New Insights in Learning Analytics
A special issue of Analytics (ISSN 2813-2203).
Deadline for manuscript submissions: closed (31 December 2023) | Viewed by 13584
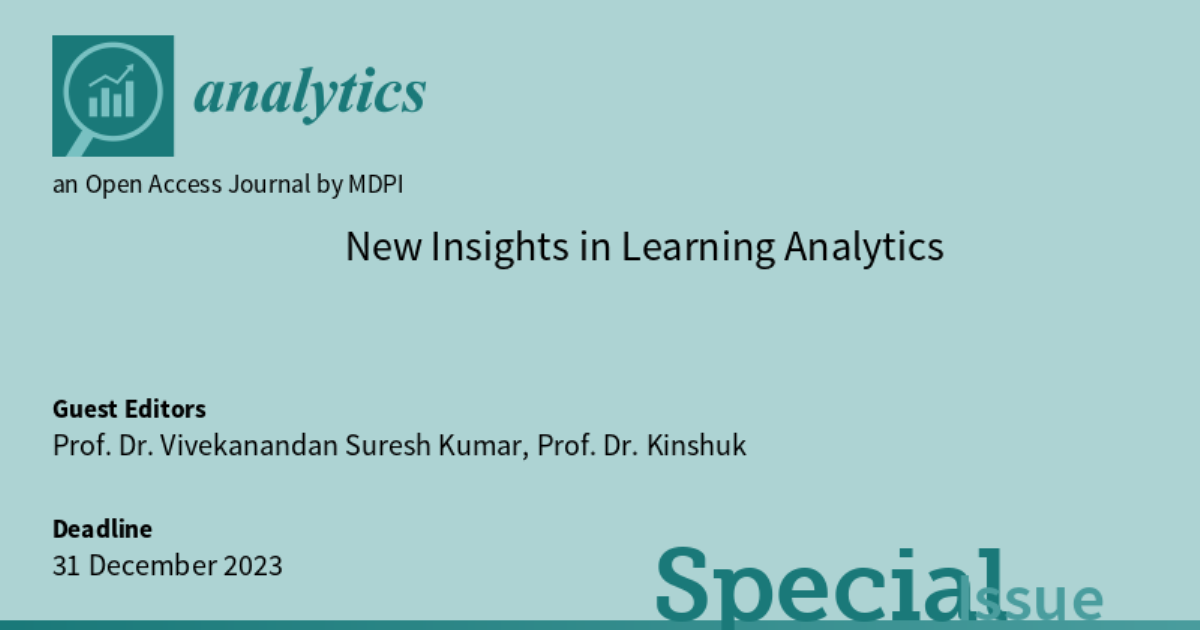
Special Issue Editors
Interests: big data learning analytics; artificial intelligence; self-regulated and co-regulated learning; human-computer interaction; causal modelling
Special Issue Information
Dear Colleagues,
Akin to collecting intelligence on individuals for national security purposes, learning analytics collects intelligence on humans and provides insightful frameworks for the proper application of the same. Observing learning engagements in study episodes, LA agents/sensors tend to collect ethically clean and time-sensitive data over these episodes. LA then exacts actionable insights from these securely communicated datasets to infer information and create models, yielding actionable insights. Specific actions can then be carried out considering these insights, with the impact of those actions measuring the quality of learning. Generating intelligence that helps humans succeed in learning is the singular goal of learning analytics.
Learning Science explores the nature of learning as an interweaving of theories, models, algorithms, traits, datasets, practices, and insights originating from areas such as computing, mathematics, health, education, sociology, humanities, and statistics. Learning Analytics(LA) is an emerging area of study in learning science. LA offers a meta-level view on the quality of human learning with respect to influencing variables of interest. Technology enhancement, cognition and meta-cognition, pedagogy and andragogy, end-user competences, learning environments as well as governance and policymaking are some of the key influencing variables.
