Nanophotonic Devices and Technologies
A special issue of Applied Sciences (ISSN 2076-3417). This special issue belongs to the section "Optics and Lasers".
Deadline for manuscript submissions: closed (28 February 2022) | Viewed by 12491
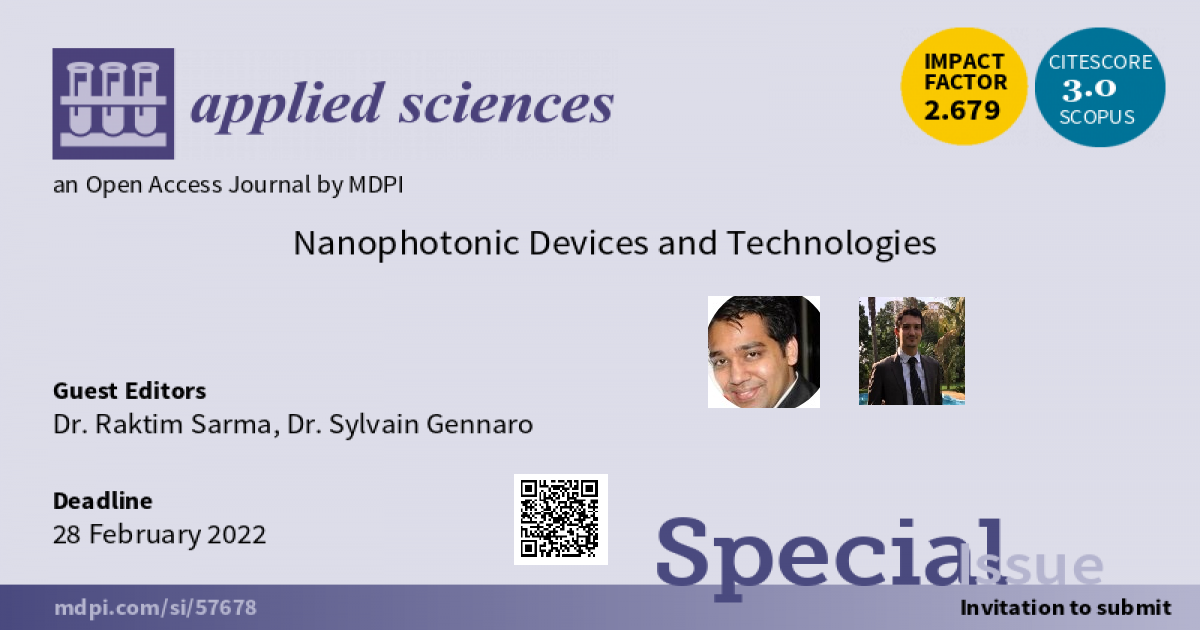
Special Issue Editors
Interests: mesoscopic physics; wave propagation in complex media; nanophotonics; optical gyroscopes and sensors; optoelectronics; tunable and nonlinear metamaterials
Special Issue Information
Dear Colleagues,
Light–matter interaction at the nanoscale is an extensively studied topic both for fundamental reasons as well as for practical applications ranging from sensing, biomedical imaging, energy harvesting, holography, and new light sources to lasers. The complexity of light–matter interaction in complex media originates from the spatially varying refractive index profile of the photonic medium. The refractive index profile can be spatially uncorrelated for random media, aperiodic, or periodic for metasurfaces and photonic crystals. While random media are extensively studied for applications ranging from biomedical imaging, lasing, to energy harvesting, periodic structures, such as metasurfaces, have made it possible to control light–matter interactions in ways that were not possible before.
In this Special Edition, our focus will be on advances in the areas of controlling light–matter interactions at the nanoscale using complex photonic media such as random media, photonic crystals, metamaterials, and metasurfaces. We are seeking publications in the form of original research articles or review papers addressing recent advancements in the field of light–matter interaction at the nanoscale.
Sincerely,
Dr. Raktim Sarma
Dr. Sylvain Gennaro
Guest Editors
Manuscript Submission Information
Manuscripts should be submitted online at www.mdpi.com by registering and logging in to this website. Once you are registered, click here to go to the submission form. Manuscripts can be submitted until the deadline. All submissions that pass pre-check are peer-reviewed. Accepted papers will be published continuously in the journal (as soon as accepted) and will be listed together on the special issue website. Research articles, review articles as well as short communications are invited. For planned papers, a title and short abstract (about 100 words) can be sent to the Editorial Office for announcement on this website.
Submitted manuscripts should not have been published previously, nor be under consideration for publication elsewhere (except conference proceedings papers). All manuscripts are thoroughly refereed through a single-blind peer-review process. A guide for authors and other relevant information for submission of manuscripts is available on the Instructions for Authors page. Applied Sciences is an international peer-reviewed open access semimonthly journal published by MDPI.
Please visit the Instructions for Authors page before submitting a manuscript. The Article Processing Charge (APC) for publication in this open access journal is 2400 CHF (Swiss Francs). Submitted papers should be well formatted and use good English. Authors may use MDPI's English editing service prior to publication or during author revisions.
Keywords
- Control and study of light transport through random media
- Studies of employing adaptive wavefront shaping techniques for controlling light
- transport and for imaging applications in turbid media
- Photonic and sensing applications using correlated disordered structures
- Wavefront control, sensing, nonlinear optical effects, and novel optical
- phenomena using metasurfaces and metamaterials
- Photonic crystals
- Topological and non-reciprocal photonics
- Non-Hermitian Optics and P–T symmetric systems
- Phase-change materials for photonic applications
- New materials for tunable photonics
- Photonics with atomically thin materials