Deep Learning-Based Biometric Recognition
A special issue of Applied Sciences (ISSN 2076-3417). This special issue belongs to the section "Computing and Artificial Intelligence".
Deadline for manuscript submissions: closed (31 December 2021) | Viewed by 24026
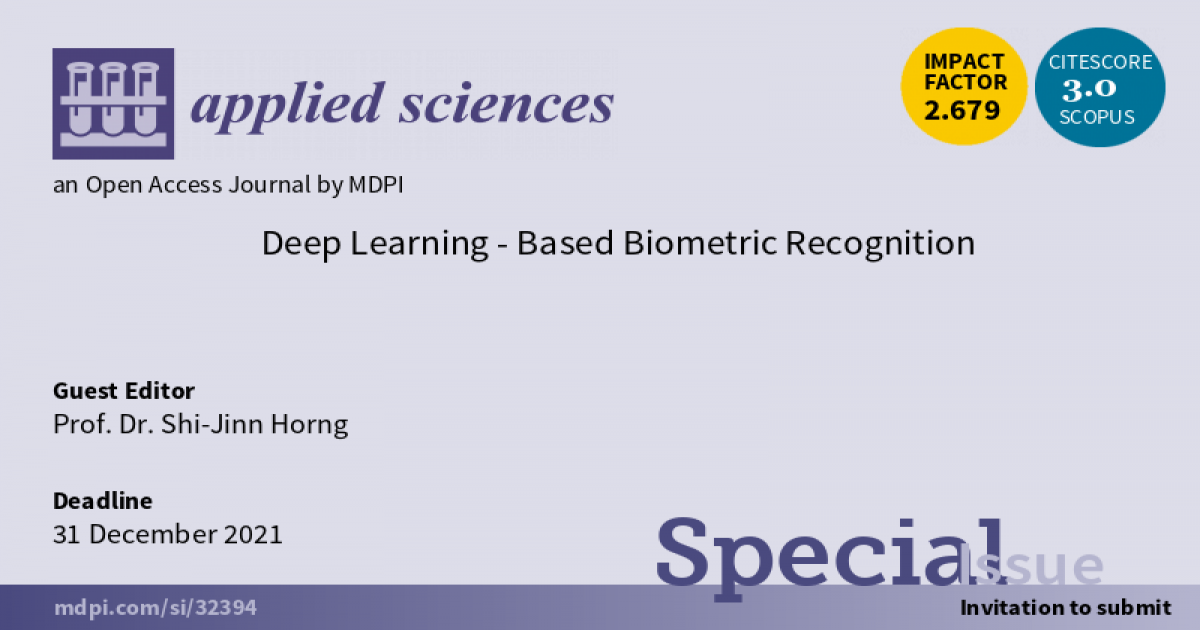
Special Issue Editor
Interests: deep learning and big data; biometric recognition; information security; cloud and fault computing; multimedia applications; medical applications
Special Issues, Collections and Topics in MDPI journals
Special Issue Information
Dear Colleagues,
Deep learning is one of machine learning methods based on artificial neural networks. During the past few years, with the advances in deep learning, many new computation models have been proposed and significantly applied in speech processing, language processing, computer vision, information security, etc.
Biometric authentication is part of a broader family of information security. Biometrics authentication is used in computer science as a form of identification and access control. Biometric recognitions include both physiological and behavioral characteristics. The former is related to the shape of the body, including fingerprint, veins, face, palm print, DNA, hand geometry, iris, retina, EEG, heart beat and odour/scent. The latter is related to the pattern of behavior of a person, including typing rhythm, gait, and voice.
The recognition rates of the biometric identifiers are usually decreased in the different environments using the heuristic approaches but they can be improved through deep learning.
The goal of this special issue is to bring together a diverse but complementary set of contributions on emerging deep learning methods for problems in biometrics. The topics of this special issue include but not limit to the following:
- Big data and large scale methods for biometrics
- Biometrics in fingerprint, veins, face, DNA, palm print, hand geometry, iris, retina, EEG, heart beat and odour/scent
- Biometrics in typing rhythm, gait, and voice
- Biometrics in medical applications
Prof. Shi-Jinn Horng
Guest Editor
Manuscript Submission Information
Manuscripts should be submitted online at www.mdpi.com by registering and logging in to this website. Once you are registered, click here to go to the submission form. Manuscripts can be submitted until the deadline. All submissions that pass pre-check are peer-reviewed. Accepted papers will be published continuously in the journal (as soon as accepted) and will be listed together on the special issue website. Research articles, review articles as well as short communications are invited. For planned papers, a title and short abstract (about 100 words) can be sent to the Editorial Office for announcement on this website.
Submitted manuscripts should not have been published previously, nor be under consideration for publication elsewhere (except conference proceedings papers). All manuscripts are thoroughly refereed through a single-blind peer-review process. A guide for authors and other relevant information for submission of manuscripts is available on the Instructions for Authors page. Applied Sciences is an international peer-reviewed open access semimonthly journal published by MDPI.
Please visit the Instructions for Authors page before submitting a manuscript. The Article Processing Charge (APC) for publication in this open access journal is 2400 CHF (Swiss Francs). Submitted papers should be well formatted and use good English. Authors may use MDPI's English editing service prior to publication or during author revisions.
Keywords
- Deep learning
- Biometric recognitions
- Biometric applications
Benefits of Publishing in a Special Issue
- Ease of navigation: Grouping papers by topic helps scholars navigate broad scope journals more efficiently.
- Greater discoverability: Special Issues support the reach and impact of scientific research. Articles in Special Issues are more discoverable and cited more frequently.
- Expansion of research network: Special Issues facilitate connections among authors, fostering scientific collaborations.
- External promotion: Articles in Special Issues are often promoted through the journal's social media, increasing their visibility.
- Reprint: MDPI Books provides the opportunity to republish successful Special Issues in book format, both online and in print.
Further information on MDPI's Special Issue policies can be found here.