Study on Optimization Design of Airfoil Transonic Buffet with Reinforcement Learning Method
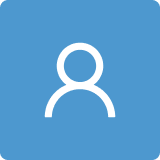
Round 1
Reviewer 1 Report
The reviewer thanks the authors for this interesting work. I am recommending the paper for publication but however after a major revision. The content is very interesting and relevant, but the form these are presented must be improved. In general, the text quality and language use together with the figures quality must be improved. In particular, please address the following points (the index refers to the specific lines:
71: adjoint gradient optimization -> gradient optimization
99: And reduced -> reduced
101: people -> researchers
109: steady state of a vehicle -> steady state
110: simpler and more efficient -> more efficient
113: delete "it is easy to implement"
114: delete "which is an unsteady state of aerodynamic shape optimization, and it is" (redundant)
118: delete "it is a good idea to apply reinforcement learning to transonic buffet optimization" (too colloquial language, and it is to be addressed in the conclusions)
124: DDPC -> using the deep deterministic policy gradient (DDPG) algorithm
124: shape transformation airfoil parametric method -> shape transformation parametric method
127: delete "which"
130: delete "performed by most researchers. It improves the efficient and reliability of the aerodynamic shape optimization design" (redundant)
132: Computing methods -> Numerical setup
134: provide some reference for class shape transformation (CST)
162: a conserved variable -> the vector of conservative variables
In this section, the description of both the spatial discretization and the time discretization for the numerical integration is missing. Please add them.
Please rewrite the paragraph in lines 178-192, the use of language is difficult to follow.
Caption text in Figure 3 can be simplified. Also, add the authors name to the wording "Reference" or "Experiment" in the legend. Harmonize the legend and the plot markers between Fig. 3a and 3b.
For Section 3.1 please provide references to the DDPG algorithm. Also, add a discusion if this is model-based or model-free reinformcement learning. Is the model only used when evaluationg the reward?
218: please specify in detail the parameters used for the implementaion of the RL algorithm. How have the neural networks been set up ? Is there an actor-critic neural network setup ? What kind of environment has been used for the algorithm ? Has it been implemented from scratch ? Please remember that the reader must be able to reproduce the results you are showing.
238: deformed -> written
245: DQN -> deep Q-learning (DQN). Also, provide some reference to DQN.
262: next moment of state -> next state
268: superiority -> with respect to what ?
286: delete ", which has a stronger drag reduction effect" (redundant text)
292: two shock -> two shocks
The caption text of Figure 6 should be improved (redundant wording)
In general, the quality of the figure resolution should be improved.
305: Optimization of Transonic Buffet -> Optimization for Transonic Buffet
In this section 4 some discussion regarding possible side effects of the optimization for flutter / aerodynamic efficiency should be stated, such as possible consequences for loads or stability of the airframe. It is not part of the current work, but it should be clearly stated.
319: the specific research ideas in this paper are as follows -> in this work the following points are considered
Please delete the redundant "in this paper" wording throughout the paper.
349: according to the optimized mathematical model Equation (9), we set the reinforcement learning reward reasonably -> The reinforcement learning reward is set according to Equation (9)
351: how is the most "intense" trasonic buffet the optimized state? Is the reference to be compared with instead ? Please revise the wording
In Sections 4.1 and 4.2 something about the numerical effort of the algorithm compared to others should be added.
Fig. 8a: what is the angle of attack corresponding to this pressure distribution ?
412: advancedness -> advantages
416: most violent -> highest
417: is selected as the buffet optimization state. Please clarify this sentence. How is the most pronounced buffet case the optimized state ?
436: explain the meaning of "flow stability of the airfoil surface"
468: conclusions affecting the flow stability are presented in this paper -> conclusions can be drawn
476: near 0.4 times the chord length. And what is the location for the baseline configuration ?
485: contingent -> specific to these configurations
501: calculated and observed -> computed
534: index second only to the lift-drag ratio -> index to the lift-drag ratio
544: RANS equations -> unsteady RANS equations
575-590: this paragraph is almost identical to the following one, please remove redudant text
The text quality and language use must be improved
Author Response
Please see the attachment.
Author Response File: Author Response.docx
Reviewer 2 Report
Reinforcement learning is applied to airfoil shape optimization considering transonic buffet. Some questions:
What is the buffet boundary for the optimized airfoil in new operating conditions (e.g., Mach number)? Will the buffet boundary be improved for these conditions?
Additional comment is needed for the advantage of reinforcement learning in terms of computational cost, compared to other methods, like global optimization. Also, how many buffet simulations are needed for the entire optimization loop?
Due to the cost of buffet simulation, some related references on reduced-order modeling for buffet (like those based on DMD) can be added and commented on.
English is fine.
Author Response
Please see the attachment
Author Response File: Author Response.docx
Round 2
Reviewer 1 Report
Thank you for addressing all points.
Language use can be improved.