Deep Learning and Predictive Maintenance
A special issue of Applied Sciences (ISSN 2076-3417). This special issue belongs to the section "Mechanical Engineering".
Deadline for manuscript submissions: 20 November 2025 | Viewed by 1947
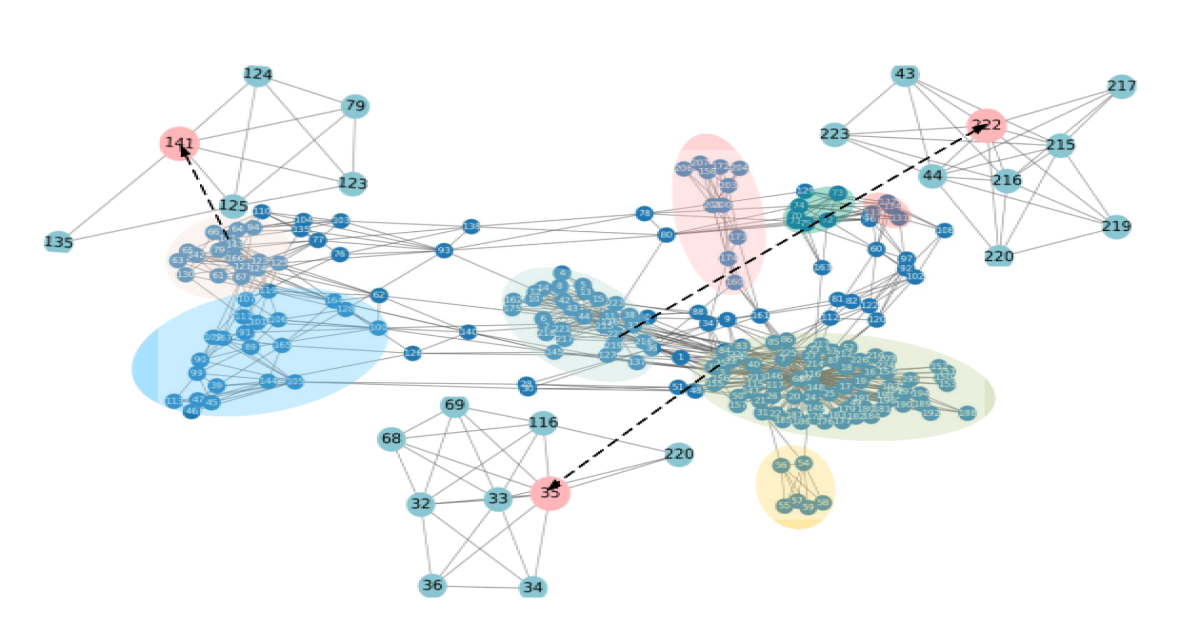
Special Issue Editors
Interests: predictive maintenance and health management
Special Issues, Collections and Topics in MDPI journals
Interests: intelligent operation and maintenance
Interests: health monitoring and device development
Special Issue Information
Dear Colleagues,
This Special Issue, entitled "Deep Learning and Predictive Maintenance", highlights the immense potential of deep learning in achieving precise prediction and efficiently processing large volumes of data. Owing to the widespread application of deep learning across various domains, the capabilities of predictive maintenance are being enhanced. However, a current challenge lies in leveraging deep learning for predictive maintenance in real-world industrial processes. For instance, in industries such as manufacturing, energy, and transportation, predictive maintenance can assist businesses in reducing downtime, lowering maintenance costs, and improving production efficiency. Investigating the status and behavior of equipment during actual industrial processes is a crucial task in predictive maintenance, as it enables early warnings of equipment failures, prevents unexpected shutdowns, and enhances production continuity. Moreover, predictive maintenance facilitated by deep learning can help enterprises plan maintenance schedules effectively and extend the lifespan of equipment.
We are, therefore, interested in articles that explore the application of deep learning for predictive maintenance. Potential topics include, but are not limited to, the following:
- Application cases of deep learning in predictive maintenance;
- Predictive models and algorithms based on deep learning;
- Application of deep learning in fault diagnosis and prediction;
- Utilization of deep learning in equipment condition monitoring and warning systems;
- Application of deep learning in industrial big data analysis;
- Integration of deep learning in maintenance decision support systems;
- Role of deep learning in the Industrial Internet and Industry 4.0;
- Application of deep learning in predictive maintenance in the energy, manufacturing, and transportation sectors;
- Application of deep learning in equipment lifecycle management;
- Challenges and future directions in the field of deep learning for predictive maintenance.
Original work that highlights the latest research and technical developments is encouraged, but review papers and comparative studies are also welcome.
Prof. Dr. Wei Cheng
Dr. Zelin Nie
Dr. Yilong Liu
Dr. Shuming Wu
Guest Editors
Manuscript Submission Information
Manuscripts should be submitted online at www.mdpi.com by registering and logging in to this website. Once you are registered, click here to go to the submission form. Manuscripts can be submitted until the deadline. All submissions that pass pre-check are peer-reviewed. Accepted papers will be published continuously in the journal (as soon as accepted) and will be listed together on the special issue website. Research articles, review articles as well as short communications are invited. For planned papers, a title and short abstract (about 100 words) can be sent to the Editorial Office for announcement on this website.
Submitted manuscripts should not have been published previously, nor be under consideration for publication elsewhere (except conference proceedings papers). All manuscripts are thoroughly refereed through a single-blind peer-review process. A guide for authors and other relevant information for submission of manuscripts is available on the Instructions for Authors page. Applied Sciences is an international peer-reviewed open access semimonthly journal published by MDPI.
Please visit the Instructions for Authors page before submitting a manuscript. The Article Processing Charge (APC) for publication in this open access journal is 2400 CHF (Swiss Francs). Submitted papers should be well formatted and use good English. Authors may use MDPI's English editing service prior to publication or during author revisions.
Keywords
- deep learning
- predictive maintenance
- health managment
- fault diagnosis
- remaining useful life
- monitoring and warning
Benefits of Publishing in a Special Issue
- Ease of navigation: Grouping papers by topic helps scholars navigate broad scope journals more efficiently.
- Greater discoverability: Special Issues support the reach and impact of scientific research. Articles in Special Issues are more discoverable and cited more frequently.
- Expansion of research network: Special Issues facilitate connections among authors, fostering scientific collaborations.
- External promotion: Articles in Special Issues are often promoted through the journal's social media, increasing their visibility.
- Reprint: MDPI Books provides the opportunity to republish successful Special Issues in book format, both online and in print.
Further information on MDPI's Special Issue policies can be found here.