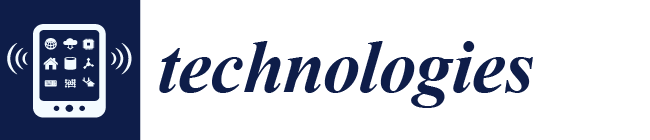
Journal Menu
► ▼ Journal Menu-
- Technologies Home
- Aims & Scope
- Editorial Board
- Reviewer Board
- Topical Advisory Panel
- Instructions for Authors
- Special Issues
- Topics
- Sections & Collections
- Article Processing Charge
- Indexing & Archiving
- Editor’s Choice Articles
- Most Cited & Viewed
- Journal Statistics
- Journal History
- Journal Awards
- Conferences
- Editorial Office
Journal Browser
► ▼ Journal BrowserNeed Help?
Announcements
27 September 2022
Technologies | Collection of Highly Cited Papers Ⅰ
1. “A Survey on Contrastive Self-Supervised Learning”
by Jaiswal, A.; Babu, A. R.; Zadeh, M. Z.; Banerjee, D. and Makedon, F.
Technologies 2021, 9(1), 2; https://doi.org/10.3390/technologies9010002
Available online: https://www.mdpi.com/2227-7080/9/1/2
Highlights:
- Exploration and detailed analysis of the existing state-of-the-art techniques in contrastive learning;
- Extensive evaluation of contrastive learning in various domains such as computer vision (Images, Videos), Natural Language Processing, etc., with state-of-the-art results in multiple datasets;
- Future direction of contrastive learning.
2. “Unsupervised Domain Adaptation in Semantic Segmentation: A Review”
by Toldo, M.; Maracani, A.; Michieli, U. and Zanuttigh, P.
Technologies 2020, 8(2), 35; https://doi.org/10.3390/technologies8020035
Available online: https://www.mdpi.com/2227-7080/8/2/35
Highlights:
- Gives a comprehensive overview of recent advancements in Unsupervised Domain Adaptation of deep networks for Semantic Segmentation;
- Identifies 3 main representation levels at which domain adaptation can be applied, i.e., at the input, at the intermediate feature representation or at the output of the deep network;
- Categorizes the vast range of UDA techniques into 7 groups: Domain Adversarial Learning, Generative-based Adaptation, Classifier Discrepancy, Self-Training, Entropy Minimization, Curriculum Learning and Multi-Task Learning;
- Presents the widely used case study of synthetic-to-real adaptation for the semantic understanding of road scenes;
- Provides an extensive comparison of state-of-the-art approaches on multiple benchmarks and with different segmentation models.
3. “The Road to Improved Fiber-Reinforced 3D Printing Technology”
by Kabir, S. M. F.; Mathur, K. and Seyam, A.-F. M.
Technologies 2020, 8(4), 51; https://doi.org/10.3390/technologies8040051
Available online: https://www.mdpi.com/2227-7080/8/4/51
Highlights:
- Evaluates scopes and capabilities of commercial 3D printing technology to develop fiber-reinforced composites from material (filaments) and technology (slicer) perspectives;
- Unveils detailed properties of commercial 3D printing filaments (fiber and polymer) used for printing fiber-reinforced composites;
- Demonstrates and illustrates the routes to avail high performance printed composites as well as potential avenues of improvement of the printing technology.
4. “Hardware Implementation of a Softmax-Like Function for Deep Learning”
by Kouretas, I. and Paliouras, V.
Technologies 2020, 8(3), 46; https://doi.org/10.3390/technologies8030046
Available online: https://www.mdpi.com/2227-7080/8/3/46
Highlights:
- Proposes a simplified architecture for a softmax-like function, the hardware implementation of which is based on a proposed approximation that exploits the statistical structure of the vectors processed by the softmax layers in various CNNs;
- The proposed architecture is parametrized taking into account the requirements of the targeted application;
- The proposed architecture is efficiently implemented in hardware.
5. “A Parametric EIT System Spice Simulation with Phantom Equivalent Circuits”
by Dimas, C.; Uzunoglu, N. and Sotiriadis, P. P.
Technologies 2020, 8(1), 13; https://doi.org/10.3390/technologies8010013
Available online: https://www.mdpi.com/2227-7080/8/1/13
Highlights:
- Parametric Simulation interface for an Electrical Impedance Tomography Hardware System;
- SPICE and MATLAB software are utilized to simulate the system's analog and digital parts;
- The Phantom Subject Under Test (SUT) is simulated as a frequency-dependent multiport RLC circuitry;
- Sources of measurement errors are examined.