Advancing Action Recognition: Novel Approaches, Techniques and Applications
A special issue of Journal of Imaging (ISSN 2313-433X).
Deadline for manuscript submissions: 31 July 2025 | Viewed by 5835
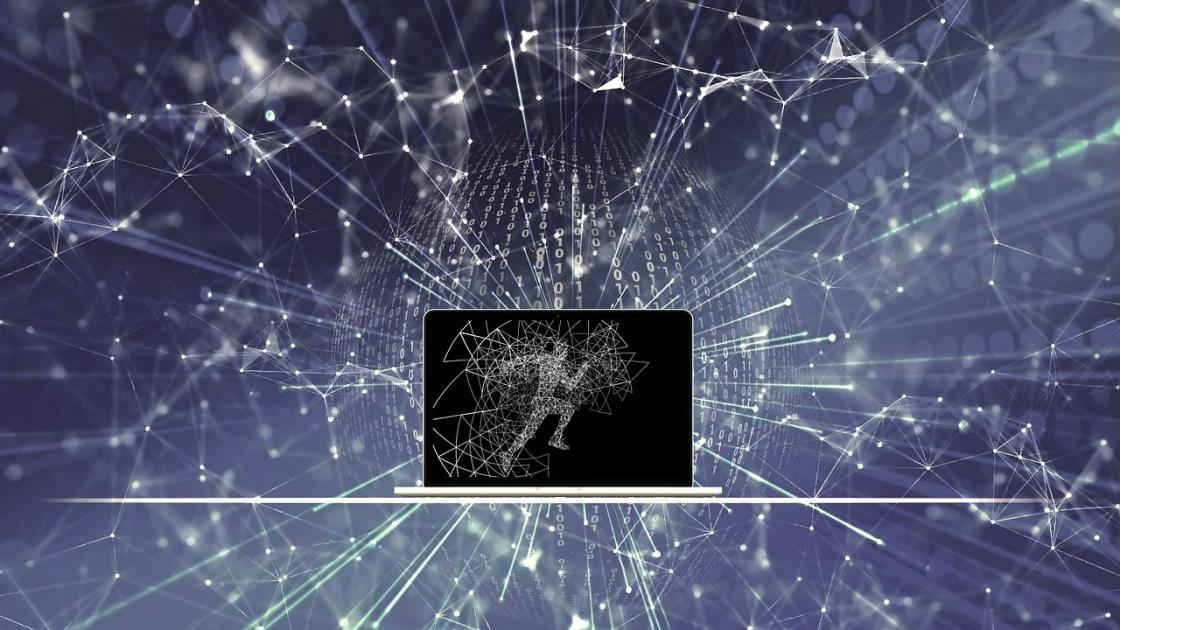
Special Issue Editor
Special Issue Information
Dear Colleagues,
"Advancing Action Recognition: Novel Approaches, Techniques and Applications" aims to explore cutting-edge research in the field of action recognition, focusing on innovative approaches, techniques, and their practical applications. Action recognition, a fundamental task in computer vision and artificial intelligence, holds immense significance across various domains, from surveillance and security to healthcare, robotics, and human–computer interaction. In this era of rapid technological advancement, the quest for innovative methodologies, techniques, and applications in action recognition has never been more critical.
This Special Issue seeks to showcase recent advancements in machine learning, computer vision, and related areas, with a particular emphasis on novel methodologies for detecting and understanding human actions in various contexts. Topics of interest include but are not limited to deep learning models, spatiotemporal modeling techniques, multimodal fusion techniques, transfer learning methods, and model generalization methods. Moreover, the action recognition techniques enhanced by large language models are also a heating topic for discussion.
Furthermore, the Special Issue seeks to highlight emerging applications and novel contexts where action recognition technology can make a transformative impact. Whether it is enhancing security measures through intelligent surveillance systems, revolutionizing healthcare with gesture-based interfaces and patient monitoring solutions, or empowering robots with the ability to understand and interact with human actions, the potential applications of action recognition are vast and far-reaching. By bringing together researchers and practitioners, this Special Issue aims to foster collaboration and drive forward the state of the art in action recognition technology.
Dr. Xiaoguang Zhu
Guest Editor
Manuscript Submission Information
Manuscripts should be submitted online at www.mdpi.com by registering and logging in to this website. Once you are registered, click here to go to the submission form. Manuscripts can be submitted until the deadline. All submissions that pass pre-check are peer-reviewed. Accepted papers will be published continuously in the journal (as soon as accepted) and will be listed together on the special issue website. Research articles, review articles as well as short communications are invited. For planned papers, a title and short abstract (about 100 words) can be sent to the Editorial Office for announcement on this website.
Submitted manuscripts should not have been published previously, nor be under consideration for publication elsewhere (except conference proceedings papers). All manuscripts are thoroughly refereed through a single-blind peer-review process. A guide for authors and other relevant information for submission of manuscripts is available on the Instructions for Authors page. Journal of Imaging is an international peer-reviewed open access monthly journal published by MDPI.
Please visit the Instructions for Authors page before submitting a manuscript. The Article Processing Charge (APC) for publication in this open access journal is 1800 CHF (Swiss Francs). Submitted papers should be well formatted and use good English. Authors may use MDPI's English editing service prior to publication or during author revisions.
Keywords
- action recognition
- multi-modal fusion
- anomaly detection
- domain adaptation/generalization
- computer vision
- video assessment
- robotics
- video understanding
Benefits of Publishing in a Special Issue
- Ease of navigation: Grouping papers by topic helps scholars navigate broad scope journals more efficiently.
- Greater discoverability: Special Issues support the reach and impact of scientific research. Articles in Special Issues are more discoverable and cited more frequently.
- Expansion of research network: Special Issues facilitate connections among authors, fostering scientific collaborations.
- External promotion: Articles in Special Issues are often promoted through the journal's social media, increasing their visibility.
- Reprint: MDPI Books provides the opportunity to republish successful Special Issues in book format, both online and in print.
Further information on MDPI's Special Issue policies can be found here.