Explainable Artificial Intelligence (XAI) in Biomedical Research and Clinical Practice
A special issue of BioMedInformatics (ISSN 2673-7426).
Deadline for manuscript submissions: closed (31 December 2021) | Viewed by 39352
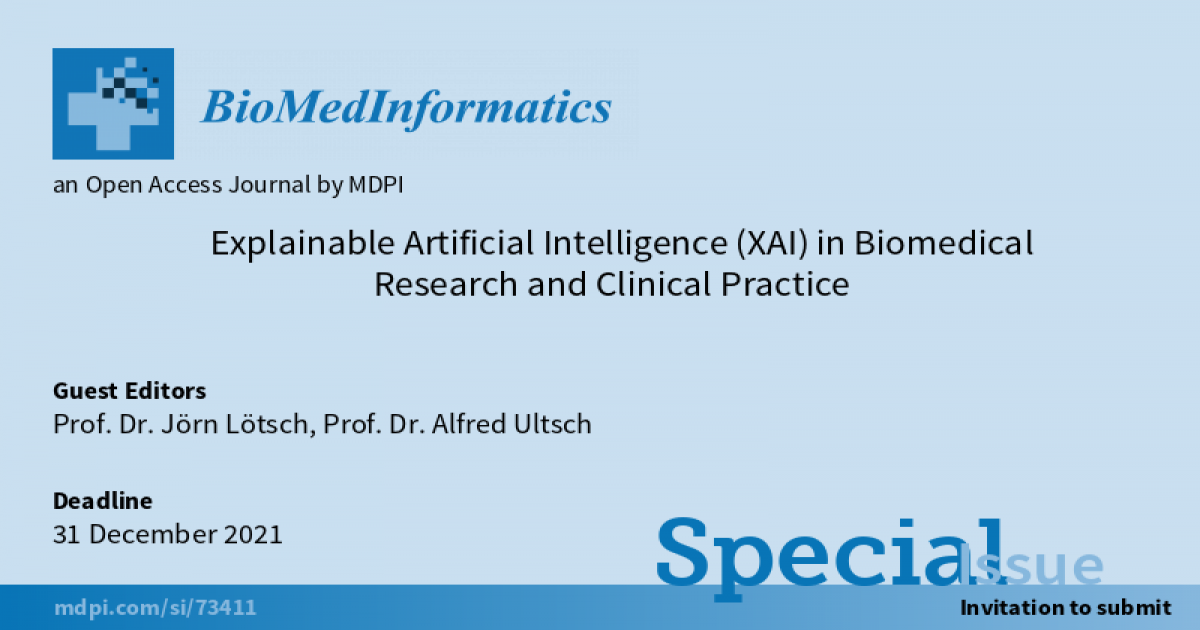
Special Issue Editors
2. Fraunhofer Institute for Translational Medicine and Pharmacology ITMP, Theodor-Stern-Kai 7, 60596 Frankfurt am Main, Germany
Interests: pharmacological data science; applied artificial intelligence; statistical parametric mapping; nonlinear-mixed effects modeling
Special Issue Information
Dear Colleagues,
Advanced computational methods of machine learning and related artificial intelligence are increasingly entering biomedical research and clinical practice. These processes are bidirectional. Computational methods are used to solve biomedical problems and biological systems are studied to develop and improve artificial intelligence methods, enabling a paradigm shift from hypothesis-driven research and clinical decision-making to data-driven approaches to discovering knowledge from biomedical data.
The shift from therapy-relevant decisions based on biomedical knowledge to black-box-like computer algorithms makes the decision-making increasingly incomprehensible to medical staff and patients. This has been recognized in the issuance of guidelines, e.g., by the European Union or DARPA (USA), which emphasize the need for computer-based decisions to be transparent and in a form that can be communicated in an understandable way to medical personnel and patients. To address this problem, the concept of explainable artificial intelligence (XAI) is attracting scientific interest. XAI uses a representation of human knowledge, usually (a subset of) predicate logic, for its reasoning, deduction, and classification (diagnosis).
In this Special Issue of Biomedinformatics, we invite contributions on the development and implementation of explainable artificial intelligence (XAI) algorithms in biomedical research and practice, focusing on, but not limited to, original research reports.
Prof. Dr. Jörn Lötsch
Prof. Alfred Ultsch
Guest Editors
Manuscript Submission Information
Manuscripts should be submitted online at www.mdpi.com by registering and logging in to this website. Once you are registered, click here to go to the submission form. Manuscripts can be submitted until the deadline. All submissions that pass pre-check are peer-reviewed. Accepted papers will be published continuously in the journal (as soon as accepted) and will be listed together on the special issue website. Research articles, review articles as well as short communications are invited. For planned papers, a title and short abstract (about 100 words) can be sent to the Editorial Office for announcement on this website.
Submitted manuscripts should not have been published previously, nor be under consideration for publication elsewhere (except conference proceedings papers). All manuscripts are thoroughly refereed through a single-blind peer-review process. A guide for authors and other relevant information for submission of manuscripts is available on the Instructions for Authors page. BioMedInformatics is an international peer-reviewed open access quarterly journal published by MDPI.
Please visit the Instructions for Authors page before submitting a manuscript. The Article Processing Charge (APC) for publication in this open access journal is 1000 CHF (Swiss Francs). Submitted papers should be well formatted and use good English. Authors may use MDPI's English editing service prior to publication or during author revisions.
Keywords
- Computer-aided classification and subgroup detection
- Personalized and precision medicine
- Supervised and unsupervised machine learning
- Symbolic machine learning
- Understandable data mining
- Explainable artificial intelligence
- Biomedical knowledge representation
- Biomedical knowledge discovery
- Controlled hypothesis generation
Benefits of Publishing in a Special Issue
- Ease of navigation: Grouping papers by topic helps scholars navigate broad scope journals more efficiently.
- Greater discoverability: Special Issues support the reach and impact of scientific research. Articles in Special Issues are more discoverable and cited more frequently.
- Expansion of research network: Special Issues facilitate connections among authors, fostering scientific collaborations.
- External promotion: Articles in Special Issues are often promoted through the journal's social media, increasing their visibility.
- Reprint: MDPI Books provides the opportunity to republish successful Special Issues in book format, both online and in print.
Further information on MDPI's Special Issue policies can be found here.