Topic Menu
► Topic MenuTopic Editors

Artificial Intelligence and Fuzzy Systems
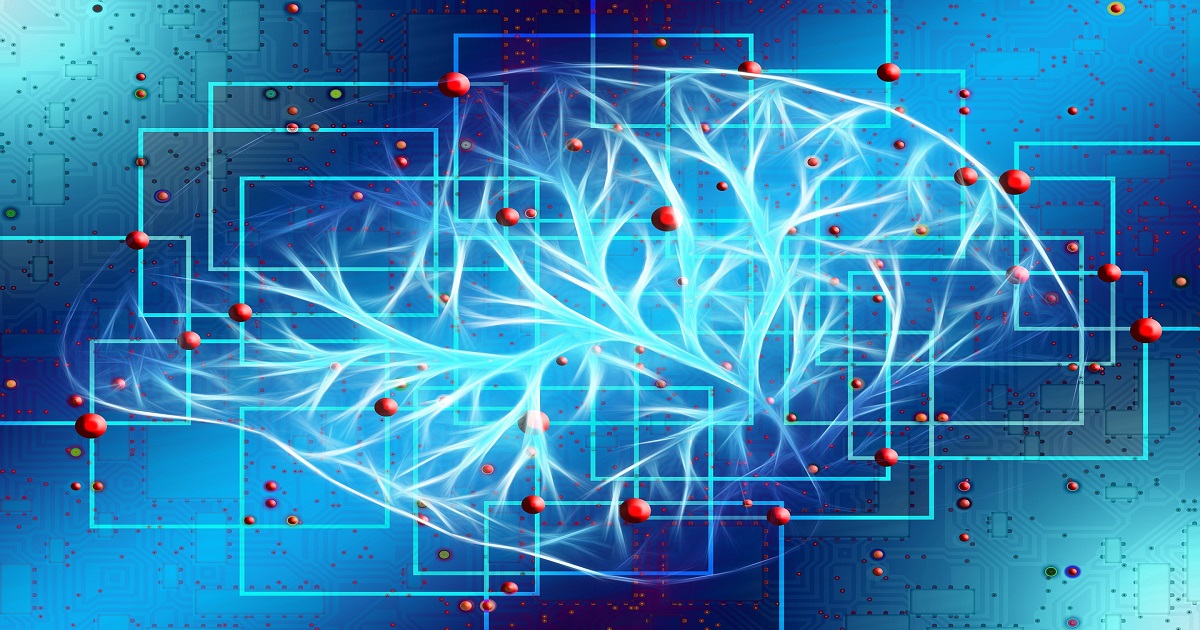
Topic Information
Dear Colleagues,
Artificial intelligence (AI) includes the theory and development of computer systems that are able to perform tasks that require human intelligence, such as problem solving, reasoning, planning, natural language understanding, computer vision, automatic programming, and machine learning, among others. It is increasingly present today. The large amount of information that is currently stored requires specialized techniques for automation, precision, and speed in data analysis. More and more organizations are interested in applying it to improve the effectiveness and efficiency of their business processes.
Indeed, fuzzy systems cover all aspects of the theory and applications of fuzzy sets and systems in addition to their hybridizations with other artificial and computational intelligence techniques. These techniques are becoming more and more relevant because they allow a transparent description of knowledge in terms of linguistic rules. In many real-world applications, fuzzy systems realize precise systems that introduce high interpretability.
This Topic, “Artificial Intelligence and Fuzzy Systems”, invites papers on theoretical and applied issues, including, but not limited to, the following:
- Big data, data engineering, and data analytics.
- Artificial intelligence, machine learning, and cognitive computing.
- Data mining, information retrieval, and business intelligence.
- Artificial intelligence in business, robotics, healthcare, or multimedia technology.
- Artificial neural networks and deep learning.
- Ethics of artificial intelligence.
- Explainable artificial intelligence.
- Interpretable and interactive approaches to uncertainty in AI.
- Fuzzy databases and informational retrieval.
- Theory and applications of fuzzy logic and probabilistic approaches.
- Fuzzy decision analysis, multicriteria decision making and decision support.
- Interdisciplinary field of fuzzy logic and data mining.
This Topic will present the results of research describing recent advances in both the artificial intelligence and the fuzzy systems.
Prof. Dr. Amelia Zafra
Prof. Dr. Jose Manuel Soto Hidalgo
Topic Editors
Keywords
- artificial intelligence
- fuzzy systems
- deep learning
- machine learning
- data mining
Participating Journals
Journal Name | Impact Factor | CiteScore | Launched Year | First Decision (median) | APC |
---|---|---|---|---|---|
![]()
AI
|
3.1 | 7.2 | 2020 | 18.9 Days | CHF 1600 |
![]()
Algorithms
|
1.8 | 4.1 | 2008 | 18.9 Days | CHF 1600 |
![]()
Applied Sciences
|
2.5 | 5.3 | 2011 | 18.4 Days | CHF 2400 |
![]()
Big Data and Cognitive Computing
|
3.7 | 7.1 | 2017 | 25.3 Days | CHF 1800 |
![]()
Machine Learning and Knowledge Extraction
|
4.0 | 6.3 | 2019 | 20.8 Days | CHF 1800 |
![]()
Sensors
|
3.4 | 7.3 | 2001 | 18.6 Days | CHF 2600 |
Preprints.org is a multidisciplinary platform offering a preprint service designed to facilitate the early sharing of your research. It supports and empowers your research journey from the very beginning.
MDPI Topics is collaborating with Preprints.org and has established a direct connection between MDPI journals and the platform. Authors are encouraged to take advantage of this opportunity by posting their preprints at Preprints.org prior to publication:
- Share your research immediately: disseminate your ideas prior to publication and establish priority for your work.
- Safeguard your intellectual contribution: Protect your ideas with a time-stamped preprint that serves as proof of your research timeline.
- Boost visibility and impact: Increase the reach and influence of your research by making it accessible to a global audience.
- Gain early feedback: Receive valuable input and insights from peers before submitting to a journal.
- Ensure broad indexing: Web of Science (Preprint Citation Index), Google Scholar, Crossref, SHARE, PrePubMed, Scilit and Europe PMC.