Inshore Ship Detection in Large-Scale SAR Images Based on Saliency Enhancement and Bhattacharyya-like Distance
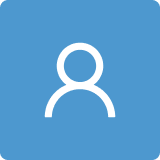
Round 1
Reviewer 1 Report
This paper is well written and shows better of the state of art performance
Author Response
Please see the attachment.
Author Response File: Author Response.pdf
Reviewer 2 Report
The paper is a study on the inshore ship detection in large-scale SAR images based on saliency enhancement and bhattacharyya-like distance and is considered a valuable and interesting study in related fields. The reviewer's opinions are as follows.
1. Abstract should be concisely and clearly described, including the background, purpose, method, result, and conclusion of the study.
2. In the description, ambiguous expressions should be avoided and quantitative numerical values or objective grounds should be presented.
3. It is necessary to describe existing efforts(papers) regarding the problems (not the simple description of the existing studies). The methods that solved the problems perceived in previous similar studies should be described in detail(academic excellence on this paper).
4. In the section describing the simulations and experiments, the composition of the simulations should be clearly explained. It should be described in such a way that readers who related the fields can understand processes in detail. In other words, it should be possible to solve the questions by the composition of the provided experimental environmets including the models suggested.
5. In the 'Conclusion' part, it is necessary to describe the limitations of the study and additional studies required in the future. It is recommended to describe the interpretation of the research results in an easy-to-understand manner.
6. Authors should ensure the quality of the paper in its overall descriptive expression and follow the format of the journal. The composition of the paper should comply with the journal template.
Thank you very much.
Author Response
Please see the attachment.
Author Response File: Author Response.pdf
Reviewer 3 Report
- "The experimental results on LS-SSDD-v1.0 dataset indicate that the proposed method outperforms the state-of-the-art methods" This is a too strong statement. The authors try only basic state-of-the-art architectures that weren't optimized for the Ship Detection task.
- I can not see "the high similarity in scattering intensity and texture information between harbor facilities and ships" in fig 1. A. Authors should explain where is the similarity or use another example.
-! The "related works" section doesn't include related works... There are a lot of interesting papers in the ship detection area. There are even some researches where LS-SSDD-v1.0 database is used. The authors should mention this research, compare the accuracies and show the advantages of their approach. Here is a short list:
Xiaowo Xu, Xiaoling Zhang, and Tianwen Zhang. "Lite-yolov5: A Lightweight Deep Learning Detector for On-board Ship Detection In Large-scene Sentinel-1 Sar Images." Remote Sensing, v. 14 ,.4 -. doi: 10.3390/rs14041018
X. Xu, X. Zhang, T. Zhang, J. Shi, S. Wei and J. Li, "On-Board Ship Detection in SAR Images Based on L-YOLO," 2022 IEEE Radar Conference (RadarConf22), 2022, pp. 1-5, doi: 10.1109/RadarConf2248738.2022.9764190.
A Fast and Lightweight Detection Network for Multi-Scale SAR Ship Detection under Complex Backgrounds Yu, Jimin; Zhou, Guangyu; Zhou, Shangbo; Qin, Maowei., Remote Sensing; Basel Том 14, Изд. 1, (2022): 31. DOI:10.3390/rs14010031
etc.
- The authors use ResNet50 as the backbone. It is interesting whether they try other backbones that lighter and faster than ResNet50.
- The authors compare their method with YOLOv3. This is odd as YOLOv5 have been released a long time ago and it is working with multiscale objects much better.
Author Response
Please see the attachment.
Author Response File: Author Response.pdf
Reviewer 4 Report
- The authors present an exciting paper that proposes a novel saliency enhancement algorithm based on an anisotropic pyramid (DoAP) difference. Firstly, the anisotropic pyramid of the SAR image is constructed by a bilateral filter (BF). Then, the differences between the most delicate two scales and the coarsest two scales are used to generate the saliency map, which can enhance ship pixels and suppress the background clutter. The manuscript’s results are reproducible based on the details given in the methods section. The manuscript is well written and should be of great interest to the readers. However, all figures with charts could be more significant (especially in figure 1 and figure 2- misunderstanding), and the text because it could not be read. Also, the conclusion should mention more about their future work.
Author Response
Please see the attachment.
Author Response File: Author Response.pdf
Round 2
Reviewer 3 Report
The authors have done a good work. Revised version can be published.