Topic Menu
► Topic MenuTopic Editors
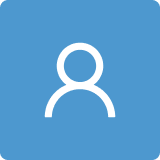
Cloud and Edge Computing for Smart Devices
Topic Information
Dear Colleagues,
In recent years, mobile devices have become indispensable devices that can share a variety of data despite their distance or specifications. Additionally, cloud and edge computing are emerging as promising technologies for the Internet of Things and cyber-physical systems. Moving computing to the edge has many benefits: faster response times, data privacy and security, and resilience if the cloud becomes unavailable.
The Topic of “Cloud and Edge Computing for Smart Devices” invites papers on theoretical and applied issues including, but not limited to, the following:
- cloud, mobile cloud and fog computing;
- blockchain and its application;
- vehicular cloud computing;
- smart vehicles and connected vehicles smart city;
- cloud computing;
- algorithms;
- artificial intelligence;
- parallel computing;
- Internet of Things.
Dr. Mehdi Sookhak
Dr. Francesco Moscato
Topic Editors
Keywords
- cloud, mobile cloud, and fog computing
- blockchain and its application
- vehicular cloud computing
- smart vehicles and connected vehicles smart city
- Internet of Things
Participating Journals
Journal Name | Impact Factor | CiteScore | Launched Year | First Decision (median) | APC | |
---|---|---|---|---|---|---|
![]()
Applied Sciences
|
2.5 | 5.3 | 2011 | 18.4 Days | CHF 2400 | Submit |
![]()
Electronics
|
2.6 | 5.3 | 2012 | 16.4 Days | CHF 2400 | Submit |
![]()
Future Internet
|
2.8 | 7.1 | 2009 | 16.9 Days | CHF 1600 | Submit |
![]()
Sensors
|
3.4 | 7.3 | 2001 | 18.6 Days | CHF 2600 | Submit |
![]()
Smart Cities
|
7.0 | 11.2 | 2018 | 28.4 Days | CHF 2000 | Submit |
Preprints.org is a multidisciplinary platform offering a preprint service designed to facilitate the early sharing of your research. It supports and empowers your research journey from the very beginning.
MDPI Topics is collaborating with Preprints.org and has established a direct connection between MDPI journals and the platform. Authors are encouraged to take advantage of this opportunity by posting their preprints at Preprints.org prior to publication:
- Share your research immediately: disseminate your ideas prior to publication and establish priority for your work.
- Safeguard your intellectual contribution: Protect your ideas with a time-stamped preprint that serves as proof of your research timeline.
- Boost visibility and impact: Increase the reach and influence of your research by making it accessible to a global audience.
- Gain early feedback: Receive valuable input and insights from peers before submitting to a journal.
- Ensure broad indexing: Web of Science (Preprint Citation Index), Google Scholar, Crossref, SHARE, PrePubMed, Scilit and Europe PMC.