AI in Software Engineering: Challenges, Solutions and Applications
A special issue of Applied Sciences (ISSN 2076-3417). This special issue belongs to the section "Computing and Artificial Intelligence".
Deadline for manuscript submissions: closed (20 June 2025) | Viewed by 27374
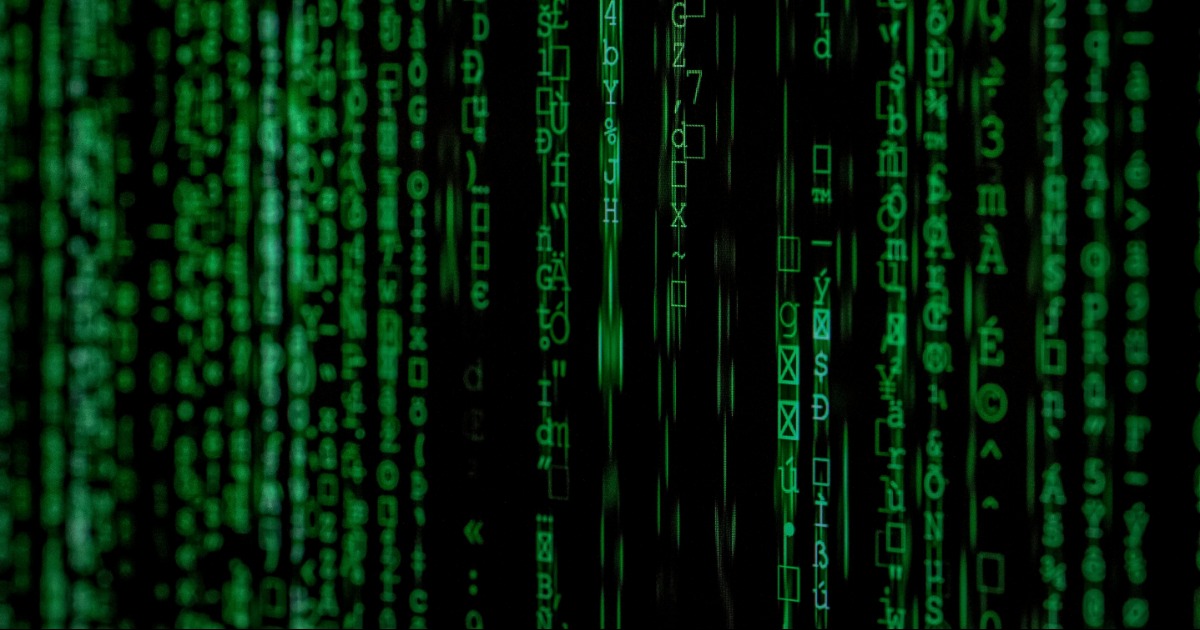
Special Issue Editors
Interests: collective intelligence; deep learning testing and optimization; big data quality; mobile application testing
Special Issues, Collections and Topics in MDPI journals
Interests: crowdsourced testing; mobile application testing; deep learning testing
Special Issues, Collections and Topics in MDPI journals
Special Issue Information
Dear Colleagues,
Researchers and practitioners are invited to submit original studies for the Special Issue on “AI in Software Engineering: Challenges, Solutions and Applications”, which aims to explore the transformative role of artificial intelligence in software engineering, addressing both current challenges and pioneering solutions. We welcome submissions covering AI-driven approaches to software engineering, design, testing, maintenance, and project management. Topics may also include innovative AI applications for code generation, debugging, quality assurance, and enhancing developer productivity. This Special Issue offers a platform for multidisciplinary insights that bridge AI advancements and software engineering needs. Join us in advancing knowledge on AI’s potential to shape the future of software engineering.
We look forward to receiving your contributions.
Prof. Dr. Zhenyu Chen
Dr. Chunrong Fang
Guest Editors
Manuscript Submission Information
Manuscripts should be submitted online at www.mdpi.com by registering and logging in to this website. Once you are registered, click here to go to the submission form. Manuscripts can be submitted until the deadline. All submissions that pass pre-check are peer-reviewed. Accepted papers will be published continuously in the journal (as soon as accepted) and will be listed together on the special issue website. Research articles, review articles as well as short communications are invited. For planned papers, a title and short abstract (about 100 words) can be sent to the Editorial Office for announcement on this website.
Submitted manuscripts should not have been published previously, nor be under consideration for publication elsewhere (except conference proceedings papers). All manuscripts are thoroughly refereed through a single-blind peer-review process. A guide for authors and other relevant information for submission of manuscripts is available on the Instructions for Authors page. Applied Sciences is an international peer-reviewed open access semimonthly journal published by MDPI.
Please visit the Instructions for Authors page before submitting a manuscript. The Article Processing Charge (APC) for publication in this open access journal is 2400 CHF (Swiss Francs). Submitted papers should be well formatted and use good English. Authors may use MDPI's English editing service prior to publication or during author revisions.
Keywords
- software engineering
- software testing
- software quality assurance
- artificial intelligence
- collective intelligence
- application testing
- crowdsourced testing
- deep learning testing
Benefits of Publishing in a Special Issue
- Ease of navigation: Grouping papers by topic helps scholars navigate broad scope journals more efficiently.
- Greater discoverability: Special Issues support the reach and impact of scientific research. Articles in Special Issues are more discoverable and cited more frequently.
- Expansion of research network: Special Issues facilitate connections among authors, fostering scientific collaborations.
- External promotion: Articles in Special Issues are often promoted through the journal's social media, increasing their visibility.
- Reprint: MDPI Books provides the opportunity to republish successful Special Issues in book format, both online and in print.
Further information on MDPI's Special Issue policies can be found here.