Topic Menu
► Topic MenuTopic Editors

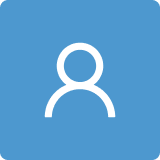
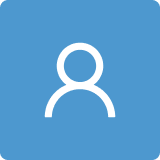

Progress in Satellite Remote Sensing of Land Surface: New Algorithms, Sensors and Datasets
Topic Information
Dear Colleagues,
In scientific research on Earth systems, satellite remote sensing plays the most important role in quantifying land surface states, which are represented by soil moisture, land surface temperature, vegetation, snow cover, water bodies, and glaciers. In addition to providing direct support for various industrial applications, it can also provide vital input data for researchers in the fields of climate change, river basin hydrology, agricultural application, energy budget, and the water and carbon cycle. This Topic on “Progress in Satellite Remote Sensing of Land Surface: New Algorithms, Sensors and Datasets” will cover recent advances in remote sensing sensors, algorithms, and datasets for quantifying land surface parameters. Original research reports, review articles, and commentaries are welcome. The issue will host papers covering remote sensing algorithms for retrieving land surface parameters including, but not limited to, soil, snow and ice, forest, grass land, farmland, and water and urban areas. Papers focusing on new orbital sensors and land surface datasets are also welcome. Data from new satellites, such as the recently launched FengYun satellite series, are warmly encouraged to be used in this Topic.
Dr. Shengli Wu
Dr. Lingmei Jiang
Dr. Jinyang Du
Prof. Dr. Kebiao Mao
Dr. Tianjie Zhao
Topic Editors
Keywords
- soil moisture
- vegetation index
- snow depth
- snow water equivalents
- water body
- glacier
- land surface temperature
Participating Journals
Journal Name | Impact Factor | CiteScore | Launched Year | First Decision (median) | APC |
---|---|---|---|---|---|
![]()
Atmosphere
|
2.5 | 4.6 | 2010 | 16.1 Days | CHF 2400 |
![]()
Climate
|
3.0 | 5.5 | 2013 | 19.7 Days | CHF 1800 |
![]()
Land
|
3.2 | 4.9 | 2012 | 16.9 Days | CHF 2600 |
![]()
Remote Sensing
|
4.2 | 8.3 | 2009 | 23.9 Days | CHF 2700 |
![]()
Sensors
|
3.4 | 7.3 | 2001 | 18.6 Days | CHF 2600 |
Preprints.org is a multidisciplinary platform offering a preprint service designed to facilitate the early sharing of your research. It supports and empowers your research journey from the very beginning.
MDPI Topics is collaborating with Preprints.org and has established a direct connection between MDPI journals and the platform. Authors are encouraged to take advantage of this opportunity by posting their preprints at Preprints.org prior to publication:
- Share your research immediately: disseminate your ideas prior to publication and establish priority for your work.
- Safeguard your intellectual contribution: Protect your ideas with a time-stamped preprint that serves as proof of your research timeline.
- Boost visibility and impact: Increase the reach and influence of your research by making it accessible to a global audience.
- Gain early feedback: Receive valuable input and insights from peers before submitting to a journal.
- Ensure broad indexing: Web of Science (Preprint Citation Index), Google Scholar, Crossref, SHARE, PrePubMed, Scilit and Europe PMC.