Advances and Applications of Deep Learning Methods and Image Processing
A special issue of Big Data and Cognitive Computing (ISSN 2504-2289).
Deadline for manuscript submissions: 16 July 2025 | Viewed by 33069
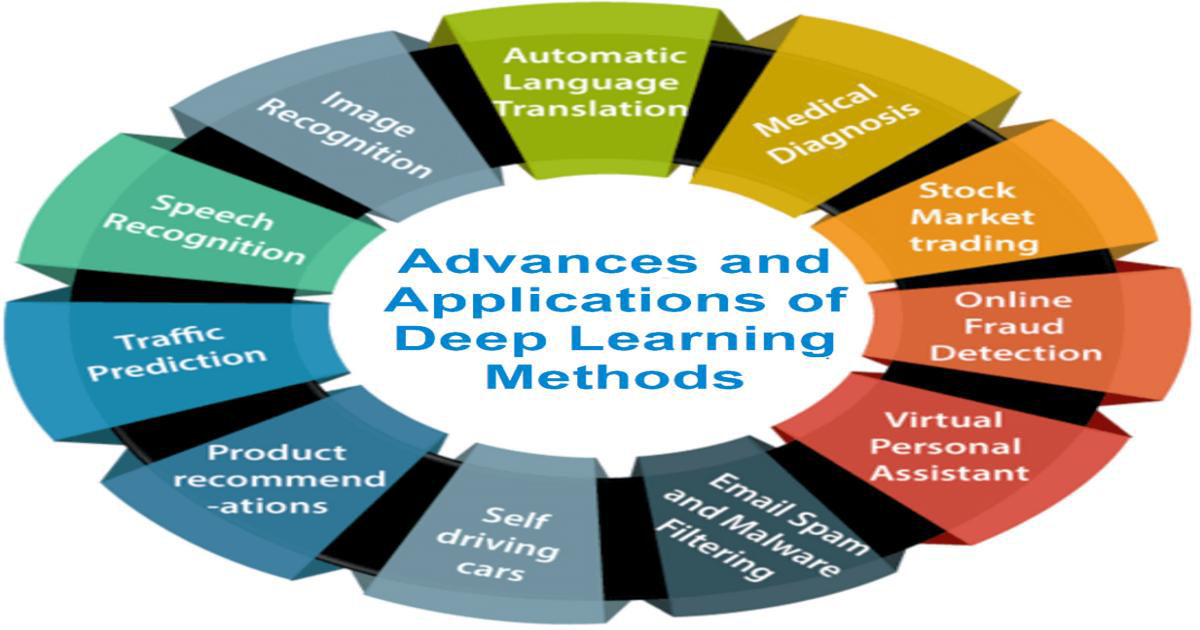
Special Issue Editors
Interests: machine learning; AI
Special Issue Information
Dear Colleagues,
Deep learning has revolutionized the field of image processing since its emergence. As a result, manual feature extraction techniques have become obsolete. The field of deep learning (DL) has been one of the fastest-growing areas in artificial intelligence and data science, with rapidly emerging applications spanning read-scene understanding, medical image analysis, plant phenotyping, textual data modalities, etc. This allows researchers to analyze a variety of signal and information-processing tasks by automatically identifying features. This Special Issue explores the application of deep learning and artificial neural networks to analyze data and make predictions across a wide range of industries and fields and will present a wide variety of deep learning applications, from solving complex data science problems to developing automatic user controls.
The main objective is to bring together deep learning researchers from different disciplines to discuss new ideas, research questions, recent results, and challenges in this emerging area.
Potential topics include but are not limited to:
- Deep learning models for medical image analysis (healthcare);
- Deep learning models for plant phenotyping;
- Deep learning models for road scene understanding (self-driving);
- Deep learning models for fighting deepfakes;
- Deep learning models for pixel restoration;
- Deep learning models for video gaming;
- Deep learning models for online marketing support;
- Deep learning models for user behavior analysis.
In this Special Issue, original research articles and reviews are welcome.
We look forward to receiving your contributions.
Dr. Robail Yasrab
Dr. Md Mostafa Kamal Sarker
Guest Editors
Manuscript Submission Information
Manuscripts should be submitted online at www.mdpi.com by registering and logging in to this website. Once you are registered, click here to go to the submission form. Manuscripts can be submitted until the deadline. All submissions that pass pre-check are peer-reviewed. Accepted papers will be published continuously in the journal (as soon as accepted) and will be listed together on the special issue website. Research articles, review articles as well as short communications are invited. For planned papers, a title and short abstract (about 100 words) can be sent to the Editorial Office for announcement on this website.
Submitted manuscripts should not have been published previously, nor be under consideration for publication elsewhere (except conference proceedings papers). All manuscripts are thoroughly refereed through a single-blind peer-review process. A guide for authors and other relevant information for submission of manuscripts is available on the Instructions for Authors page. Big Data and Cognitive Computing is an international peer-reviewed open access monthly journal published by MDPI.
Please visit the Instructions for Authors page before submitting a manuscript. The Article Processing Charge (APC) for publication in this open access journal is 1800 CHF (Swiss Francs). Submitted papers should be well formatted and use good English. Authors may use MDPI's English editing service prior to publication or during author revisions.
Keywords
- deep learning
- image analysis
- scene understanding
Benefits of Publishing in a Special Issue
- Ease of navigation: Grouping papers by topic helps scholars navigate broad scope journals more efficiently.
- Greater discoverability: Special Issues support the reach and impact of scientific research. Articles in Special Issues are more discoverable and cited more frequently.
- Expansion of research network: Special Issues facilitate connections among authors, fostering scientific collaborations.
- External promotion: Articles in Special Issues are often promoted through the journal's social media, increasing their visibility.
- Reprint: MDPI Books provides the opportunity to republish successful Special Issues in book format, both online and in print.
Further information on MDPI's Special Issue policies can be found here.