Deep Learning in Bioinformatics and Biomedicine
A special issue of Applied Sciences (ISSN 2076-3417). This special issue belongs to the section "Applied Biosciences and Bioengineering".
Deadline for manuscript submissions: closed (20 October 2022) | Viewed by 9984
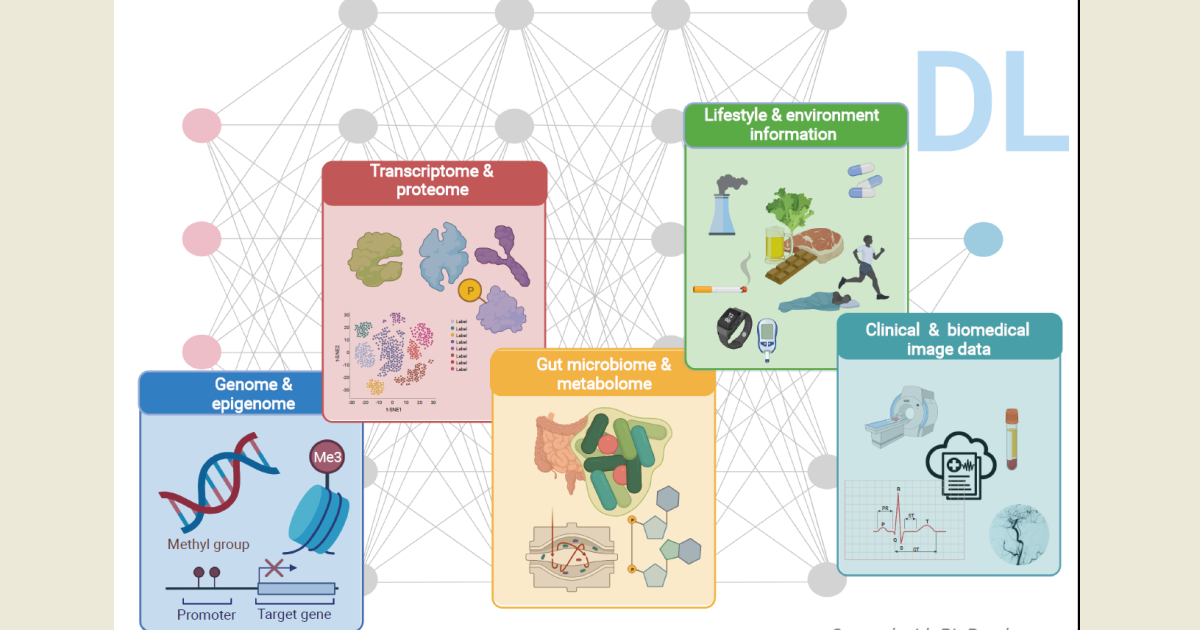
Special Issue Editor
Special Issue Information
Dear Colleagues,
The Special Issue on The Deep Learning in Bioinformatics and Biomedicine is dedicated to discussing recent developments in the field of deep learning (DL) and its applications in bioinformatics and biomedicine ranging from the analyses of biomedical images to omics (genome, transcriptome, proteome, metabolome, and microbiome) and healthcare data.
DL is a subfield of machine learning, concerned with algorithms that are neural implementations with multiple processing layers, inspired by the structure and function of the human brain to learn and make intelligent decisions. DL approaches are designed for supervised classification, requiring input–output mapping that has to be learned from large amounts of labeled training data and requires the power of parallel and distributed computing. With the advent of the big data era, fueled by the rapid development of high-throughput technologies and digitalization of health records, exponentially increasing volumes of complex data are accumulating and becoming widely available, and their transformation into valuable knowledge is urgently required, especially in the context of personalized medicine and disease risk prediction to improve therapies for patients.
DL is believed to have the necessary transformative impact for the field and has recently caught the interest of both academia and industry, with the number of publications describing its application in bioinformatics and biomedicine steadily increasing, especially in the last 5 years. DL has been applied for a broad range of tasks, mostly the analysis of medical images, e.g., for the detection of cancer metastases. In genomics, DL has been used to prioritize potential disease-causing genetic variants and for the splice junction prediction. DL has also been recognized as more suitable to deal with electronic health records, which contain individual’s entire medical history as a series of multimodal data. Overall, the capabilities of DL for bioinformatics and biomedicine are not yet fully exploited, and future progress is expected to come, for example, from improving the encoding and learning from the raw data instead of using hand-crafted features, requiring domain expertise.
In this Special Issue, we invite submissions presenting or exploring cutting-edge research, recent advances, and innovative ideas in the area of DL for the fields of bioinformatics and biomedicine. Studies from both academia and industry analyzing biomedical images, omics (genome, transcriptome, proteome, metabolome, and microbiome) and/or healthcare data are welcome, as well as comprehensive review papers elaborating on the topic.
Dr. Baiba Vilne
Guest Editor
Manuscript Submission Information
Manuscripts should be submitted online at www.mdpi.com by registering and logging in to this website. Once you are registered, click here to go to the submission form. Manuscripts can be submitted until the deadline. All submissions that pass pre-check are peer-reviewed. Accepted papers will be published continuously in the journal (as soon as accepted) and will be listed together on the special issue website. Research articles, review articles as well as short communications are invited. For planned papers, a title and short abstract (about 100 words) can be sent to the Editorial Office for announcement on this website.
Submitted manuscripts should not have been published previously, nor be under consideration for publication elsewhere (except conference proceedings papers). All manuscripts are thoroughly refereed through a single-blind peer-review process. A guide for authors and other relevant information for submission of manuscripts is available on the Instructions for Authors page. Applied Sciences is an international peer-reviewed open access semimonthly journal published by MDPI.
Please visit the Instructions for Authors page before submitting a manuscript. The Article Processing Charge (APC) for publication in this open access journal is 2400 CHF (Swiss Francs). Submitted papers should be well formatted and use good English. Authors may use MDPI's English editing service prior to publication or during author revisions.
Keywords
- machine learning
- deep learning
- big data
- biomedicine
- bioinformatics
- biomedical imaging
- (multi)-omics
- genomics
- transcriptomics
- epigenomics
- proteomics
- metabolomics
- metagenomics
- health-care data
- disease risk prediction
- personalized medicine
Benefits of Publishing in a Special Issue
- Ease of navigation: Grouping papers by topic helps scholars navigate broad scope journals more efficiently.
- Greater discoverability: Special Issues support the reach and impact of scientific research. Articles in Special Issues are more discoverable and cited more frequently.
- Expansion of research network: Special Issues facilitate connections among authors, fostering scientific collaborations.
- External promotion: Articles in Special Issues are often promoted through the journal's social media, increasing their visibility.
- Reprint: MDPI Books provides the opportunity to republish successful Special Issues in book format, both online and in print.
Further information on MDPI's Special Issue policies can be found here.