In general, LA derives intelligence on the quality of human learning from educational data. Meta-level views of LA include the measurements and relations about the utility, optimality, generalizability, intervention, explainability, and customizability, among other ‘unknown common truths’ pertaining to the quality of human learning. For instance, LA intelligence concerns learners’ capacity, challenges, progress, accomplishments, and the effectiveness of learning contexts, approaching the elusive causality on learning quality as a function of contextual factors such as instructional effectiveness and adaptability. As another example, LA intelligence technology enhancement concerns computational entities, learning machines, and technological gadgets, among others, as well as their impact on the quality of learner interaction.
This Special Issue invites research contributions on learning analytics. Whether research focuses on tracing skills development or metacognition in human learning, whether it focuses on face-to-face, online or blended environments, this Issue seeks to share new insights in the field of LA.
Topics of interest include:
A. Learning analytic theory and science
- Analysis of unstructured and semi-structured data;
- Security, privacy, and veracity of analytics;
- Ethics of learning analytics;
- Scalability of machine learning and data mining for analytics;
- Computing infrastructure for analytics—cloud, grid, autonomic, stream, mobile, high-performance computing;
- Search in learning traces;
- Artificial intelligence in learning analytics;
- Uncertainty handling in analytics;
- IoT and learning analytics.
B. Applications of learning analytics
- Detecting students’ approach to learning;
- Analytics in academic administration;
- Analytics in complex training;
- Gaming analytics and sports analytics;
- Evidence-driven instruction in interdisciplinary and single-discipline areas;
- Big data and educational technology;
- Analytics in academic strategic planning;
- Cultural analytics;
- Large-scale social networks;
- Data literacy;
- Technological literacy and analytics;
- Human literacy and analytics.
C. Techniques and technology in learning analytics
- Evidence-driven mixed-initiative learning;
- Data-intensive learning and instructional design;
- Emerging standards in learning analytics;
- Sentiment analysis;
- Large-scale productivity analysis;
- Analytic infrastructure for academic institutions;
- Scalable knowledge management;
- Research methods for learning analytics;
- Immersive learning.
Prof. Dr. Vivekanandan Suresh Kumar
Prof. Dr. Kinshuk
Guest Editors
Manuscript Submission Information
Manuscripts should be submitted online at www.mdpi.com by registering and logging in to this website. Once you are registered, click here to go to the submission form. Manuscripts can be submitted until the deadline. All submissions that pass pre-check are peer-reviewed. Accepted papers will be published continuously in the journal (as soon as accepted) and will be listed together on the special issue website. Research articles, review articles as well as short communications are invited. For planned papers, a title and short abstract (about 100 words) can be sent to the Editorial Office for announcement on this website.
Submitted manuscripts should not have been published previously, nor be under consideration for publication elsewhere (except conference proceedings papers). All manuscripts are thoroughly refereed through a single-blind peer-review process. A guide for authors and other relevant information for submission of manuscripts is available on the Instructions for Authors page. Analytics is an international peer-reviewed open access quarterly journal published by MDPI.
Please visit the Instructions for Authors page before submitting a manuscript. The Article Processing Charge (APC) for publication in this open access journal is 1000 CHF (Swiss Francs). Submitted papers should be well formatted and use good English. Authors may use MDPI's English editing service prior to publication or during author revisions.
Benefits of Publishing in a Special Issue
- Ease of navigation: Grouping papers by topic helps scholars navigate broad scope journals more efficiently.
- Greater discoverability: Special Issues support the reach and impact of scientific research. Articles in Special Issues are more discoverable and cited more frequently.
- Expansion of research network: Special Issues facilitate connections among authors, fostering scientific collaborations.
- External promotion: Articles in Special Issues are often promoted through the journal's social media, increasing their visibility.
- e-Book format: Special Issues with more than 10 articles can be published as dedicated e-books, ensuring wide and rapid dissemination.
Further information on MDPI's Special Issue policies can be found here.