Computing for Sustainable Energy
A special issue of Energies (ISSN 1996-1073). This special issue belongs to the section "A: Sustainable Energy".
Deadline for manuscript submissions: closed (31 March 2022) | Viewed by 6216
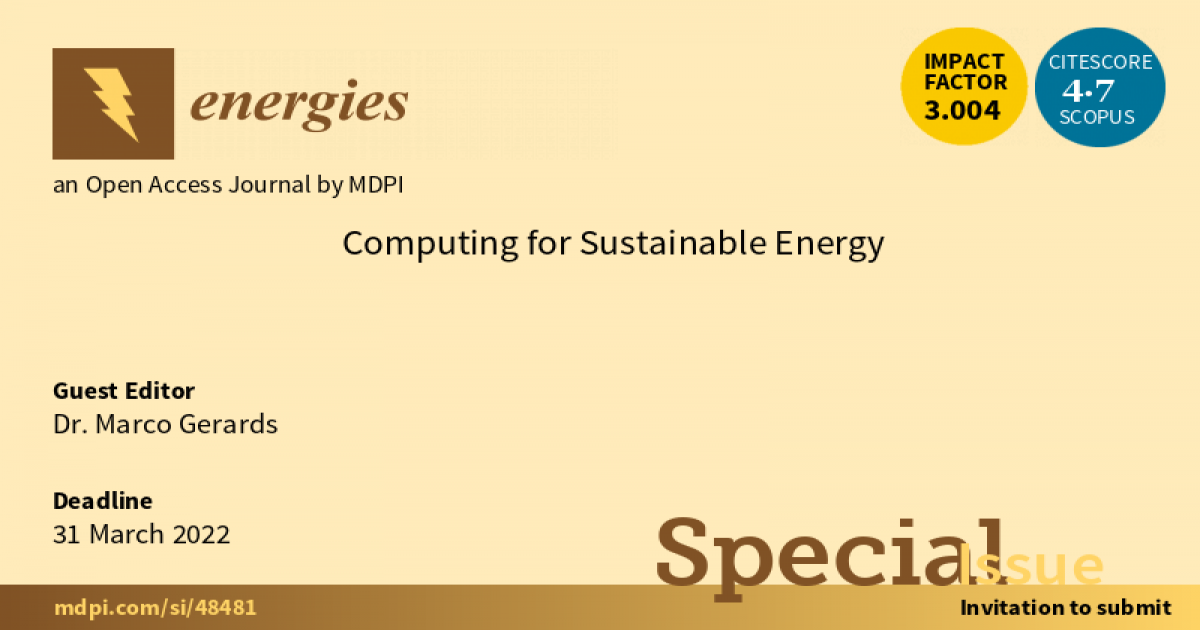
Special Issue Editor
Special Issue Information
Dear Colleagues,
Due to global warming, it is essential to prepare our energy system for a high level of renewable energy penetration and an increased electrification of transportation and heating. This requires a shift from top–down demand-driven energy generation towards a bottom–up distributed system where demand adapts to local (renewable) generation. This shift requires a smart grid that is supported by a high volume of measurements, efficient algorithms, and a modern communication infrastructure.
With the development of the smart grid, many computational challenges occur. Decisions for operating the network, controlling batteries, and smart appliances will be automated by ICT systems that optimally control the equipment and the smart grid as a whole. For this new way of steering the energy system, new efficient optimization, machine learning, and control algorithms are needed. To be successful, the algorithm designer needs to take into account efficiency, computational complexity, required communication volumes, data quality, privacy and security, etc. For this Special Issue, we invite submissions that address these and related issues.
Dr. Marco GerardsGuest Editor
Manuscript Submission Information
Manuscripts should be submitted online at www.mdpi.com by registering and logging in to this website. Once you are registered, click here to go to the submission form. Manuscripts can be submitted until the deadline. All submissions that pass pre-check are peer-reviewed. Accepted papers will be published continuously in the journal (as soon as accepted) and will be listed together on the special issue website. Research articles, review articles as well as short communications are invited. For planned papers, a title and short abstract (about 100 words) can be sent to the Editorial Office for announcement on this website.
Submitted manuscripts should not have been published previously, nor be under consideration for publication elsewhere (except conference proceedings papers). All manuscripts are thoroughly refereed through a single-blind peer-review process. A guide for authors and other relevant information for submission of manuscripts is available on the Instructions for Authors page. Energies is an international peer-reviewed open access semimonthly journal published by MDPI.
Please visit the Instructions for Authors page before submitting a manuscript. The Article Processing Charge (APC) for publication in this open access journal is 2600 CHF (Swiss Francs). Submitted papers should be well formatted and use good English. Authors may use MDPI's English editing service prior to publication or during author revisions.
Keywords
- Algorithmic game theory for smart grids
- Data science for smart grids
- Decentralized energy management
- Demand side management
- Demand response
- Machine learning for energy applications
- Optimization algorithms for smart grids