Cloud Computing and Distributed Big Data Analytics
A special issue of Applied Sciences (ISSN 2076-3417). This special issue belongs to the section "Computing and Artificial Intelligence".
Deadline for manuscript submissions: closed (20 January 2024) | Viewed by 2260
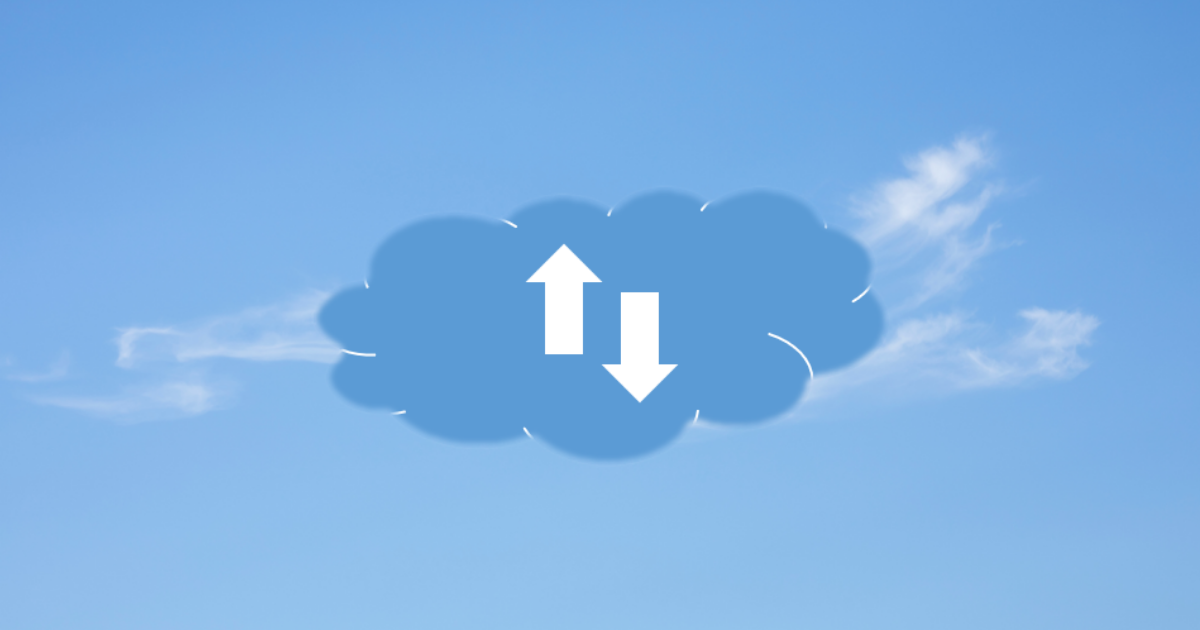
Special Issue Editor
Special Issue Information
Dear Colleagues,
Both cloud computing and big data play a huge role in our digital society. In particularly, cloud computing allows us to easily process big data via a “software as a service” model. In turn, big data also greatly fuel cloud-computing-based application development. The two, linked together, allow people with great ideas but limited resources a chance at business success. They also allow established businesses to utilize data that they collect but previously had no way of analyzing. However, due to the exponentially increasing data and the emerging computing demands in the post-pandemic era, cloud computing and big data applications are facing enormous challenges in many aspects, such as infrastructure, system design, computing paradigm, trust, security and privacy, etc.
In this Special Issue, recent efforts and advances made for cloud computing, big data, and their applications will be discussed. The topics of interest for this Special Issue include but are not limited to the following:
- Cloud-native databases and applications;
- Cloud gaming, virtual reality (VR), and augmented reality (AR);
- Artificial intelligence (AI) and AI-driven clouds;
- The Internet of Things, edge computing, and cloud–edge collaboration;
- Distributed clouds and federated learning;
- Serverless computing and edge clouds;
- Trust and Blockchain;
- System and data security;
- Privacy computing and privacy-enhanced technologies.
Dr. Peng Zhao
Guest Editor
Manuscript Submission Information
Manuscripts should be submitted online at www.mdpi.com by registering and logging in to this website. Once you are registered, click here to go to the submission form. Manuscripts can be submitted until the deadline. All submissions that pass pre-check are peer-reviewed. Accepted papers will be published continuously in the journal (as soon as accepted) and will be listed together on the special issue website. Research articles, review articles as well as short communications are invited. For planned papers, a title and short abstract (about 100 words) can be sent to the Editorial Office for announcement on this website.
Submitted manuscripts should not have been published previously, nor be under consideration for publication elsewhere (except conference proceedings papers). All manuscripts are thoroughly refereed through a single-blind peer-review process. A guide for authors and other relevant information for submission of manuscripts is available on the Instructions for Authors page. Applied Sciences is an international peer-reviewed open access semimonthly journal published by MDPI.
Please visit the Instructions for Authors page before submitting a manuscript. The Article Processing Charge (APC) for publication in this open access journal is 2400 CHF (Swiss Francs). Submitted papers should be well formatted and use good English. Authors may use MDPI's English editing service prior to publication or during author revisions.
Keywords
- cloud computing
- big data
- cloud-based applications
- data-based AI algorithms
- data-driven applications
- data security
- data privacy
Benefits of Publishing in a Special Issue
- Ease of navigation: Grouping papers by topic helps scholars navigate broad scope journals more efficiently.
- Greater discoverability: Special Issues support the reach and impact of scientific research. Articles in Special Issues are more discoverable and cited more frequently.
- Expansion of research network: Special Issues facilitate connections among authors, fostering scientific collaborations.
- External promotion: Articles in Special Issues are often promoted through the journal's social media, increasing their visibility.
- Reprint: MDPI Books provides the opportunity to republish successful Special Issues in book format, both online and in print.
Further information on MDPI's Special Issue policies can be found here.