Performance Assessment of Four Data-Driven Machine Learning Models: A Case to Generate Sentinel-2 Albedo at 10 Meters
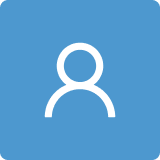
Round 1
Reviewer 1 Report
The reviewer would like to thank the authors for this thoughtful manuscript. This work has good potential. The authors are requested to put in some additional efforts to improve the quality of this manuscript.
Introduction
The authors are requested to elaborate on how such methodology can help in early response of creeping natural disaster related events. Please cite the following article that reports a major disaster and discuss how such an event can benefit from the proposed methodology. The event largely impacted the local human livelihoods as well as manmade infrastructure. How can machine learning techniques help in pre and post disaster management?
-Li et al., “High Mountain Asia Hydropower Systems Threatened by Climate-Driven Landscape Instability”. Nature Geoscience, 2022.
Flowchart
The flowchart provided is difficult to understand. Please increase the size and improve the presentation.
Figures
The authors are requested to provide high-resolution figures of the regression results presented in the paper. The metrics are not clear. Please present the metrics in the bar chart format so they are comparable for different machine learning techniques. Please zoom in the area where the sample cloud is concentrated.
Fig. 12
Model training time is not such an important thing to present. With a lot of preceding power available this is no more an issue.
Mathematical Equations
The authors have not provided equations in the paper. They are requested to include the most relevant ones.
High Resolution Albedo Maps
The authors are requested to provide the high resolution albedo maps. The regression result is not alone sufficient.
Sub-Canopy Snow Cover Mapping
The authors are requested to include this discussion of snow detection under canopy cover and cite the contributions of the following articles that investigated the sub-canopy snow cover with lidar and satellite datasets.
-Gascoin et al., 2019. Theia Snow collection: high-resolution operational snow cover maps from Sentinel-2 and Landsat-8 data, ESSD.
-Kostadinov et al., 2019. “Watershed-scale mapping of fractional snow cover under conifer forest canopy using lidar”, RSE.
The operational High-Resolution Snow & Ice Monitoring (HRSI) algorithm yielded similar performances as the computationally intensive spectral unmixing approach while retrieving the subcanopy ground fractional snow cover. Please highlight the important finding that spectral unmixing is not better than NDSI. Explain how the proposed high resolution albedo will enhance the detection of snow cover even under poorly illuminated conditions.
-Muhuri et al., 2021. Performance Assessment of Optical Satellite-Based Operational Snow Cover Monitoring Algorithms in Forested Landscapes, IEEE JSTARS.
Please highlight that the following investigation mentions contradictory/incorrectly that spectral-unmixing methods can improve snow cover mapping at the global scale. HIghlight here that with the proposed approach the high resolution albedo maps will outdate the spectral-unmixing approach.
-Stillinger et al., 2023. “Landsat, MODIS, and VIIRS Snow Cover Mapping Algorithm Performance as Validated by Airborne Lidar Datasets”, The Cryosphere.
Conclusion
The authors are requested to list the key contributions in this section. At the moment the section is not detailed enough.
Author Response
Dear Editor and reviewers:
Thank you very much for handling our manuscript entitled "(Manuscript ID: Remote sensing-2307300) Performance Assessment of Four Data-driven Machine Learning models: a Case to Generate Sentinel-2 Albedo at 10 Meters ". Both three reviewers raised many insightful comments to help us improve our manuscript's scientific rigor and presentation clarity. Following your and the referees' comments, we have carefully revised and adequately addressed all the concerns that you and the referees have raised.
Several key points regarding our revision are summarized as follows.
First, we rewritten the discussion section over the revised manuscript. The discussion of the consume time to the machine learning models have been deleted for the reason that it is not meaningful. Also, the advantage of the fine-scale albedo has been added in the discussion section by compared the Sentinel-2 albedo with the 500m MCD43A3 albedo to detect the snow-covered surface in Insukati glaciers over Qinghai-Tibet plateau (From Line 483 to 508, in revised manuscript).
