Topic Menu
► Topic MenuTopic Editors


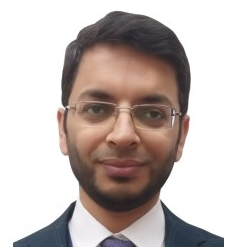

Recent Advances in Computational Optimization Techniques and Their Modern Applications for Smart HealthCare
Topic Information
Dear Colleagues,
Medical and healthcare-related research is moving to new fronts in accuracy due to the growing number of data being acquired in healthcare systems and the convergence of diverse areas of knowledge in the health sector. Along with providing a rare chance and solid assurance for tackling various pressing issues, this trend also has technical applications of artificial intelligence (AI) and operations research (OR). Recently, AI techniques have been researched and used as potentially useful tools for the creation and implementation of intelligent systems inside the healthcare system. By taking into account the undeniable uncertainty of health data and procedures, AI-based systems can often learn from data and adapt according to real-time changes and fluctuations. There have been numerous attempts to date using a variety of techniques, including machine learning (ML), neural networks, optimization, computational intelligence, and human-machine interaction. This Topic Issue invites papers discussing recent advances in the development and application of Optimization, Machine Learning, and Artificial Intelligence in Healthcare.
Dr. Mohd Dilshad Ansari
Prof. Dr. Mohd Fauzi Bin Othman
Dr. Jawad Rasheed
Dr. Mazdak Zamani
Topic Editors
Keywords
- optimization methods
- computational approaches
- machine learning
- artificial intelligence
- smart healthcare system
- heuristic search methods
- automation by AI, ML and DL in healthcare etc.
Participating Journals
Journal Name | Impact Factor | CiteScore | Launched Year | First Decision (median) | APC |
---|---|---|---|---|---|
![]()
Mathematics
|
2.3 | 4.0 | 2013 | 18.3 Days | CHF 2600 |
![]()
Applied Sciences
|
2.5 | 5.3 | 2011 | 18.4 Days | CHF 2400 |
![]()
Electronics
|
2.6 | 5.3 | 2012 | 16.4 Days | CHF 2400 |
![]()
Healthcare
|
2.4 | 3.5 | 2013 | 20.3 Days | CHF 2700 |
![]()
Symmetry
|
2.2 | 5.4 | 2009 | 17.3 Days | CHF 2400 |
Preprints.org is a multidisciplinary platform offering a preprint service designed to facilitate the early sharing of your research. It supports and empowers your research journey from the very beginning.
MDPI Topics is collaborating with Preprints.org and has established a direct connection between MDPI journals and the platform. Authors are encouraged to take advantage of this opportunity by posting their preprints at Preprints.org prior to publication:
- Share your research immediately: disseminate your ideas prior to publication and establish priority for your work.
- Safeguard your intellectual contribution: Protect your ideas with a time-stamped preprint that serves as proof of your research timeline.
- Boost visibility and impact: Increase the reach and influence of your research by making it accessible to a global audience.
- Gain early feedback: Receive valuable input and insights from peers before submitting to a journal.
- Ensure broad indexing: Web of Science (Preprint Citation Index), Google Scholar, Crossref, SHARE, PrePubMed, Scilit and Europe PMC.