Topic Menu
► Topic MenuTopic Editors





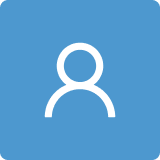
Artificial Intelligence in Sensors, 2nd Volume
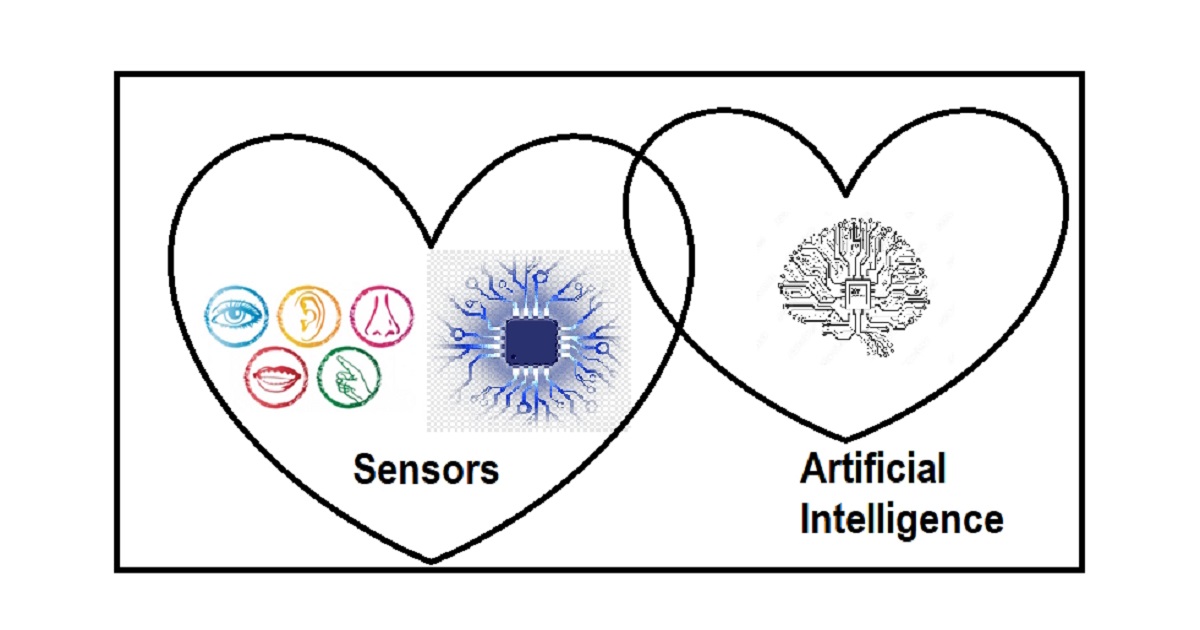
Topic Information
Dear Colleagues,
Following the success of the previous topic “Artificial Intelligence in Sensors”, we are pleased to announce the next in the series, entitled “Artificial Intelligence in Sensors, 2nd Volume”.
This topic comprises several interdisciplinary research areas that cover the main aspects of sensor sciences. There has been an increase in both the capabilities and challenges related within numerous application fields, e.g., robotics, industry 4.0, automotive, smart cties, medicine, diagnosis, food, telecommunication, environmental and civil applications, health and security.
The associated applications constantly require novel sensors to improve their capabilities and challenges. Thus, sensor sciences represents a paradigm characterized by the integration of modern nanotechnologies and nanomaterials into manufacturing and industrial practice to develop tools for several application fields. The primary underlying goal of sensor sciences is to facilitate the closer interconnection and control of complex systems, machines, devices, and people to increase the support provided to humans in several application fields.
Sensor sciences comprises a set of significant research fields, including:
- Advanced data visualization techniques;
- Advanced interactive technologies, including augmented/virtual reality;
- Artificial intelligence;
- Big data processing and analytics;
- Biosensors;
- Chemical sensors;
- Cognitive computing platforms and applications;
- Computer vision;
- Data security;
- Deep learning;
- Electronics and mechanicals;
- Image processing;
- Instrumentation science and technology;
- Intelligent sensor;
- Interdisciplinary sciences.
- Internet of Things platforms and their applications;
- Machine learning;
- Machine vision;
- Materials and nanomaterials;
- Measurement science and technology;
- Mechatronics;
- MEMS, microwaves and acoustic waves;
- Microfluidics;
- Nanotechnology;
- Optical sensors;
- Optoelectronics, photonics, and optical fibers;
- Organic electronics, biophotonics and smart materials;
- Physical sensors;
- Physics and biophysics;
- Remote sensing;
- Robotics;
- Sensor networks;
- Smart sensors and sensing;
- UAV;
- UGV.
This topic aims to collect the results of research in these fields and others. Therefore, submitting papers within those areas connected to sensors is strongly encouraged.
Prof. Dr. Yangquan Chen
Prof. Dr. Nunzio Cennamo
Prof. Dr. M. Jamal Deen
Dr. Junseop Lee
Prof. Dr. Subhas Mukhopadhyay
Prof. Dr. Simone Morais
Topic Editors
Keywords
- sensors
- sensing
- artificial intelligence
- deep learning
- machine learning
- computer vision
- big data
- IoT
Participating Journals
Journal Name | Impact Factor | CiteScore | Launched Year | First Decision (median) | APC |
---|---|---|---|---|---|
![]()
Applied Sciences
|
2.5 | 5.3 | 2011 | 18.4 Days | CHF 2400 |
![]()
Drones
|
4.4 | 5.6 | 2017 | 19.2 Days | CHF 2600 |
![]()
Electronics
|
2.6 | 5.3 | 2012 | 16.4 Days | CHF 2400 |
![]()
Remote Sensing
|
4.2 | 8.3 | 2009 | 23.9 Days | CHF 2700 |
![]()
Sensors
|
3.4 | 7.3 | 2001 | 18.6 Days | CHF 2600 |
![]()
Technologies
|
4.2 | 6.7 | 2013 | 21.1 Days | CHF 1600 |
Preprints.org is a multidisciplinary platform offering a preprint service designed to facilitate the early sharing of your research. It supports and empowers your research journey from the very beginning.
MDPI Topics is collaborating with Preprints.org and has established a direct connection between MDPI journals and the platform. Authors are encouraged to take advantage of this opportunity by posting their preprints at Preprints.org prior to publication:
- Share your research immediately: disseminate your ideas prior to publication and establish priority for your work.
- Safeguard your intellectual contribution: Protect your ideas with a time-stamped preprint that serves as proof of your research timeline.
- Boost visibility and impact: Increase the reach and influence of your research by making it accessible to a global audience.
- Gain early feedback: Receive valuable input and insights from peers before submitting to a journal.
- Ensure broad indexing: Web of Science (Preprint Citation Index), Google Scholar, Crossref, SHARE, PrePubMed, Scilit and Europe PMC.