Methods, Applications and Developments in Biomedical Informatics: 2nd Edition
A special issue of Applied Sciences (ISSN 2076-3417). This special issue belongs to the section "Biomedical Engineering".
Deadline for manuscript submissions: 31 May 2025 | Viewed by 2378
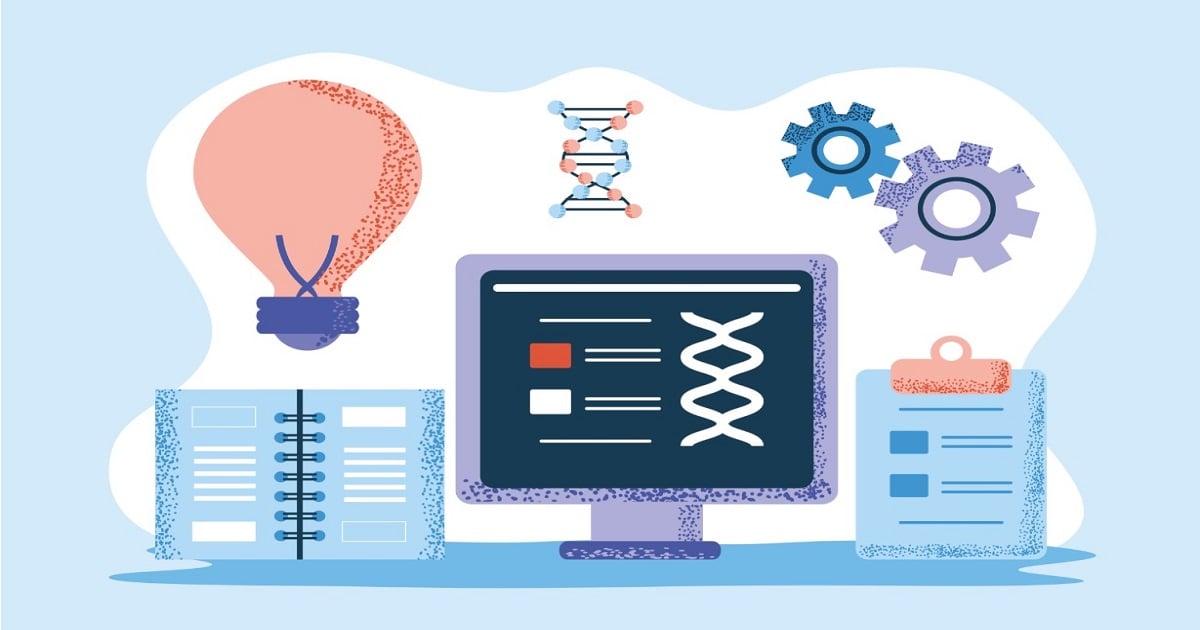
Special Issue Editors
Interests: artificial intelligence; biomedical applications of AI; business process management; case based reasoning; temporal abstractions; computational ontologies
Special Issues, Collections and Topics in MDPI journals
Interests: data standardization; medical technology; data exchange; e-health; biomedical informatics; privacy and security
Special Issues, Collections and Topics in MDPI journals
Special Issue Information
Dear Colleagues,
Computer science and artificial intelligence play a central role in the healthcare domain in various aspects, e.g., clinical research, decision support, process organization, management and optimization, telemedicine, and public health. In this Special Issue, we encourage the submission of original research articles, review articles, and short technical communications that focus on the above topics and areas. More specifically, we seek methodological articles on applications and recent developments in the context of artificial intelligence and biomedical informatics applied to the healthcare domain.
Topics include, but are not limited to, the following:
- Artificial intelligence;
- Data mining/machine learning;
- Clinical guidelines;
- Decision support and therapy improvement;
- Business process management/process mining;
- Health data acquisition and analysis;
- Healthcare information systems/knowledge representation/reasoning;
- Medical imaging and pattern recognition;
- Architectures and technologies for telehealth;
- Medical signal and data processing.
Dr. Giorgio Leonardi
Dr. Enno van der Velde
Guest Editors
Manuscript Submission Information
Manuscripts should be submitted online at www.mdpi.com by registering and logging in to this website. Once you are registered, click here to go to the submission form. Manuscripts can be submitted until the deadline. All submissions that pass pre-check are peer-reviewed. Accepted papers will be published continuously in the journal (as soon as accepted) and will be listed together on the special issue website. Research articles, review articles as well as short communications are invited. For planned papers, a title and short abstract (about 100 words) can be sent to the Editorial Office for announcement on this website.
Submitted manuscripts should not have been published previously, nor be under consideration for publication elsewhere (except conference proceedings papers). All manuscripts are thoroughly refereed through a single-blind peer-review process. A guide for authors and other relevant information for submission of manuscripts is available on the Instructions for Authors page. Applied Sciences is an international peer-reviewed open access semimonthly journal published by MDPI.
Please visit the Instructions for Authors page before submitting a manuscript. The Article Processing Charge (APC) for publication in this open access journal is 2400 CHF (Swiss Francs). Submitted papers should be well formatted and use good English. Authors may use MDPI's English editing service prior to publication or during author revisions.
Keywords
- artificial intelligence
- data mining/machine learning
- clinical guidelines
- decision support and therapy improvement
- business process management/process mining
- health data acquisition and analysis
- healthcare information systems/knowledge representation/reasoning
- medical imaging and pattern recognition
- architectures and technologies for telehealth
- medical signal and data processing
Benefits of Publishing in a Special Issue
- Ease of navigation: Grouping papers by topic helps scholars navigate broad scope journals more efficiently.
- Greater discoverability: Special Issues support the reach and impact of scientific research. Articles in Special Issues are more discoverable and cited more frequently.
- Expansion of research network: Special Issues facilitate connections among authors, fostering scientific collaborations.
- External promotion: Articles in Special Issues are often promoted through the journal's social media, increasing their visibility.
- e-Book format: Special Issues with more than 10 articles can be published as dedicated e-books, ensuring wide and rapid dissemination.
Further information on MDPI's Special Issue policies can be found here.