Advances in Biomedical Image Processing and Diagnostic Techniques
A special issue of Applied Sciences (ISSN 2076-3417). This special issue belongs to the section "Computing and Artificial Intelligence".
Deadline for manuscript submissions: closed (20 March 2023) | Viewed by 3700
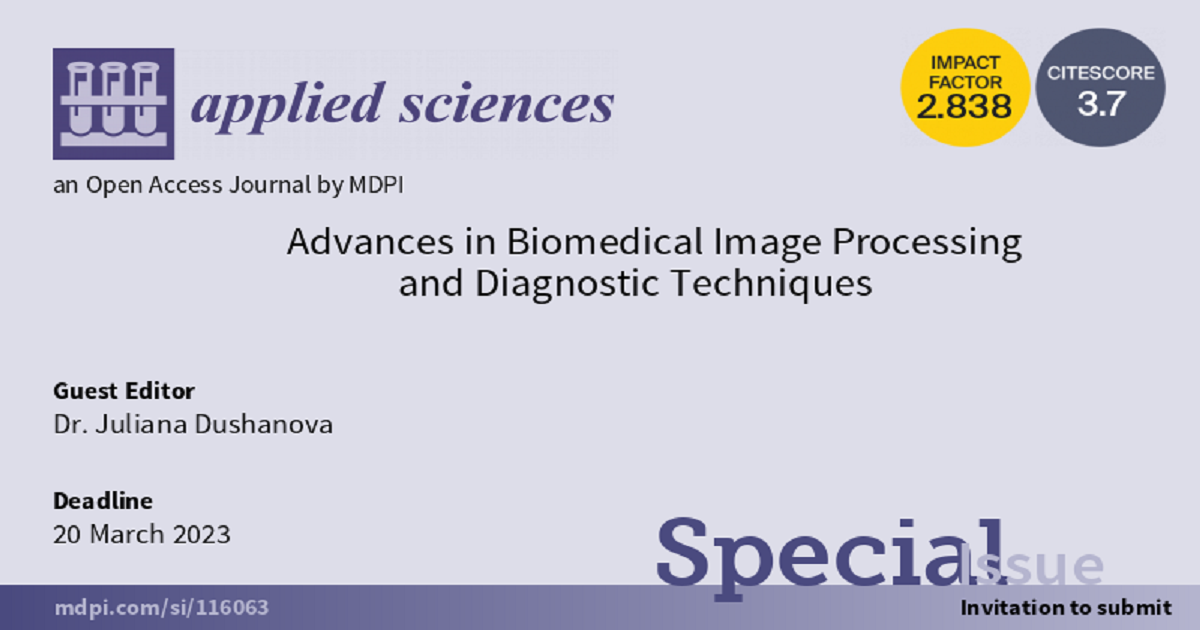
Special Issue Editor
Interests: electrophysiological assessment of brain mechanisms of visual; auditory and cognitive processes; neuropathology in patients with neurological disorders in development and aging
Special Issue Information
Dear Colleagues,
Since the beginning of the 21st century, the connectome has been used in various medical imaging tasks and electrophysiological applications, propelling research into the era of artificial intelligence. This Special Issue will highlight both clinical needs and technical challenges in medical imaging processing and describe how emerging trends in connectome are addressing these issues. Topics covered will include network architecture, learning, interpretability, uncertainty quantification. We are looking to present studies that are related to digital pathology mainly in the brain, particularly prominent research highlights related to clinical study applications. Artificial intelligence and neural networks are significant procedures that tackle this kind of medical diagnosis issue. Moreover, medical imaging processing presents a unique challenge that confronts other connected approaches such as deep learning and different machine learning techniques, which are employed in a variety of tasks along with the different medical image modalities. Disease prediction is one of the basic tasks for medical diagnosis software. As of late, deep learning strategies have been effectively used in different applications to aid medical diagnosis. The contribution of deep learning traits and learning its concepts is helpful for the medical community. We will address research challenges and suggested solutions, as well as future promises and directions for further developments. Proposing new neural network algorithms, different machine learning techniques, and deep learning architectures could improve the detection of brain anomalies, providing timely diagnosis that could reduce the patient's risk for long-term symptoms and lasting deficits. This Special Issue will show the importance of these techniques in medical diagnostics and present future research topics that could be utilized to lead further research.
Dr. Juliana Dushanova
Guest Editor
Manuscript Submission Information
Manuscripts should be submitted online at www.mdpi.com by registering and logging in to this website. Once you are registered, click here to go to the submission form. Manuscripts can be submitted until the deadline. All submissions that pass pre-check are peer-reviewed. Accepted papers will be published continuously in the journal (as soon as accepted) and will be listed together on the special issue website. Research articles, review articles as well as short communications are invited. For planned papers, a title and short abstract (about 100 words) can be sent to the Editorial Office for announcement on this website.
Submitted manuscripts should not have been published previously, nor be under consideration for publication elsewhere (except conference proceedings papers). All manuscripts are thoroughly refereed through a single-blind peer-review process. A guide for authors and other relevant information for submission of manuscripts is available on the Instructions for Authors page. Applied Sciences is an international peer-reviewed open access semimonthly journal published by MDPI.
Please visit the Instructions for Authors page before submitting a manuscript. The Article Processing Charge (APC) for publication in this open access journal is 2400 CHF (Swiss Francs). Submitted papers should be well formatted and use good English. Authors may use MDPI's English editing service prior to publication or during author revisions.
Benefits of Publishing in a Special Issue
- Ease of navigation: Grouping papers by topic helps scholars navigate broad scope journals more efficiently.
- Greater discoverability: Special Issues support the reach and impact of scientific research. Articles in Special Issues are more discoverable and cited more frequently.
- Expansion of research network: Special Issues facilitate connections among authors, fostering scientific collaborations.
- External promotion: Articles in Special Issues are often promoted through the journal's social media, increasing their visibility.
- Reprint: MDPI Books provides the opportunity to republish successful Special Issues in book format, both online and in print.
Further information on MDPI's Special Issue policies can be found here.