Future Autonomous Vehicles and Their Systems
A special issue of Applied Sciences (ISSN 2076-3417). This special issue belongs to the section "Transportation and Future Mobility".
Deadline for manuscript submissions: closed (20 January 2025) | Viewed by 18618
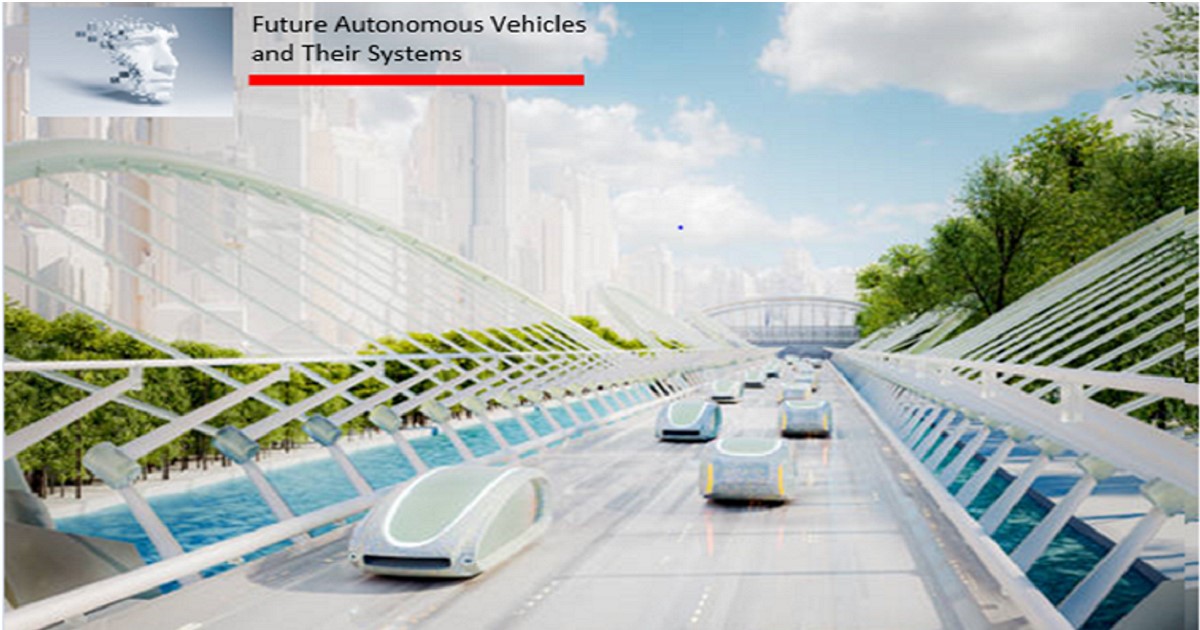
Special Issue Editors
Interests: artificial intelligence; deep learning; mobile robotics; environment perception; computer vision; embedded systems; autonomous vehicles; smart mobility
Special Issues, Collections and Topics in MDPI journals
Interests: computer vision; localization; artificial intelligence; calibration; autonomous vehicle; image processing; mobile robotics
Special Issues, Collections and Topics in MDPI journals
Interests: algorithmic geometry; pattern recognition; computer vision; artificial intelligence; image processing; machine learning; deep learning
Special Issues, Collections and Topics in MDPI journals
2. EPI Inria DISCO, Laboratoire des Signaux et Systèmes, CNRS-CentraleSupélec, 91192 Gif-sur-Yvette, France
Interests: observers design; nonlinear systems; neur adaptive observers; robust control; machine learning; artificial intelligence; robust observers
Special Issues, Collections and Topics in MDPI journals
Special Issue Information
Dear Colleagues,
In the future, autonomous systems, encompassing mobile robots, cars, drones, trains, boats, and more, will become increasingly integrated into our daily lives, reshaping the landscape of smart mobility. The core of an effective autonomous navigation system lies in its ability to perform three essential functions: perception, decision-making, and action execution. By perceiving and comprehending its environment, a vehicle can make informed decisions regarding its actions, ultimately leading to the execution of high-quality maneuvers. As autonomous vehicles continue to evolve, the development process brings forth new challenges that demand innovative solutions in both software and hardware domains.
To address these challenges and pave the way for the advancement of autonomous vehicles, this Special Issue aims to foster the exploration of novel ideas and experimental results in the field. A primary focus of this endeavor is to ensure that autonomous vehicles meet the crucial requirements of safety, comfort, and energy efficiency. Future autonomous vehicle systems necessary to achieve high performance in real-time embedded systems, very high accuracy, energy consumption optimization, robust control decisions, and very high actions are also topics of interest.
The scope of this Special Issue covers a diverse range of areas relevant to the future of autonomous vehicles and their systems. These areas include, but are not limited to:
- Environment perception: Research and advancements in techniques that enable autonomous vehicles to accurately perceive and understand their surroundings. This involves utilizing various sensors and technologies to capture and interpret relevant environmental data.
- Object detection and tracking: Developing robust algorithms and methodologies for detecting and tracking objects in the vehicle's environment. This includes the identification and tracking of pedestrians, vehicles, obstacles, and other relevant entities.
