Topic Editors


Mathematical Applications and Computational Intelligence in Medicine and Biology
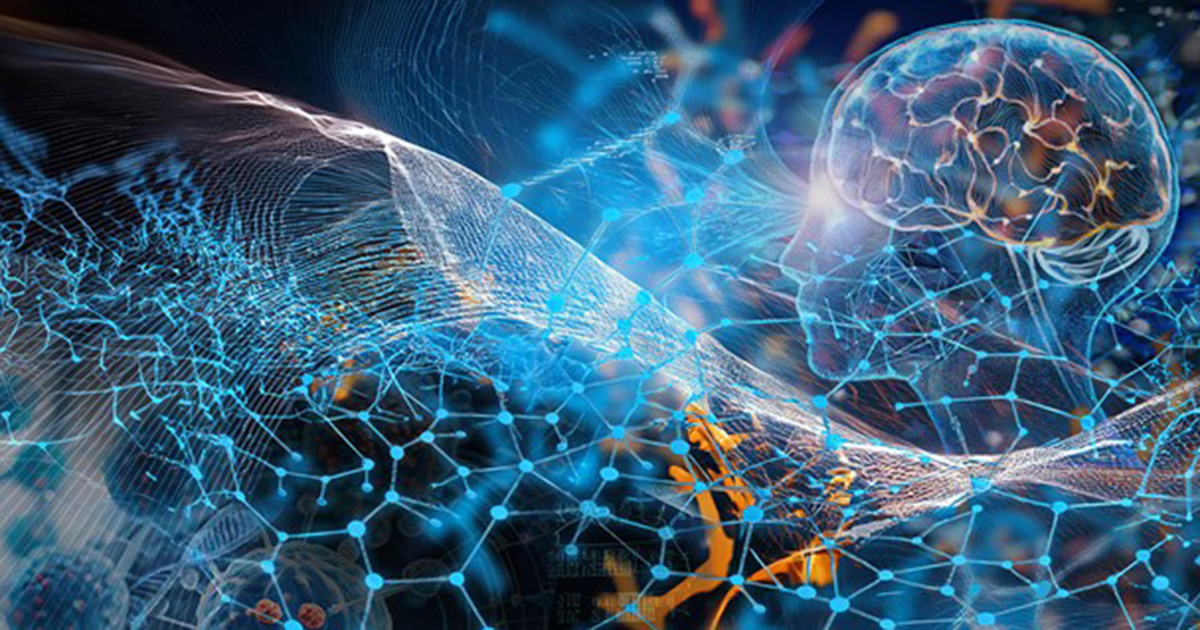
Topic Information
Dear Colleagues,
Mathematical modeling, triaged in an optimized and controlled manner through applicable computational methods with regard to uncertain phenomena, is pertinent for describing and computing complex behaviors, which entails the viable mathematical formulations and applications of neural dynamics across the temporal and spatial scales in medical and biological systems. Systems based on computational intelligence are capable of learning data, manifesting an evolutionary process based on the alterations in the environment while considering uncertainty, complexities and risks and ensuring the characterization of clinical, medical, synthetic, fragmented, omics, imaging, signal, sensor data, amongst other elements to improve the processing of a multitude of data in healthcare toward the development of timely and intelligent solutions to diagnose, prognose, treat, manage and analyze the course of complex dynamic diseases. Accordingly, computational analyses based on advanced mathematical models and computational intelligence, as biologically inspired computational algorithms, constituting the use of genetic algorithms, fuzzy systems, neural networks, probabilistic methods, and so forth, address the employment of computational techniques, methods and algorithms geared towards voluminous data analysis, control, as well as problem-solving in various realms spanning and progressing across advancing applied sciences, engineering applications and scientific research in which mathematical and computational analyses include computational models, simulations, predictions, numerical analyses, processes, etc.
The theory of mathematics and computational theory constitute the essential foundational structure for comprehending computation and its applications in medicine and biology along with their inherent intricate details to analyze the capabilities concerning computer systems, neural networks and algorithms, with relevant implications ranging across various disciplines including mathematics, natural sciences, life sciences, applied sciences, data science, computer science, engineering, bioengineering, etc.
Based on these considerations, mathematical applications and computational intelligence entail the techniques, algorithms, approaches and methods for customized problems and solutions so that mathematical and computational power can be enhanced to process data and conduct advanced implementations regarding real-world complex problems, diseases, biological, epidemiological, environmental factors and other conceivable elements by means of simulations, experimental and/or computational processes, numerical methods, optimization, control and so forth.
Potential topics include, but are not limited to, the following:
- Stochastic disease dynamics of complex networks
- Neural network complexity
- Computational intelligence in oncology
- Complex feedback circuitry
- Gene regulatory networks
- Advanced biomedical data analysis
- Predictive mathematical modeling in medicine/biology
- Medical / Clinical decision-support systems
- Systems biology and medicine
- Precision medicine
- Applied mathematics modeling in treatment regimens
- Soft computing with advanced applications for clinical purposes
- Fractional calculus and its applications in systems medicine and biology
- Difference and differential equations (PDEs, ODEs, FDEs, SDEs, and so on) with applications for diagnostics
- Multidrug-resistant treatment regiments
- Advanced mathematical methods in intelligence systems
- Mathematical modeling, control systems and optimization methods
- Chaos and nonlinear systems
- Fractal physiology and chaos in medicine/biology
- Image and/or signal processing
- Applied complexity, computational complexity and complex systems
- Applied statistics and probability
- Advances in computational modeling in engineering and science applications
- Application of advanced AI (machine learning, deep learning, quantum, and so forth)
- Artificial Intelligence of Things (AIoT) in medicine/biology
Prof. Dr. Yeliz Karaca
Prof. Dr. Majaz Moonis (MD)
Prof. Dr. Yudong Zhang
Topic Editors
Keywords
- intelligence dynamics
- applied analyses and computational methods
- stochastic processes, analyses and models
- computational stochastics
- applied mathematics
- medical imaging and analysis
- data-driven approaches
- computational complexity
- artificial intelligence
- machine learning and deep learning
Participating Journals
Journal Name | Impact Factor | CiteScore | Launched Year | First Decision (median) | APC | |
---|---|---|---|---|---|---|
![]()
Algorithms
|
1.8 | 4.1 | 2008 | 18.9 Days | CHF 1600 | Submit |
![]()
AppliedMath
|
- | - | 2021 | 25.3 Days | CHF 1000 | Submit |
![]()
Mathematics
|
2.3 | 4.0 | 2013 | 18.3 Days | CHF 2600 | Submit |
![]()
Bioengineering
|
3.8 | 4.0 | 2014 | 16.4 Days | CHF 2700 | Submit |
![]()
AI
|
3.1 | 7.2 | 2020 | 18.9 Days | CHF 1600 | Submit |
Preprints.org is a multidisciplinary platform offering a preprint service designed to facilitate the early sharing of your research. It supports and empowers your research journey from the very beginning.
MDPI Topics is collaborating with Preprints.org and has established a direct connection between MDPI journals and the platform. Authors are encouraged to take advantage of this opportunity by posting their preprints at Preprints.org prior to publication:
- Share your research immediately: disseminate your ideas prior to publication and establish priority for your work.
- Safeguard your intellectual contribution: Protect your ideas with a time-stamped preprint that serves as proof of your research timeline.
- Boost visibility and impact: Increase the reach and influence of your research by making it accessible to a global audience.
- Gain early feedback: Receive valuable input and insights from peers before submitting to a journal.
- Ensure broad indexing: Web of Science (Preprint Citation Index), Google Scholar, Crossref, SHARE, PrePubMed, Scilit and Europe PMC.