Artificial Intelligence in Civil Engineering: Latest Advances and Prospects
A special issue of Applied Sciences (ISSN 2076-3417). This special issue belongs to the section "Civil Engineering".
Deadline for manuscript submissions: 20 July 2025 | Viewed by 4287
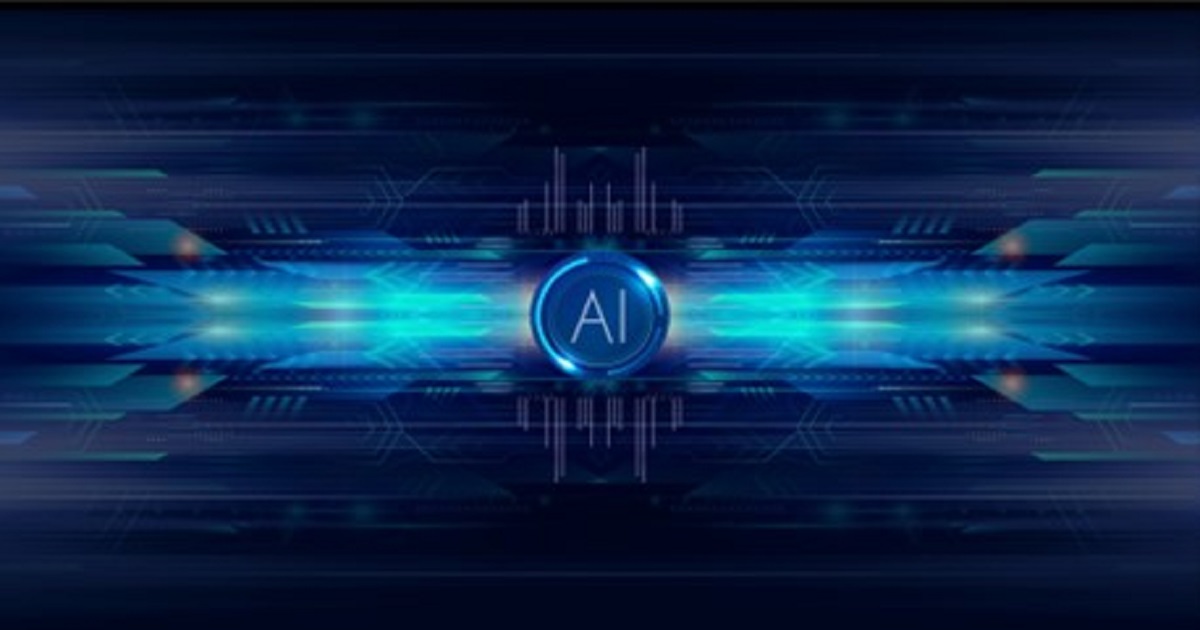
Special Issue Editor
Interests: construction budgeting and cost monitoring and control; information technologies and expert systems in construction management; cost and time prediction models
Special Issues, Collections and Topics in MDPI journals
Special Issue Information
Dear Colleagues,
Nowadays, there are notable technological advancements and innovations that produce significant changes and transformations, providing serious prospects along with challenges, risks, and opportunities. Innovation is incorporated into various sectors such as the engineering field. This integration of new tools, techniques, methodologies, and approaches is drastically changing the traditional engineering practice, transforming both processes and procedures. Essentially, scientific breakthroughs and leaps revolutionize all project life cycles. In this Special Issue, the focus is on Artificial Intelligence (AI). The synergies of AI and civil engineering facilitate a reengineering of project management.
All project phases, from planning and construction, to operation, maintenance, and finally removal of the project, are taken into consideration. The aim is also to apply AI early within the business planning of project enterprises to achieve sustainable development and environmentally friendly projects with minimum externalities. Case studies of a plethora of project types are welcomed, including but not limited to building projects, highway/road projects, geotechnical projects, and hydraulic projects, both private and state owned.
It is interesting to highlight the established or potential synergies among AI and project management sectors such as human resource, scope, time, cost, risk, quality, integration, procurement, communication, earned value, and health and safety management.
The following topics in association with artificial intelligence could complement and elaborate on the previously mentioned areas of interest. More specifically: project cost and time, project quality, simulation, mathematical programming, digital twins, indoor environmental quality, user comfort and well-being, energy efficiency, building and project performance, intelligent design—urban planning, deep learning with satellite and aerial imagery, optimization, fuzzy decision making, health and safety, structural monitoring, image classification, artificial neural networks, decision support automation, machine learning, and last but not least blockchain technologies.
I look forward to receiving your contributions.
Dr. Georgios N. Aretoulis
Guest Editor
Manuscript Submission Information
Manuscripts should be submitted online at www.mdpi.com by registering and logging in to this website. Once you are registered, click here to go to the submission form. Manuscripts can be submitted until the deadline. All submissions that pass pre-check are peer-reviewed. Accepted papers will be published continuously in the journal (as soon as accepted) and will be listed together on the special issue website. Research articles, review articles as well as short communications are invited. For planned papers, a title and short abstract (about 100 words) can be sent to the Editorial Office for announcement on this website.
Submitted manuscripts should not have been published previously, nor be under consideration for publication elsewhere (except conference proceedings papers). All manuscripts are thoroughly refereed through a single-blind peer-review process. A guide for authors and other relevant information for submission of manuscripts is available on the Instructions for Authors page. Applied Sciences is an international peer-reviewed open access semimonthly journal published by MDPI.
Please visit the Instructions for Authors page before submitting a manuscript. The Article Processing Charge (APC) for publication in this open access journal is 2400 CHF (Swiss Francs). Submitted papers should be well formatted and use good English. Authors may use MDPI's English editing service prior to publication or during author revisions.
Keywords
- civil engineering
- artificial intelligence
- artificial neural networks
- expert systems
- digital twins
- blockchain
- machine learning
- optimization
- decision support automation
- quality control
- road infrastructure
Benefits of Publishing in a Special Issue
- Ease of navigation: Grouping papers by topic helps scholars navigate broad scope journals more efficiently.
- Greater discoverability: Special Issues support the reach and impact of scientific research. Articles in Special Issues are more discoverable and cited more frequently.
- Expansion of research network: Special Issues facilitate connections among authors, fostering scientific collaborations.
- External promotion: Articles in Special Issues are often promoted through the journal's social media, increasing their visibility.
- e-Book format: Special Issues with more than 10 articles can be published as dedicated e-books, ensuring wide and rapid dissemination.
Further information on MDPI's Special Issue policies can be found here.