- Semantic segmentation: Exploring approaches to segment and label different elements in the environment, allowing for a more comprehensive understanding of the scene. This includes assigning semantic labels to regions, objects, and other relevant components.
- Localization: Investigating techniques and methods for accurately determining the vehicle's precise position in real-time. This involves utilizing sensors, such as GPS, LiDAR, and cameras, along with advanced algorithms for localization.
- Autonomous navigation: Designing intelligent systems and algorithms that enable vehicles to navigate autonomously in various scenarios and terrains. This includes path planning, obstacle avoidance, and decision-making processes.
- Multisensor fusion systems: Developing techniques for integrating data from multiple sensors to enhance the perception capabilities of autonomous vehicles. This includes sensor fusion algorithms, data fusion methodologies, and fusion architectures.
- Machine learning and artificial intelligence: Leveraging machine learning and AI algorithms to enhance the performance and decision-making capabilities of autonomous vehicles. This includes developing models for object recognition, behavior prediction, and intelligent control systems.
- Datasets and scientific experiments: Creating and utilizing large-scale datasets for training and evaluating autonomous vehicle systems. This includes collecting real-world data, designing benchmark datasets, and conducting scientific experiments to validate the performance and robustness of autonomous systems.
- Collecting and processing high-voluminous datasets: Developing techniques and frameworks to handle and process the large volumes of data generated by autonomous vehicles. This involves efficient data storage, data compression, and data processing methodologies.
- Connected vehicles (V2X): Exploring the integration of autonomous vehicles with the surrounding infrastructure and other connected vehicles. This includes vehicle-to-vehicle (V2V), vehicle-to-infrastructure (V2I), and vehicle-to-everything (V2X) communication technologies for enhanced safety and coordination.
- Internet of Things (IoT): Investigating the potential of IoT technologies in the context of autonomous vehicles. This includes utilizing IoT devices and platforms for data exchange, real-time monitoring, and enhanced vehicle connectivity.
Furthermore, future autonomous systems must strive for high performance in real-time embedded systems. This involves achieving very high accuracy in perception and decision-making tasks, optimizing energy consumption for prolonged operation, ensuring robust control decisions in dynamic environments, and executing precise actions with minimal latency.
By delving into these areas of research and innovation, this Special Issue aims to foster the development of autonomous vehicles that meet the highest standards of safety, comfort, and energy efficiency, thus shaping a future where intelligent and self-driving vehicles seamlessly integrate into our daily lives.
Therefore, this Special Issue will publish high-quality, original research papers in the overlapping fields of:
- Artificial intelligence, machine learning, and deep learning;
- Reinforcement learning;
- Environment perception;
- Cooperative, augmented, and agnostic perception;
- Object detection and localization;
- Real-time tracking;
- Localization;
- Semantic segmentation;
- Scenes analysis and understanding;
- Control, optimization, and prediction;
- Neuro-adaptive observers and nonlinear controllers;
- Vehicle localization and autonomous navigation;
- Security, privacy, and safety systems;
- Cyberattacks systems;
- Multisensor fusion systems;
- V2X communications and networks;
- Sensors and detectors;
- Human factors and HMI;
- Embedded systems;
- Traffic modeling;
- Autonomous vehicle and users interactions.
Dr. Redouane Khemmar
Prof. Dr. Rémi Boutteau
Dr. Karim Hammoudi
Prof. Dr. Ali Zemouche
Guest Editors
Manuscript Submission Information
Manuscripts should be submitted online at www.mdpi.com by registering and logging in to this website. Once you are registered, click here to go to the submission form. Manuscripts can be submitted until the deadline. All submissions that pass pre-check are peer-reviewed. Accepted papers will be published continuously in the journal (as soon as accepted) and will be listed together on the special issue website. Research articles, review articles as well as short communications are invited. For planned papers, a title and short abstract (about 100 words) can be sent to the Editorial Office for announcement on this website.
Submitted manuscripts should not have been published previously, nor be under consideration for publication elsewhere (except conference proceedings papers). All manuscripts are thoroughly refereed through a single-blind peer-review process. A guide for authors and other relevant information for submission of manuscripts is available on the Instructions for Authors page. Applied Sciences is an international peer-reviewed open access semimonthly journal published by MDPI.
Please visit the Instructions for Authors page before submitting a manuscript. The Article Processing Charge (APC) for publication in this open access journal is 2400 CHF (Swiss Francs). Submitted papers should be well formatted and use good English. Authors may use MDPI's English editing service prior to publication or during author revisions.
Keywords
- environment perception
- object detection
- deep learning
- artificial intelligence
- object tracking
- mobiles robotics
- localization
- autonomous vehicles
- scenes analysis and understanding
- smart mobility
Benefits of Publishing in a Special Issue
- Ease of navigation: Grouping papers by topic helps scholars navigate broad scope journals more efficiently.
- Greater discoverability: Special Issues support the reach and impact of scientific research. Articles in Special Issues are more discoverable and cited more frequently.
- Expansion of research network: Special Issues facilitate connections among authors, fostering scientific collaborations.
- External promotion: Articles in Special Issues are often promoted through the journal's social media, increasing their visibility.
- Reprint: MDPI Books provides the opportunity to republish successful Special Issues in book format, both online and in print.
Further information on MDPI's Special Issue policies can be found here.