Topic Editors
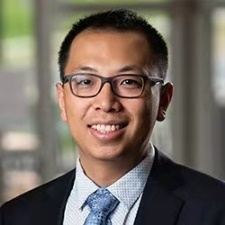

Innovative Horizons: Digital Technologies in Modern Construction and Infrastructure
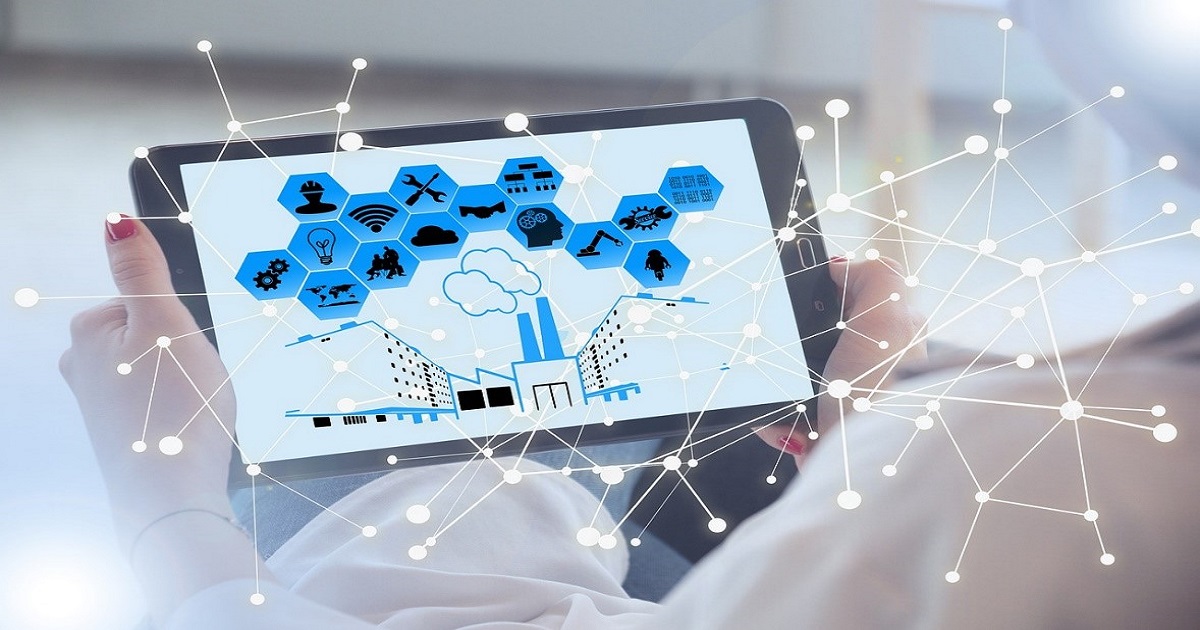
Topic Information
Dear Colleagues,
The construction industry is experiencing a significant shift towards digital adoption, particularly in the realm of modern construction methods like offsite manufacturing. This digital revolution is restructuring how we approach building and infrastructure projects, offering new ways to enhance efficiency, productivity, sustainability, and overall project outcomes. Our proposed Topic Collection, about "Innovative Horizons: Digital Technologies in Modern Construction and Infrastructure", seeks to shed light on the latest advancements driving this transformation. Through the lens of the People, Process, and Technology (PPT) framework, we are particularly interested in exploring how digital tools are supporting and advancing modern construction and building techniques, as well as their application across the broader construction and infrastructure sectors. We welcome high-quality research papers that address, but are not limited to, the following focus areas:
- Reality capture technologies in construction planning and control;
- Remote sensing technologies in infrastructure management;
- Drone technology for project monitoring;
- Mobile technologies for on-site quality control and safety management;
- Data analytics for performance improvement in modern construction Blockchain applications in sustainable supply chain management for construction;
- AI and machine learning for project planning, optimization, and control;
- IoT applications in smart buildings and infrastructure management;
- AR/VR use in design and offsite manufacturing;
- Automation and robotics in prefabrication processes;
- Digital twins for lifecycle management;
- BIM integration in offsite and onsite construction;
- Cloud-based collaboration in distributed construction teams.
We invite original research, reviews, and case studies that offer fresh insights into these technologies and their impact. Our goal is to create a valuable resource for researchers and practitioners, fostering innovation in this rapidly evolving field. We look forward to your contributions to this exciting collection
Dr. Zhen Lei
Dr. Ala Suliman
Dr. Hexu Liu
Topic Editors
Keywords
- digital construction
- building information modeling (BIM)
- Internet of Things (IoT)
- artificial intelligence
- digital twin
- smart infrastructure
- Construction 4.0
- automation
- virtual reality
- smart cities
Participating Journals
Journal Name | Impact Factor | CiteScore | Launched Year | First Decision (median) | APC | |
---|---|---|---|---|---|---|
![]()
Architecture
|
- | - | 2021 | 36.3 Days | CHF 1000 | Submit |
![]()
Buildings
|
3.1 | 3.4 | 2011 | 15.3 Days | CHF 2600 | Submit |
![]()
Infrastructures
|
2.7 | 5.2 | 2016 | 17.8 Days | CHF 1800 | Submit |
![]()
Sensors
|
3.4 | 7.3 | 2001 | 18.6 Days | CHF 2600 | Submit |
![]()
Sustainability
|
3.3 | 6.8 | 2009 | 19.7 Days | CHF 2400 | Submit |
![]()
ISPRS International Journal of Geo-Information
|
2.8 | 6.9 | 2012 | 35.8 Days | CHF 1900 | Submit |
Preprints.org is a multidisciplinary platform offering a preprint service designed to facilitate the early sharing of your research. It supports and empowers your research journey from the very beginning.
MDPI Topics is collaborating with Preprints.org and has established a direct connection between MDPI journals and the platform. Authors are encouraged to take advantage of this opportunity by posting their preprints at Preprints.org prior to publication:
- Share your research immediately: disseminate your ideas prior to publication and establish priority for your work.
- Safeguard your intellectual contribution: Protect your ideas with a time-stamped preprint that serves as proof of your research timeline.
- Boost visibility and impact: Increase the reach and influence of your research by making it accessible to a global audience.
- Gain early feedback: Receive valuable input and insights from peers before submitting to a journal.
- Ensure broad indexing: Web of Science (Preprint Citation Index), Google Scholar, Crossref, SHARE, PrePubMed, Scilit and Europe PMC.