Topic Menu
► Topic MenuTopic Editors

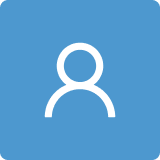
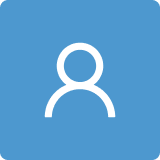
Hybrid Computational Methods in Materials Engineering
Topic Information
Dear Colleagues,
In recent years, there has been a dynamic development of methods and tools enabling the modeling and simulation of technological processes of manufacturing, processing and shaping the structure and properties of engineering materials such as steel and metal alloys, composites, plastics, and ceramics. The use of computational methods in engineering is related, inter alia, to the optimization of material production technology to achieve the desired material properties. This requires establishing the relationship between process parameters, material structure, and properties, and is often based on the analysis of existing experimental data sets. Considering the enormous potential and undoubted benefits of using computational methods in materials engineering, there are more and more examples of their combination in the so-called hybrid methods. These examples concern the connection of, for example, neural networks and evolutionary algorithms, the finite element method and the cellular automaton method, statistical methods and neural networks, or the finite element method and neural networks. Such combined hybrid methods are mainly used to analyze phenomena where their full physical description is not possible. This Topic is dedicated to the application of hybrid computational methods in the engineering of metallic and other materials, including statistical methods, artificial intelligence methods, biologically inspired methods, finite element/boundary/volume methods, data mining, machine learning, cellular automata and image analysis.
Prof. Dr. Wojciech Sitek
Prof. Dr. Jacek Trzaska
Prof. Dr. Imre Felde
Topic Editors
Keywords
- engineering materials
- alloys
- metals
- steels
- composites
- plastics
- ceramics
- material properties
- microstructure
- hybrid computational methods
- artificial intelligence methods
- mathematical modeling
- computer simulation
- data-driven modeling
- machine learning
Participating Journals
Journal Name | Impact Factor | CiteScore | Launched Year | First Decision (median) | APC |
---|---|---|---|---|---|
![]()
AI
|
5.0 | 6.9 | 2020 | 20.7 Days | CHF 1600 |
![]()
Alloys
|
- | 3.2 | 2022 | 24.7 Days | CHF 1000 |
![]()
Applied Sciences
|
2.5 | 5.5 | 2011 | 19.8 Days | CHF 2400 |
![]()
Materials
|
3.2 | 6.4 | 2008 | 15.2 Days | CHF 2600 |
![]()
Metals
|
2.5 | 5.3 | 2011 | 18 Days | CHF 2600 |
Preprints.org is a multidisciplinary platform offering a preprint service designed to facilitate the early sharing of your research. It supports and empowers your research journey from the very beginning.
MDPI Topics is collaborating with Preprints.org and has established a direct connection between MDPI journals and the platform. Authors are encouraged to take advantage of this opportunity by posting their preprints at Preprints.org prior to publication:
- Share your research immediately: disseminate your ideas prior to publication and establish priority for your work.
- Safeguard your intellectual contribution: Protect your ideas with a time-stamped preprint that serves as proof of your research timeline.
- Boost visibility and impact: Increase the reach and influence of your research by making it accessible to a global audience.
- Gain early feedback: Receive valuable input and insights from peers before submitting to a journal.
- Ensure broad indexing: Web of Science (Preprint Citation Index), Google Scholar, Crossref, SHARE, PrePubMed, Scilit and Europe PMC.