Deep Learning for Object Detection
A special issue of Applied Sciences (ISSN 2076-3417). This special issue belongs to the section "Computing and Artificial Intelligence".
Deadline for manuscript submissions: 20 May 2025 | Viewed by 39104
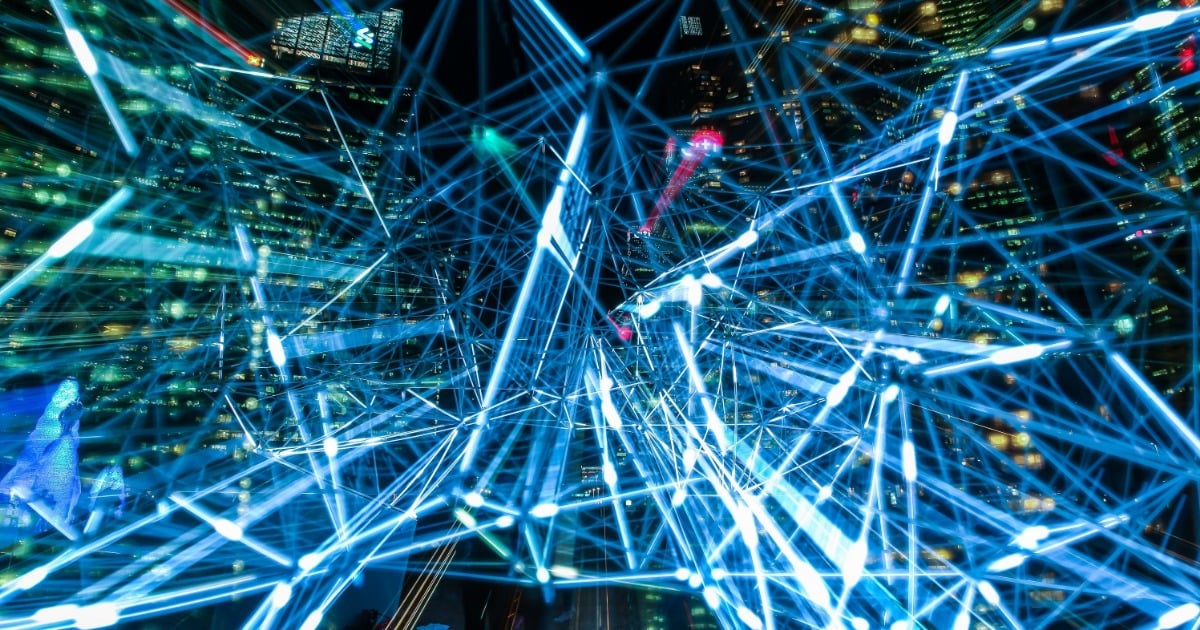
Special Issue Editor
Interests: you only look once (YOLO); big data; convolutional neural networks (CNNs); object detection; artificial intelligence
Special Issues, Collections and Topics in MDPI journals
Special Issue Information
Dear Colleagues,
Currently, models based on convolutional neural networks (CNNs) are increasingly being applied for image classification due to their ability to handle big data. Models such as you only look once (YOLO) have become very popular for having greater flexibility and good performance in object identification.
In this Special Issue, we are aiming to collate studies on all of the aspects surrounding “Deep Learning for Object Detection”. Any original, unpublished work is welcome. If you have an interest in this topic, please let us know.
Dr. Stéfano Frizzo Stefenon
Guest Editor
Manuscript Submission Information
Manuscripts should be submitted online at www.mdpi.com by registering and logging in to this website. Once you are registered, click here to go to the submission form. Manuscripts can be submitted until the deadline. All submissions that pass pre-check are peer-reviewed. Accepted papers will be published continuously in the journal (as soon as accepted) and will be listed together on the special issue website. Research articles, review articles as well as short communications are invited. For planned papers, a title and short abstract (about 100 words) can be sent to the Editorial Office for announcement on this website.
Submitted manuscripts should not have been published previously, nor be under consideration for publication elsewhere (except conference proceedings papers). All manuscripts are thoroughly refereed through a single-blind peer-review process. A guide for authors and other relevant information for submission of manuscripts is available on the Instructions for Authors page. Applied Sciences is an international peer-reviewed open access semimonthly journal published by MDPI.
Please visit the Instructions for Authors page before submitting a manuscript. The Article Processing Charge (APC) for publication in this open access journal is 2400 CHF (Swiss Francs). Submitted papers should be well formatted and use good English. Authors may use MDPI's English editing service prior to publication or during author revisions.
Keywords
- you only look once (YOLO)
- big data
- convolutional neural networks (CNNs)
- object detection
- artificial intelligence
Benefits of Publishing in a Special Issue
- Ease of navigation: Grouping papers by topic helps scholars navigate broad scope journals more efficiently.
- Greater discoverability: Special Issues support the reach and impact of scientific research. Articles in Special Issues are more discoverable and cited more frequently.
- Expansion of research network: Special Issues facilitate connections among authors, fostering scientific collaborations.
- External promotion: Articles in Special Issues are often promoted through the journal's social media, increasing their visibility.
- e-Book format: Special Issues with more than 10 articles can be published as dedicated e-books, ensuring wide and rapid dissemination.
Further information on MDPI's Special Issue policies can be found here.