Topic Menu
► Topic MenuTopic Editors
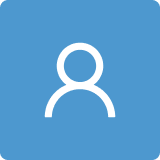

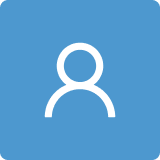
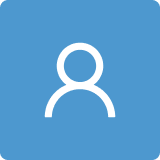
Advances in the Monitoring and Diagnosis of Faults in Wind Power Plants
Topic Information
Dear Colleagues,
The generation of electrical power in wind farms is currently a key technology in the energy transition to a system based on renewable sources, free from greenhouse gas emissions, and therefore essential in the fight against climate change.
Although wind power generation is currently a mature technology, one of the inherent problems it presents is the difficulty of accessing the generator units, which complicates maintenance and repair work in case of breakdowns. This problem worsens with the aging of the wind farms and also with the installation of new ones, where the power of the units (and consequently the height of the towers and the difficulty of access) continuously increases. For all these reasons, the importance of monitoring and early fault detection techniques for wind power plants is growing every day. These techniques allow the development of predictive maintenance systems that minimize operating costs and production losses due to unexpected shutdowns.
The objective of this topic is to provide a multidisciplinary overview of the most recent advances in the field of monitoring and early detection of faults in wind power units. Works related to fault detection in any of the elements or systems of wind turbine units (turbine, powertrain, bearings, gearbox, electrical generator, electronic controller, yaw system, pitch system, etc.) are of interest in this section. These works may be based on the use of any type of sensor or data provided by the SCADA system, using signal analysis techniques, model-based methods, data-driven based methods, or AI-based methods. In particular, contributions suited for this topic include, but are not limited to:
- New diagnostic techniques based on conventional sensors for wind turbine fault diagnosis
- Development and application of smart sensors for wind turbine fault diagnosis
- Use of electrical drive as a sensor for fault detection in wind turbines; embedded diagnostic systems in pre-existing controllers
- Fault diagnosis based on SCADA data processing
- Diagnostic techniques in wind power units, based on the integration of information provided by different types of sensors
- Application of AI to wind turbine fault diagnosis.
- Development of deep learning based methods to fault detection in wind turbines.
- Development of models for fault detection. Methodologies based on digital twins.
Prof. Dr. Javier Martinez-Roman
Prof. Dr. Manuel Pineda-Sanchez
Prof. Dr. Martin Riera-Guasp
Prof. Dr. Angel Sapena-Bano
Prof. Dr. Jordi Burriel-Valencia
Topic Editors
Keywords
- wind power plants
- fault diagnosis
- smart sensors
- digital twins
- SCADA
- AI diagnosis methods
- data driven diagnosis methods
Participating Journals
Journal Name | Impact Factor | CiteScore | Launched Year | First Decision (median) | APC |
---|---|---|---|---|---|
![]()
Actuators
|
2.2 | 4.3 | 2012 | 17.7 Days | CHF 2400 |
![]()
Applied Sciences
|
2.5 | 5.5 | 2011 | 18.4 Days | CHF 2400 |
![]()
Energies
|
3.0 | 7.3 | 2008 | 16.8 Days | CHF 2600 |
![]()
Machines
|
2.1 | 4.7 | 2013 | 15.5 Days | CHF 2400 |
![]()
Sensors
|
3.4 | 8.2 | 2001 | 18.6 Days | CHF 2600 |
Preprints.org is a multidisciplinary platform offering a preprint service designed to facilitate the early sharing of your research. It supports and empowers your research journey from the very beginning.
MDPI Topics is collaborating with Preprints.org and has established a direct connection between MDPI journals and the platform. Authors are encouraged to take advantage of this opportunity by posting their preprints at Preprints.org prior to publication:
- Share your research immediately: disseminate your ideas prior to publication and establish priority for your work.
- Safeguard your intellectual contribution: Protect your ideas with a time-stamped preprint that serves as proof of your research timeline.
- Boost visibility and impact: Increase the reach and influence of your research by making it accessible to a global audience.
- Gain early feedback: Receive valuable input and insights from peers before submitting to a journal.
- Ensure broad indexing: Web of Science (Preprint Citation Index), Google Scholar, Crossref, SHARE, PrePubMed, Scilit and Europe PMC.