Advances in Generating Real-World Evidence from Real-World Data Using Artificial Intelligence
A special issue of Healthcare (ISSN 2227-9032). This special issue belongs to the section "Artificial Intelligence in Medicine".
Deadline for manuscript submissions: 28 December 2025 | Viewed by 4836
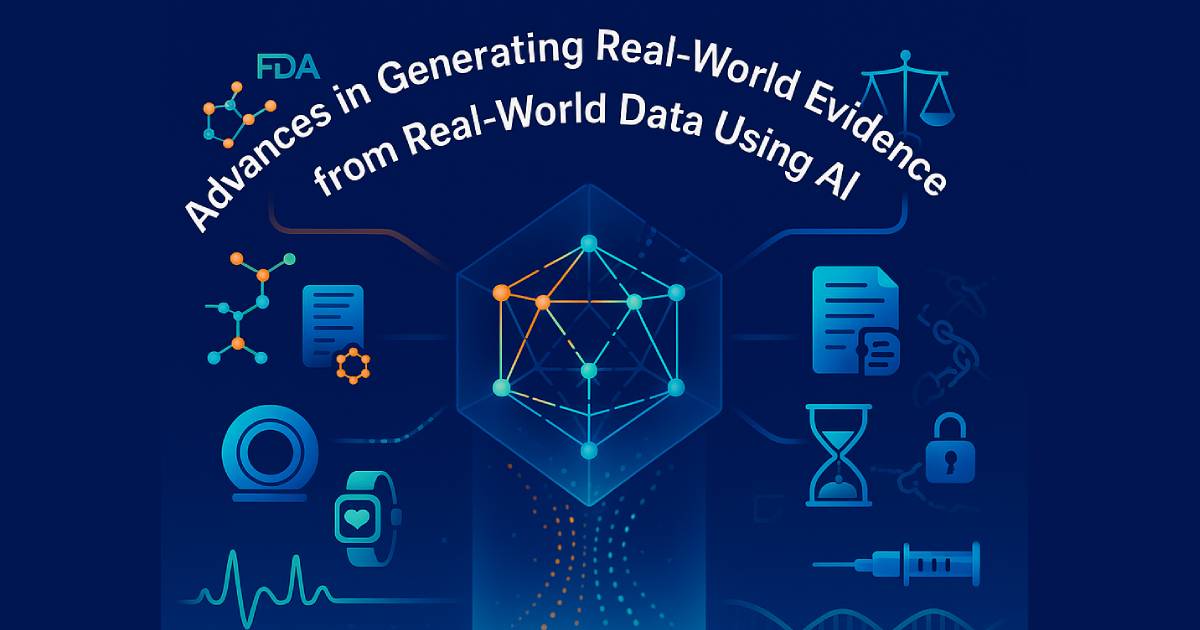
Special Issue Editor
Interests: intelligent data aggregation; predictive analytics; the conduct of clinical trials; machine learning
Special Issues, Collections and Topics in MDPI journals
Special Issue Information
Dear Colleagues,
Real-world evidence (RWE) consists of evidence on patient care and health outcomes that has been developed from real-world data (RWD) generated in routine clinical settings. Its uses span clinical and regulatory decision-making, health technology assessment, clinical trial design, assessing the burden of illness, evaluating market potential for new products, etc. The wide usage of the internet, social media, wearable sensors, mobile devices, electronic billing, disease and product registries, electronic health records and other technology-driven services, together with increased capacity in data storage, have led to the rapid generation and availability of vast amounts of RWD. The increasing accessibility of RWD and the fast development of artificial intelligence (AI) and machine learning (ML) techniques, together with rising costs and the recognized limitations of traditional trials, has spurred great interest in the use of RWD to enhance the efficiency of clinical research and discoveries, and bridge the evidence gap between clinical research and practice. Modern AI approaches have significant potential in generating RWE from complex multimodal data. However, the generation of high-quality RWE using AI faces a spectrum of challenges such as data quality, heterogeneity and completeness, selection bias and generalizability, temporal drifts of longitudinal data, ethical and privacy concerns, regulatory acceptance and validation, and explainability.
This Special Issue will invite original articles, reviews and commentaries representing the best practices and current advances in AI applications for RWE generation, as well as discussions on approaches to address existing challenges.
Prof. Dr. Joseph Finkelstein
Guest Editor
Manuscript Submission Information
Manuscripts should be submitted online at www.mdpi.com by registering and logging in to this website. Once you are registered, click here to go to the submission form. Manuscripts can be submitted until the deadline. All submissions that pass pre-check are peer-reviewed. Accepted papers will be published continuously in the journal (as soon as accepted) and will be listed together on the special issue website. Research articles, review articles as well as short communications are invited. For planned papers, a title and short abstract (about 100 words) can be sent to the Editorial Office for announcement on this website.
Submitted manuscripts should not have been published previously, nor be under consideration for publication elsewhere (except conference proceedings papers). All manuscripts are thoroughly refereed through a single-blind peer-review process. A guide for authors and other relevant information for submission of manuscripts is available on the Instructions for Authors page. Healthcare is an international peer-reviewed open access semimonthly journal published by MDPI.
Please visit the Instructions for Authors page before submitting a manuscript. The Article Processing Charge (APC) for publication in this open access journal is 2700 CHF (Swiss Francs). Submitted papers should be well formatted and use good English. Authors may use MDPI's English editing service prior to publication or during author revisions.
Keywords
- real-world evidence
- artificial intelligence
- healthcare
Benefits of Publishing in a Special Issue
- Ease of navigation: Grouping papers by topic helps scholars navigate broad scope journals more efficiently.
- Greater discoverability: Special Issues support the reach and impact of scientific research. Articles in Special Issues are more discoverable and cited more frequently.
- Expansion of research network: Special Issues facilitate connections among authors, fostering scientific collaborations.
- External promotion: Articles in Special Issues are often promoted through the journal's social media, increasing their visibility.
- Reprint: MDPI Books provides the opportunity to republish successful Special Issues in book format, both online and in print.
Further information on MDPI's Special Issue policies can be found here.