COVID-19: Medical Internet of Things and Big Data Analytics
A special issue of Big Data and Cognitive Computing (ISSN 2504-2289).
Deadline for manuscript submissions: closed (31 October 2021) | Viewed by 8027
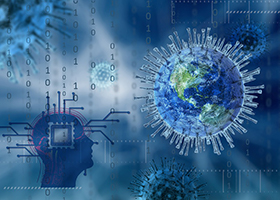
Special Issue Editor
Interests: cognitive computing; machine learning; artificial intelligence; big data; biomedical devices
Special Issues, Collections and Topics in MDPI journals
Special Issue Information
Dear Colleagues,
The coronavirus pandemic, which began in January 2020, was a crisis that affected the entire world medically, socially, and economically. Most of the world was unprepared for such a catastrophe that overwhelmed the healthcare system and forced people to stay in their homes. The pandemic not only highlighted problems and unpreparedness in our current healthcare system, but also created the opportunity for telehealth and data analytics to come to the forefront.
This Special Issue invites high quality papers that discuss COVID-19 and the medical Internet of Things and big data analytics. This can include but is not limited to the topics listed below.
Prof. Dr. Newton Howard
Guest Editor
Manuscript Submission Information
Manuscripts should be submitted online at www.mdpi.com by registering and logging in to this website. Once you are registered, click here to go to the submission form. Manuscripts can be submitted until the deadline. All submissions that pass pre-check are peer-reviewed. Accepted papers will be published continuously in the journal (as soon as accepted) and will be listed together on the special issue website. Research articles, review articles as well as short communications are invited. For planned papers, a title and short abstract (about 100 words) can be sent to the Editorial Office for announcement on this website.
Submitted manuscripts should not have been published previously, nor be under consideration for publication elsewhere (except conference proceedings papers). All manuscripts are thoroughly refereed through a single-blind peer-review process. A guide for authors and other relevant information for submission of manuscripts is available on the Instructions for Authors page. Big Data and Cognitive Computing is an international peer-reviewed open access monthly journal published by MDPI.
Please visit the Instructions for Authors page before submitting a manuscript. The Article Processing Charge (APC) for publication in this open access journal is 1800 CHF (Swiss Francs). Submitted papers should be well formatted and use good English. Authors may use MDPI's English editing service prior to publication or during author revisions.
Keywords
- Telehealth
- Big Data
- Tracking and predicting pandemics and other health crises
- Disease prevention maps
- Homestay data
- Digital surveillance
- DNA
- Genomics
- Flattening the curve COVID modeling
- Healthcare supply chain
- E-health
- M-health
- Telemedicine
- Personalized medicine
- Machine learning for testing
- Machine learning for diagnostics
- Machine learning
- Impact on internet of things
- Impact of COVID-19 on cybersecurity and hacking
- Impact on zoom and other conference platforms