Complex Systems Modeling and Analysis
A special issue of Entropy (ISSN 1099-4300). This special issue belongs to the section "Complexity".
Deadline for manuscript submissions: closed (29 April 2022) | Viewed by 17807
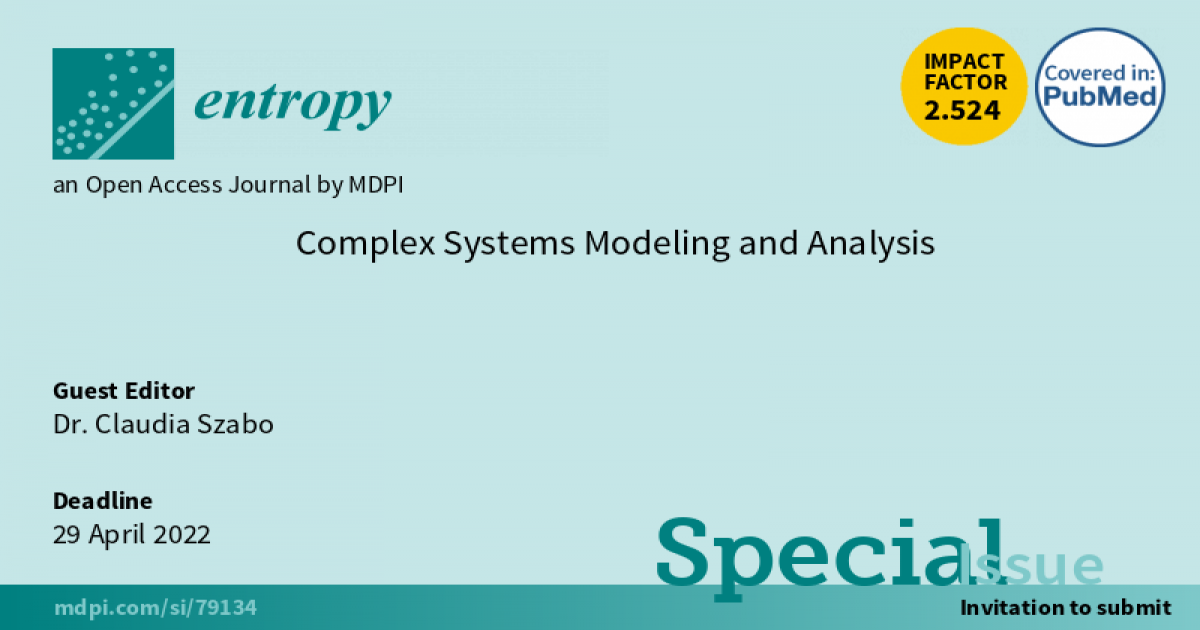
Special Issue Editor
Special Issue Information
Dear Colleagues,
Understanding the malign and benign properties of complex systems is critical as complexity becomes ubiquitous with the increase in system scale and the evolution of technology. Simulation, modeling and analysis techniques are fundamental in order to increase trust in the behavior of complex systems, in particular as they operate in dynamic environments or under unforeseen constraints. In addition, self-* such as self-organisation, self-healing, self-adaptation and self-optimization are becoming de-facto as a large number of systems employ several machine learning techniques in their quest to becoming autonomous. In this context, a good understanding of the complex system’s emergent behaviors, together with formal or informal guarantees about the system’s execution in particular settings will significantly advance the use of complex systems approaches in everyday life.
This Special Issue on “Complex Systems Modeling and Analysis” presents a platform where academic and industry researchers can present methodologies, techniques, applications and experiments that aim to increase our understanding of complex systems and their emergent behaviors. The focus of this Special Issue is both on modelling and simulation techniques but also on their practical application on various scenarios, and as such papers are welcome on a variety of topics including modelling, simulation, analysis, experimentation, and specific properties as defined above.
Dr. Claudia Szabo
Guest Editor
Manuscript Submission Information
Manuscripts should be submitted online at www.mdpi.com by registering and logging in to this website. Once you are registered, click here to go to the submission form. Manuscripts can be submitted until the deadline. All submissions that pass pre-check are peer-reviewed. Accepted papers will be published continuously in the journal (as soon as accepted) and will be listed together on the special issue website. Research articles, review articles as well as short communications are invited. For planned papers, a title and short abstract (about 100 words) can be sent to the Editorial Office for announcement on this website.
Submitted manuscripts should not have been published previously, nor be under consideration for publication elsewhere (except conference proceedings papers). All manuscripts are thoroughly refereed through a single-blind peer-review process. A guide for authors and other relevant information for submission of manuscripts is available on the Instructions for Authors page. Entropy is an international peer-reviewed open access monthly journal published by MDPI.
Please visit the Instructions for Authors page before submitting a manuscript. The Article Processing Charge (APC) for publication in this open access journal is 2600 CHF (Swiss Francs). Submitted papers should be well formatted and use good English. Authors may use MDPI's English editing service prior to publication or during author revisions.
Keywords
- complex systems
- emergent behavior
- modeling and analysis
- self-organisation
- adaptability
- self-*
- autonomous systems
Benefits of Publishing in a Special Issue
- Ease of navigation: Grouping papers by topic helps scholars navigate broad scope journals more efficiently.
- Greater discoverability: Special Issues support the reach and impact of scientific research. Articles in Special Issues are more discoverable and cited more frequently.
- Expansion of research network: Special Issues facilitate connections among authors, fostering scientific collaborations.
- External promotion: Articles in Special Issues are often promoted through the journal's social media, increasing their visibility.
- e-Book format: Special Issues with more than 10 articles can be published as dedicated e-books, ensuring wide and rapid dissemination.
Further information on MDPI's Special Issue policies can be found here.