Topic Menu
► Topic MenuTopic Editors
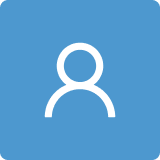
Deep Supplement Learning for Healthcare and Biomedical Applications
Topic Information
Dear Colleagues,
During the challenging years of the ongoing COVID-19 pandemic, the need for efficient artificial intelligence models, tools, and applications in healthcare has been more evident than ever. Deep learning algorithms, supplemented by advanced learning techniques, have the potential to revolutionize healthcare and biomedical technologies. This topic aims to bring together interdisciplinary approaches, focusing on innovative applications and existing AI methodologies to address pressing challenges in the healthcare sector. The topics of interest include deep learning algorithms in healthcare, supplemental learning techniques, biomedical data analysis, and medical image processing. These areas are crucial for interpreting genomic data, developing predictive models, and creating health monitoring systems. AI-driven drug discovery, personalized medicine, and the integration of deep learning with electronic health records (EHRs) are also key focal points. Moreover, this topic will explore the development of clinical decision support systems, healthcare diagnostics, and AI-driven diagnosis in healthcare. The role of AI in health science education, rehabilitation, assistive technologies, public health, and ethical and legal considerations in AI healthcare applications will also be addressed. By highlighting the latest research, innovative approaches, and practical implementations, this topic aims to improve patient outcomes, enhance diagnostic accuracy, and optimize treatment protocols. Researchers are encouraged to develop new or adapt existing AI models, tools, and applications to effectively solve the dynamic and heterogeneous nature of healthcare data problems. In addition to the open call for papers, extended versions of articles presented at relevant conferences are invited. Each submission should contain at least 50% new material, such as technical extensions, more in-depth evaluations, or additional use cases, to contribute significantly to the scientific literature in this field.
Prof. Dr. Tahir Cetin Akinci
Prof. Dr. Ömer Faruk Ertuğrul
Topic Editors
Keywords
- deep learning algorithms in healthcare and supplemental learning techniques
- medical image processing and biomedical data analysis
- cognitive systems
- genomic data interpretation
- predictive modeling in healthcare
- health monitoring systems and clinical decision support systems
- AI-driven drug discovery
- AI and its applications in medicine
- integration of deep learning with electronic health records (EHRs)
- healthcare diagnostics and personalized medicine
- AI-driven diagnosis in healthcare
- AI in health science education
- rehabilitation and assistive technologies
- AI in public health
- ethical and legal considerations in AI healthcare applications
Participating Journals
Journal Name | Impact Factor | CiteScore | Launched Year | First Decision (median) | APC | |
---|---|---|---|---|---|---|
![]()
AI
|
3.1 | 7.2 | 2020 | 18.9 Days | CHF 1600 | Submit |
![]()
Applied Sciences
|
2.5 | 5.3 | 2011 | 18.4 Days | CHF 2400 | Submit |
![]()
Electronics
|
2.6 | 5.3 | 2012 | 16.4 Days | CHF 2400 | Submit |
![]()
Machine Learning and Knowledge Extraction
|
4.0 | 6.3 | 2019 | 20.8 Days | CHF 1800 | Submit |
Preprints.org is a multidisciplinary platform offering a preprint service designed to facilitate the early sharing of your research. It supports and empowers your research journey from the very beginning.
MDPI Topics is collaborating with Preprints.org and has established a direct connection between MDPI journals and the platform. Authors are encouraged to take advantage of this opportunity by posting their preprints at Preprints.org prior to publication:
- Share your research immediately: disseminate your ideas prior to publication and establish priority for your work.
- Safeguard your intellectual contribution: Protect your ideas with a time-stamped preprint that serves as proof of your research timeline.
- Boost visibility and impact: Increase the reach and influence of your research by making it accessible to a global audience.
- Gain early feedback: Receive valuable input and insights from peers before submitting to a journal.
- Ensure broad indexing: Web of Science (Preprint Citation Index), Google Scholar, Crossref, SHARE, PrePubMed, Scilit and Europe PMC.