Topic Editors
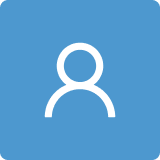
Research on Data Mining of Electronic Health Records Using Deep Learning Methods
Topic Information
Dear Colleagues,
With the explosive expansion of medical information, the era of Health 4.0 has arrived, encompassing a flood of medical data, such as electronic health records, medical images, continuous digital recordings of vital signs, etc. The integration of artificial intelligence into this field is thus unavoidable. However, frontline medical healthcare workers have a limited knowledge of coding, deep learning, and artificial intelligence, creating an unseen barrier to efficient application due to fear of replacement. Thus, for this Special Issue, we are inviting well-regarded physicians, informatics technology scientists, computer science engineers, and medical internet of things specialists to share their experience and studies in this field. Explaining the mys-tery of data mining could lead the way to improved healthcare quality.
Dr. Dawei Yang
Dr. Yu Zhu
Dr. Hongyi Xin
Topic Editors
Keywords
- data mining
- electronic health records
- deep learning
- artificial intelligence
- medical internet of things
- metaverse in medicine
- telemedicine
- ehealth
- screening
Participating Journals
Journal Name | Impact Factor | CiteScore | Launched Year | First Decision (median) | APC | |
---|---|---|---|---|---|---|
![]()
Algorithms
|
1.8 | 4.1 | 2008 | 18.9 Days | CHF 1600 | Submit |
![]()
Future Internet
|
2.8 | 7.1 | 2009 | 16.9 Days | CHF 1600 | Submit |
![]()
Information
|
2.4 | 6.9 | 2010 | 16.4 Days | CHF 1600 | Submit |
![]()
Mathematics
|
2.3 | 4.0 | 2013 | 18.3 Days | CHF 2600 | Submit |
![]()
Symmetry
|
2.2 | 5.4 | 2009 | 17.3 Days | CHF 2400 | Submit |
Preprints.org is a multidisciplinary platform offering a preprint service designed to facilitate the early sharing of your research. It supports and empowers your research journey from the very beginning.
MDPI Topics is collaborating with Preprints.org and has established a direct connection between MDPI journals and the platform. Authors are encouraged to take advantage of this opportunity by posting their preprints at Preprints.org prior to publication:
- Share your research immediately: disseminate your ideas prior to publication and establish priority for your work.
- Safeguard your intellectual contribution: Protect your ideas with a time-stamped preprint that serves as proof of your research timeline.
- Boost visibility and impact: Increase the reach and influence of your research by making it accessible to a global audience.
- Gain early feedback: Receive valuable input and insights from peers before submitting to a journal.
- Ensure broad indexing: Web of Science (Preprint Citation Index), Google Scholar, Crossref, SHARE, PrePubMed, Scilit and Europe PMC.