Wearable Sensors for Precise Exercise Monitoring and Analysis
A special issue of Biosensors (ISSN 2079-6374). This special issue belongs to the section "Biosensor and Bioelectronic Devices".
Deadline for manuscript submissions: 31 August 2025 | Viewed by 3407
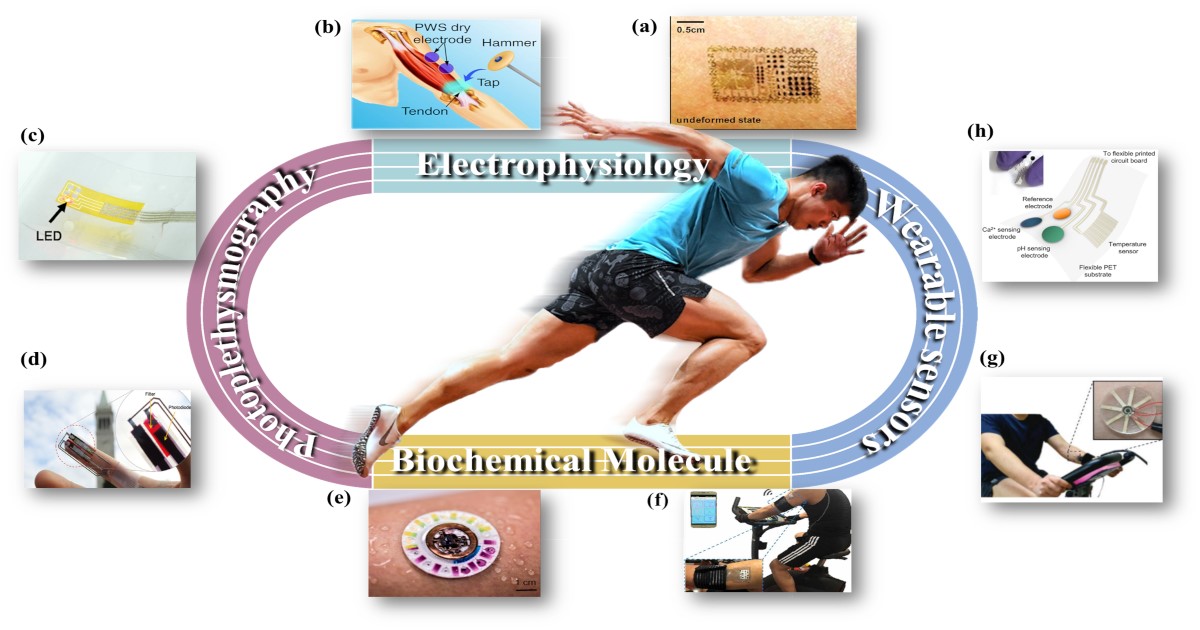
Special Issue Editors
Interests: photonic crystal materials; multi-analyte sensing; flexible electronics; printed assembly; 3D printing manufacture
Interests: nano printing; nanophotonics; quantum dots; bio-detection; optoelectronics
Special Issues, Collections and Topics in MDPI journals
Special Issue Information
Dear Colleagues,
Developed countries, such as the U.S., have used a variety of apparatuses to monitor the vital signs of athletes and to analyze their physical kineses. Then, scientific coaching schemes and body recovery procedures are programmed based on this database of quantitative vital signs. Focused on the critical challenges of exercise monitoring and analysis, we aim to extend out knowledge of the dynamic conditions and multi-analyte sensing and analysis involved in this practice. The latest research advancement in “exercise monitoring” or “sports analysis” via “wearable sensors” will be collected in this Special Issue to present a general view of the precise physical assessments conducted during exercise training for athletes. The cross-subject nature and basic theories of metrology, nanomaterials, and kinesiology will allow us to develop a new analytical methodology. We also aim to promote China’s “national sport prosperousness”.
Prof. Dr. Fengyu Li
Dr. Meng Su
Guest Editors
Manuscript Submission Information
Manuscripts should be submitted online at www.mdpi.com by registering and logging in to this website. Once you are registered, click here to go to the submission form. Manuscripts can be submitted until the deadline. All submissions that pass pre-check are peer-reviewed. Accepted papers will be published continuously in the journal (as soon as accepted) and will be listed together on the special issue website. Research articles, review articles as well as short communications are invited. For planned papers, a title and short abstract (about 100 words) can be sent to the Editorial Office for announcement on this website.
Submitted manuscripts should not have been published previously, nor be under consideration for publication elsewhere (except conference proceedings papers). All manuscripts are thoroughly refereed through a single-blind peer-review process. A guide for authors and other relevant information for submission of manuscripts is available on the Instructions for Authors page. Biosensors is an international peer-reviewed open access monthly journal published by MDPI.
Please visit the Instructions for Authors page before submitting a manuscript. The Article Processing Charge (APC) for publication in this open access journal is 2200 CHF (Swiss Francs). Submitted papers should be well formatted and use good English. Authors may use MDPI's English editing service prior to publication or during author revisions.
Keywords
- exercise monitoring
- sports analysis
- wearable sensors
- physical assessment
- fatigue detection
Benefits of Publishing in a Special Issue
- Ease of navigation: Grouping papers by topic helps scholars navigate broad scope journals more efficiently.
- Greater discoverability: Special Issues support the reach and impact of scientific research. Articles in Special Issues are more discoverable and cited more frequently.
- Expansion of research network: Special Issues facilitate connections among authors, fostering scientific collaborations.
- External promotion: Articles in Special Issues are often promoted through the journal's social media, increasing their visibility.
- Reprint: MDPI Books provides the opportunity to republish successful Special Issues in book format, both online and in print.
Further information on MDPI's Special Issue policies can be found here.