Second, we strictly analyzed the scientific points of this manuscript. We believed that out highlight lays in the consideration of topographic effects to generation Sentinel-2 albedo by using machine learning models over mountainous areas and the performance assessment of the machine learning models to the generation of land surface albedo over flat terrain, rugged terrain, and snow-covered surface. To make it clear, we rewritten the last paragraph of the manuscript from 577 to 581.
Third, all of the figures have been redrawn for improving the qualities. The DPI of the figure have been set to 300. Also, a figure has been added in supplementary figure. Lastly, we have clarified the mismatch between figure and text description, corrected spelling mistakes and redundant/overused words, and made many other editing details.
In support of our revision, we attached a point-by-point response to the reviewers' comments. Along with this submission, we also uploaded a 'change tracking' version of the manuscript to facilitate the evaluation process. Thank you very much for your consideration.
Look forward to hearing from you soon.
Yours sincerely,
Xingwen Lin, on behalf of all authors
College of Geography and Environmental Sciences, Zhejiang Normal University, Jinhua, 321004, China
Phone: +86 185-1935-3365; Email: linxw@zjnu.edu.cn
Author Response File: Author Response.docx
Reviewer 2 Report
The authors trained different machine learning models for estimating 10-m albedo from Sentinel-2 MSI data, using surface reflectance-albedo samples created from the RossThick-LiSparseR model, the 3D DART model and in situ observations. While the manuscript is well-written and easy to follow, there are some issues that could be further addressed before publishing. These include:
1) Over flat terrain, samples were derived from the MCD43A1 BRDF data and the RossThick-LiSparseR model, but the authors failed to provide the locations of these samples and how to ensure their representativeness.
2) Line 218 “80% of surface reflectance simulations were used as training datasets and 20% of the simulations were used as testing datasets”, do you split some percent as independent validation datasets?
3) Over rugged terrain, the samples were simulated using DART, I believe DART can also be used to simulate flat terrain, please elaborate more on why DART is not chosen for flat terrain.
4) The references cited in the manuscript lack the names of the journals.
Author Response
Dear Editor and reviewers:
Thank you very much for handling our manuscript entitled "(Manuscript ID: Remote sensing-2307300) Performance Assessment of Four Data-driven Machine Learning models: a Case to Generate Sentinel-2 Albedo at 10 Meters ". Both three reviewers raised many insightful comments to help us improve our manuscript's scientific rigor and presentation clarity. Following your and the referees' comments, we have carefully revised and adequately addressed all the concerns that you and the referees have raised.
Several key points regarding our revision are summarized as follows.
First, we rewritten the discussion section over the revised manuscript. The discussion of the consume time to the machine learning models have been deleted for the reason that it is not meaningful. Also, the advantage of the fine-scale albedo has been added in the discussion section by compared the Sentinel-2 albedo with the 500m MCD43A3 albedo to detect the snow-covered surface in Insukati glaciers over Qinghai-Tibet plateau (From Line 483 to 508, in revised manuscript).
Second, we strictly analyzed the scientific points of this manuscript. We believed that out highlight lays in the consideration of topographic effects to generation Sentinel-2 albedo by using machine learning models over mountainous areas and the performance assessment of the machine learning models to the generation of land surface albedo over flat terrain, rugged terrain, and snow-covered surface. To make it clear, we rewritten the last paragraph of the manuscript from 577 to 581.
Third, all of the figures have been redrawn for improving the qualities. The DPI of the figure have been set to 300. Also, a figure has been added in supplementary figure. Lastly, we have clarified the mismatch between figure and text description, corrected spelling mistakes and redundant/overused words, and made many other editing details.
In support of our revision, we attached a point-by-point response to the reviewers' comments. Along with this submission, we also uploaded a 'change tracking' version of the manuscript to facilitate the evaluation process. Thank you very much for your consideration. Please see the attachment.
Look forward to hearing from you soon.
