Draw-a-Deep Pattern: Drawing Pattern-Based Smartphone User Authentication Based on Temporal Convolutional Neural Network
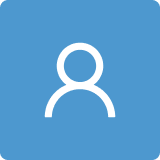
Round 1
Reviewer 1 Report
1. I enjoyed your work.
2. You may add additional explanations to figures 11-13, and the figures do not represent the findings well.
3. Perhaps the general design of networks, such as RNN, is not required.
4. the methodology can be improved
Author Response
Thank you for your comments. Based on your comments, we revised our manuscript as follows.
Comment 2) You may add additional explanations to figures 11-13, and the figures do not represent the findings well.
We provided more explanations on the Figs. 11 and 12 as follows:
This is because the gyroscope in the smartphone that measured the angular velocity is relatively less precise that the touch sensor that captured the coordinate features. Hence, angular-based features were more likely to be affected by the noise when the sequence length increased. (lines 299-302)
We also provided additional explanations on the Fig. 13 as follows:
This independence on the patten length of TCN can be a significant advantage when an authentication algorithm is implemented in an edge device with an insufficient computational capacity. (lines 317-319)
Comment 3) Perhaps the general design of networks, such as RNN, is not required.
Thank you for your comment. We decided to keep this part for those readers who are not familiar with deep learning-based authentication models.
Reviewer 2 Report
Article emphasize on user authentication in smartphones, problem statement is clear and it is well addressed by the proposed methods using DL techniques.
In related work section, authors have not clearly explained the evaluation performance of the proposed methods in their work, current section covers the methods and its limitation. It looks like summary section.
Comparative analysis between TCN and RNN models looks promising.
Experiments are carried out with very limited datasets, if more datasets are included, then it would be good to evaluate the performance of the proposed methods.
Author Response
Article emphasize on user authentication in smartphones, problem statement is clear and it is well addressed by the proposed methods using DL techniques.
In related work section, authors have not clearly explained the evaluation performance of the proposed methods in their work, current section covers the methods and its limitation. It looks like summary section.
Thank you for your comment. Since Related Work usually introduces significant past works related to the proposed method, we did not provide the performance of the proposed model. Instead, we discuss the authentication performance in more detail in Section 5 (Experimental results)
Comparative analysis between TCN and RNN models looks promising.
Experiments are carried out with very limited datasets, if more datasets are included, then it would be good to evaluate the performance of the proposed methods.
Thank you for your comment. Since there was no publicly available dataset on drawing pattern-based authentication in mobile devices, we had to construct the dataset by ourselves. Because of time and budget limit, we recruited 40 participants to collect drawing pattern data in that time. We will extend our research by collecting more data from more participants with different mobile devices.