Topic Menu
► Topic MenuTopic Editors
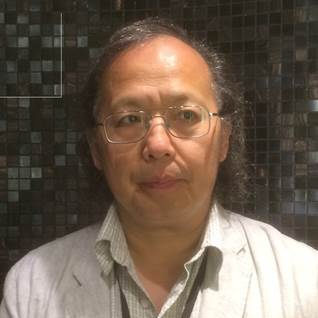

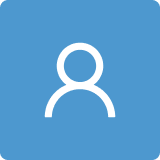
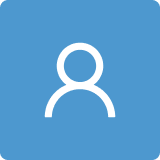
Health Informatics and Epidemiological Data Analysis in COVID-19 Based Internet of Medical Things (HIEDA-COVID19-IOMT)
Topic Information
Dear Colleagues,
In the presence of external environmental factors, COVID-19 has become a worldwide epidemic problem. The journal of the National Academy of Sciences of the United States calculates that the total weight of COVID-19 virus particles is about 100 grams to 10 kilograms, which is between an apple and a child. It is just like a nuclear bomb, with a small mass but capable of devastating destruction. The study stated that each coronavirus particle had a mass of 1 nano coronavirus gram, which calculated that each infected person carried about 1 to 10 micrograms of virus particles at the peak of infection, based on the estimated 10 to 100 billion coronavirus particles per infected person at the peak of infection. IoMT medical applications can also help the patients of COVID-19 keep in touch with doctors or nurses. The intelligent detection means using the Internet of Things technology sends various physical data of patients in real time through the communication module, and at the same time has the optimization of clinical basic nursing work, such as automatic recording, summary, and alarm. In order to further improve the hospitalization experience of patients and improve the quality of medical services, 5G technology and related smart hardware devices based on the Internet, artificial intelligence, and the Internet of Things are used to build mobile nursing, physical sign monitoring, wireless infusion monitoring, and mobile rounds under 5G technology. Then combined with deep learning to analyze and integrate medical data to optimize management methods and rationally allocate medical resources. This Special Issue is aimed at presenting the state-of-the-art, current challenges and future trends for the successful application of artificial intelligence in management of epidemic control of COVID-19 using the IoMT platform. Original contributions considering recent findings in theory, methodologies, and applications in the field of artificial Intelligence and Internet computing in COVID-19 are welcome.
Prof. Dr. Wenjun (Chris) Zhang
Prof. Dr. Dhanjoo N. Ghista
Prof. Dr. Kelvin K.L. Wong
Prof. Dr. Zhili Sun
Topic Editors
Keywords
- artificial intelligence techniques using the HIEDA-COVID19-IOMT in medical imaging
- deep learning models based on HIEDA-COVID19-IOMT
- deep learning based HIEDA-COVID19-IOMT for medical image classification, regression, localization, and segmentation
- content-based medical image retrieval
- computer-aided detection and diagnosis based on HIEDA-COVID19-IOMT
Participating Journals
Journal Name | Impact Factor | CiteScore | Launched Year | First Decision (median) | APC |
---|---|---|---|---|---|
![]()
BioMed
|
- | - | 2021 | 27 Days | CHF 1000 |
![]()
Biomedicines
|
4.7 | 3.7 | 2013 | 15.4 Days | CHF 2600 |
![]()
BioMedInformatics
|
- | - | 2021 | 21 Days | CHF 1000 |
![]()
Epidemiologia
|
- | - | 2020 | 28.4 Days | CHF 1200 |
![]()
Journal of Clinical Medicine
|
3.9 | 5.4 | 2012 | 17.9 Days | CHF 2600 |
MDPI Topics is cooperating with Preprints.org and has built a direct connection between MDPI journals and Preprints.org. Authors are encouraged to enjoy the benefits by posting a preprint at Preprints.org prior to publication:
- Immediately share your ideas ahead of publication and establish your research priority;
- Protect your idea from being stolen with this time-stamped preprint article;
- Enhance the exposure and impact of your research;
- Receive feedback from your peers in advance;
- Have it indexed in Web of Science (Preprint Citation Index), Google Scholar, Crossref, SHARE, PrePubMed, Scilit and Europe PMC.