Yours sincerely,
Xingwen Lin, on behalf of all authors
College of Geography and Environmental Sciences, Zhejiang Normal University, Jinhua, 321004, China
Phone: +86 185-1935-3365; Email: linxw@zjnu.edu.cn
Author Response File: Author Response.docx
Reviewer 3 Report
Land surface albedo is a key parameter in controlling the surface energy budget. This study proposed to compare the machine leaning algorithms and selected the best method to generate fine-scale satellite-based albedo products both over the flat terrain and rugged terrain. The paper is well written, and the technical strength of the work is adequate and appreciated. The experiment results were reported in the Results section of the paper. The premise of the work is interesting and the type of study appears to be suited for the journal. However, several features of the analysis are so unclear as to make assessing the accuracy of the inferences impossible. I suggest the paper can be published in this journal after minor revision.
Overall Comments:
1. In Introduction section,
(1) What’s the main difference between this study and Lin’s work in 2022 (Lin et al., 2022, ISPRS, reference 25 in this study)?
(2) The advantage of the fine-scale albedo needs to be emphasised in this section.
2. In Materials and Methods section,
(1) The using of the in situ observations need to be classified in this section, such as the sites from flat terrain, rugged terrain, snow-covered surface.
(2) Why didn’t use the parameter of relative solar zenith angle during the building of WSA retrieval algorithm?
3. In Discussion section,
(1) The major differences between the four machine learning models need to be added in this section.
In particular, the authors should clarify the following sentences:
1. Line 18, 29, 92, 128. The words “10 meters”, “10 m”, “10-meter” need to be standardized.
2. Line 20, 92. The word “XGBoost” and “XGBT” need to be standardized.
3. Line 61. A reference needs to be added to support this sentence.
4. Line 58-85. The disadvantages of the proposed algorithms need to be addressed.
5. Line 145. The training and testing datasets in Figure 2 were not introduced.
6. Line 152, what’s the meaning of “site surface albedo”?
7. Line 159. Why to use the DART model in this study?
8. Line 186. The symbol “” was not introduced.
9. Line 241-263. Why only used these four machine learning models to generate Sentinel-2 albedo? The advantage of the machine learning models needed to be summarized clearly.
10. Line 279. Remote the redundant explanation ‘testing’ in this sentence.
11. Line 407. It shows that the albedo retrieved by using the XGBT model had the low bias than the RF model. Why you say that the RF models have the better performance than other three models?
Author Response
Dear Editor and reviewers:
Thank you very much for handling our manuscript entitled "(Manuscript ID: Remote sensing-2307300) Performance Assessment of Four Data-driven Machine Learning models: a Case to Generate Sentinel-2 Albedo at 10 Meters ". Both three reviewers raised many insightful comments to help us improve our manuscript's scientific rigor and presentation clarity. Following your and the referees' comments, we have carefully revised and adequately addressed all the concerns that you and the referees have raised.
Several key points regarding our revision are summarized as follows.
First, we rewritten the discussion section over the revised manuscript. The discussion of the consume time to the machine learning models have been deleted for the reason that it is not meaningful. Also, the advantage of the fine-scale albedo has been added in the discussion section by compared the Sentinel-2 albedo with the 500m MCD43A3 albedo to detect the snow-covered surface in Insukati glaciers over Qinghai-Tibet plateau (From Line 483 to 508, in revised manuscript).
Second, we strictly analyzed the scientific points of this manuscript. We believed that out highlight lays in the consideration of topographic effects to generation Sentinel-2 albedo by using machine learning models over mountainous areas and the performance assessment of the machine learning models to the generation of land surface albedo over flat terrain, rugged terrain, and snow-covered surface. To make it clear, we rewritten the last paragraph of the manuscript from 577 to 581.
Third, all of the figures have been redrawn for improving the qualities. The DPI of the figure have been set to 300. Also, a figure has been added in supplementary figure. Lastly, we have clarified the mismatch between figure and text description, corrected spelling mistakes and redundant/overused words, and made many other editing details.
In support of our revision, we attached a point-by-point response to the reviewers' comments. Along with this submission, we also uploaded a 'change tracking' version of the manuscript to facilitate the evaluation process. Thank you very much for your consideration. Please see the attachment.
Look forward to hearing from you soon.
Yours sincerely,
Xingwen Lin, on behalf of all authors
College of Geography and Environmental Sciences, Zhejiang Normal University, Jinhua, 321004, China
Phone: +86 185-1935-3365; Email: linxw@zjnu.edu.cn
Author Response File: Author Response.docx