Neuromorphic Photonics: Current Devices, Systems and Perspectives
A special issue of Applied Sciences (ISSN 2076-3417). This special issue belongs to the section "Optics and Lasers".
Deadline for manuscript submissions: closed (20 September 2022) | Viewed by 6500
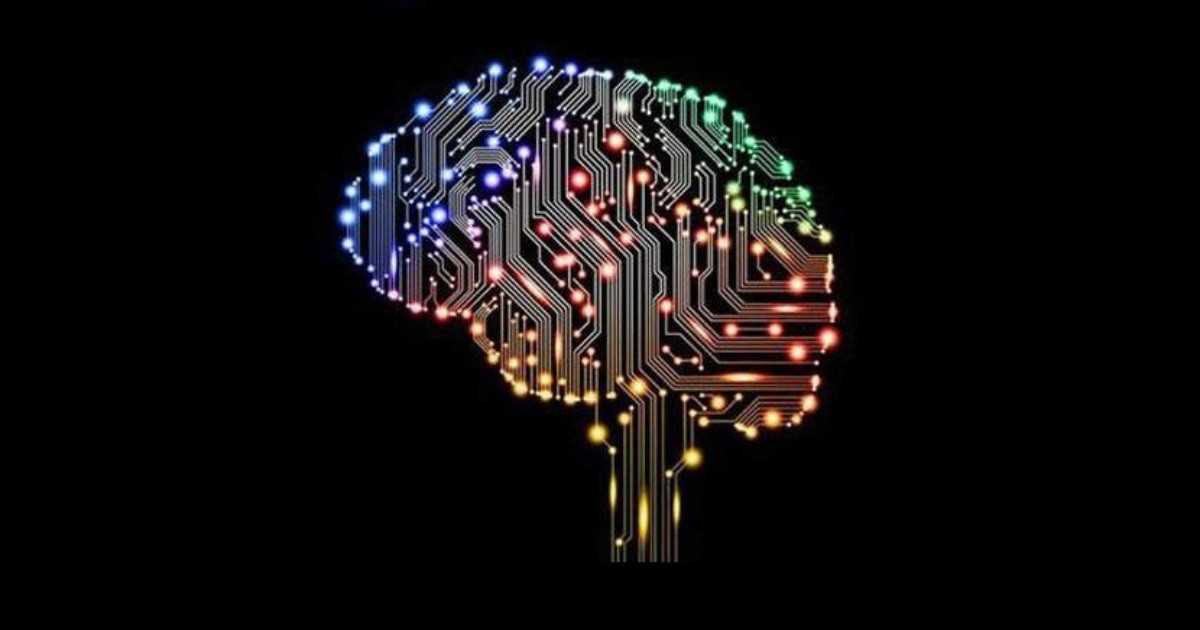
Special Issue Editors
Interests: neuromorphic photonics; optical communications; semiconductor optical amplifiers
Special Issue Information
Dear Colleagues,
The urgent need to extract or create value from digital knowledge bases with huge quantities of diverse data in an automated manner, often in real-time, together with the remarkable advances in photonic integration technologies, has given birth to one of the most exciting and multidisciplinary research fields of the present day—neuromorphic photonics. With deep learning (DL)-enabled AI being all-present in the widest spectrum of fields, challenges related to throughput, latency, and energy and footprint efficiency need to be addressed by future-proof solutions. At the same time, migrating from traditionally used digital electronics to photonics challenges the existing DL architectures and training algorithms by imposing new constraints, but also opening new possibilities through nonlinearities and architectures unique for photonic domain. In recent years, a wealth of devices, systems, and algorithms have emerged: from photonic spiking neurons to perceptrons, complemented by a rich portfolio of nonlinear activations including adaptable nonlinear functions, interconnected into layers and networks of various sizes and functionalities, embraced by photonic-hardware-aware training algorithms.
The focus of this Special Issue is multifold, from photonic devices that can enable a leap in photonic neural network (PNN) performance or provide new functionalities, through novel systems for addressing a variety of NNs and their layers, up to training algorithms that are adapted to the photonic hardware fabric and address the unique challenges that arise in neuromorphic photonics. Both original research papers and review articles are welcome.
Dr. Angelina Totovic
Dr. Nikolaos Passalis
Guest Editors
Manuscript Submission Information
Manuscripts should be submitted online at www.mdpi.com by registering and logging in to this website. Once you are registered, click here to go to the submission form. Manuscripts can be submitted until the deadline. All submissions that pass pre-check are peer-reviewed. Accepted papers will be published continuously in the journal (as soon as accepted) and will be listed together on the special issue website. Research articles, review articles as well as short communications are invited. For planned papers, a title and short abstract (about 100 words) can be sent to the Editorial Office for announcement on this website.
Submitted manuscripts should not have been published previously, nor be under consideration for publication elsewhere (except conference proceedings papers). All manuscripts are thoroughly refereed through a single-blind peer-review process. A guide for authors and other relevant information for submission of manuscripts is available on the Instructions for Authors page. Applied Sciences is an international peer-reviewed open access semimonthly journal published by MDPI.
Please visit the Instructions for Authors page before submitting a manuscript. The Article Processing Charge (APC) for publication in this open access journal is 2400 CHF (Swiss Francs). Submitted papers should be well formatted and use good English. Authors may use MDPI's English editing service prior to publication or during author revisions.
Keywords
- neuromoprhic photonics
- photonic neural networks
- photonic neurons
- photonic activation functions
- photonic AI accelerators
- photonic TPUs
- PICs for vector-by-matrix multiplication
- photonic deep learning
- reservoir computing