Advance of Applied Statistics to White-Boxing AI in Engineering
A special issue of Symmetry (ISSN 2073-8994). This special issue belongs to the section "Computer".
Deadline for manuscript submissions: closed (31 October 2021) | Viewed by 2559
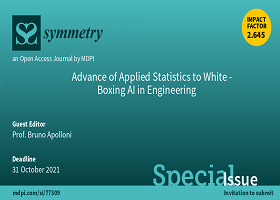
Special Issue Editor
Special Issue Information
Dear Colleagues,
Artificial intelligence implementation is vastly beneficial. Engineers may manage pre-featured AI applications either as black boxes, to be properly fed with input, or as parts of their own design tools that can be explicitly integrated into overall projects. The second option requires white boxing the apps in terms of their statistic rationale, from the current perspective of machine learning methods, so as to restore a good level of knowledge symmetry between the designer and the implementer of the apps. This Special Issue aims to collect papers that reflect the advanced practices and research of statistics to convert AI engineering applications from third-party facilities to regular tools of the design arsenal with which engineers can be called to renew the industrial world.
Prof. Bruno Apolloni
Guest Editor
Manuscript Submission Information
Manuscripts should be submitted online at www.mdpi.com by registering and logging in to this website. Once you are registered, click here to go to the submission form. Manuscripts can be submitted until the deadline. All submissions that pass pre-check are peer-reviewed. Accepted papers will be published continuously in the journal (as soon as accepted) and will be listed together on the special issue website. Research articles, review articles as well as short communications are invited. For planned papers, a title and short abstract (about 100 words) can be sent to the Editorial Office for announcement on this website.
Submitted manuscripts should not have been published previously, nor be under consideration for publication elsewhere (except conference proceedings papers). All manuscripts are thoroughly refereed through a single-blind peer-review process. A guide for authors and other relevant information for submission of manuscripts is available on the Instructions for Authors page. Symmetry is an international peer-reviewed open access monthly journal published by MDPI.
Please visit the Instructions for Authors page before submitting a manuscript. The Article Processing Charge (APC) for publication in this open access journal is 2400 CHF (Swiss Francs). Submitted papers should be well formatted and use good English. Authors may use MDPI's English editing service prior to publication or during author revisions.
Keywords
- artificial intelligence
- white boxing
- statistic rationale
- machine learning
- AI engineering applications
Benefits of Publishing in a Special Issue
- Ease of navigation: Grouping papers by topic helps scholars navigate broad scope journals more efficiently.
- Greater discoverability: Special Issues support the reach and impact of scientific research. Articles in Special Issues are more discoverable and cited more frequently.
- Expansion of research network: Special Issues facilitate connections among authors, fostering scientific collaborations.
- External promotion: Articles in Special Issues are often promoted through the journal's social media, increasing their visibility.
- Reprint: MDPI Books provides the opportunity to republish successful Special Issues in book format, both online and in print.
Further information on MDPI's Special Issue policies can be found here.