication technology (ICT), and 5G. Additionally, with the continued use of conventional oil for nearly a hundred years and with most of the remaining recoverable oil and gas resources in the deep sea, the polar region where no human life is present, the application of artificial intelligence can greatly help humankind to achieve economic security for such unconventional oil and gas development and reduce casualties and supply costs, while at the same time reducing the pollution of the environment through oil development.
A range of tools based on artificial intelligence, simulation integration, and automated data analysis are being developed and increasingly used. Artificial intelligence is undoubtedly an epoch-making leap in software development. It can constantly store, learn, and analyze data to help to improve the accuracy of our future predictions, and it can help more enterprises to use big data technology and analyze data to gain more a strategic understanding.
As petroleum is the most widely used and highly efficient energy raw material, its supply and management play a huge role in the survival and development of petroleum enterprises. However, with the gradual depletion of petroleum, the remaining recoverable resources are primarily located in areas beyond human reach, such as the deep sea and the polar regions. The development of artificial intelligence (AI) is a rare opportunity for petroleum enterprises. Firstly, with the help of AI technology, petroleum enterprises can effectively control and optimize the production process, improve production efficiency, and reduce energy consumption and material consumption. Secondly, AI technology can help petroleum enterprises to introduce safety management services, allowing machines to replace people in inspecting safety hazards, forecasting safety risks, ensuring installations’ safe operation, and preventing safety accidents.
This Special Issue aims to introduce the application of AI technology, such as machine learning, in petroleum supply and management. The ultimate goal is to identify the most promising approaches for each of the current and future challenges in the petroleum sector and provide readers with a set of concrete applications of AI. These applications are not only conducive to the transformation of the petroleum industry but also of great significance to promoting energy conservation and emission reduction for the whole industry.
Prof. Dr. Wei Pan
Dr. Gang Xie
Guest Editors
Manuscript Submission Information
Manuscripts should be submitted online at www.mdpi.com by registering and logging in to this website. Once you are registered, click here to go to the submission form. Manuscripts can be submitted until the deadline. All submissions that pass pre-check are peer-reviewed. Accepted papers will be published continuously in the journal (as soon as accepted) and will be listed together on the special issue website. Research articles, review articles as well as short communications are invited. For planned papers, a title and short abstract (about 100 words) can be sent to the Editorial Office for announcement on this website.
Submitted manuscripts should not have been published previously, nor be under consideration for publication elsewhere (except conference proceedings papers). All manuscripts are thoroughly refereed through a single-blind peer-review process. A guide for authors and other relevant information for submission of manuscripts is available on the Instructions for Authors page. Energies is an international peer-reviewed open access semimonthly journal published by MDPI.
Please visit the Instructions for Authors page before submitting a manuscript. The Article Processing Charge (APC) for publication in this open access journal is 2600 CHF (Swiss Francs). Submitted papers should be well formatted and use good English. Authors may use MDPI's English editing service prior to publication or during author revisions.
Keywords
- AI in petroleum supply systems
- AI in petroleum management systems
- AI in energy storage
- energy forecast
- energy system
- energy consumption
- energy optimization
- sustainability
- artificial intelligence (AI)
- Internet of Things (IoT)
- Information and communication technology (ICT)
- big data
- 5G
- intelligent control
- machine learning
- deep learning
Benefits of Publishing in a Special Issue
- Ease of navigation: Grouping papers by topic helps scholars navigate broad scope journals more efficiently.
- Greater discoverability: Special Issues support the reach and impact of scientific research. Articles in Special Issues are more discoverable and cited more frequently.
- Expansion of research network: Special Issues facilitate connections among authors, fostering scientific collaborations.
- External promotion: Articles in Special Issues are often promoted through the journal's social media, increasing their visibility.
- Reprint: MDPI Books provides the opportunity to republish successful Special Issues in book format, both online and in print.
Further information on MDPI's Special Issue policies can be found here.