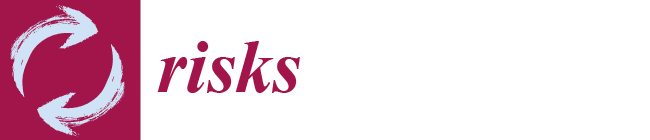
Journal Menu
► ▼ Journal Menu-
- Risks Home
- Aims & Scope
- Editorial Board
- Reviewer Board
- Topical Advisory Panel
- Instructions for Authors
- Special Issues
- Topics
- Topical Collections
- Article Processing Charge
- Indexing & Archiving
- Editor’s Choice Articles
- Most Cited & Viewed
- Journal Statistics
- Journal History
- Journal Awards
- Conferences
- Editorial Office
Journal Browser
► ▼ Journal BrowserNeed Help?
Announcements
5 July 2021
Risks | 2020 Best Paper Awards—Winners Announced
Risks is introducing the Best Paper Award to recognize outstanding papers published in the journal. We are pleased to announce the “Risks Best Paper Award” for 2020. Nominations were chosen from all papers published between 1 January 2019 and 31 December 2019. The Selection Committee examined each paper and expressed their preferences, releasing the rankings of the “Risks 2020 Best Paper Award”.
First Award: “Measuring and Allocating Systemic Risk”
by Markus K. Brunnermeier and Patrick Cheridito
Affiliation: Patrick Cheridito is a professor of mathematics at ETH Zurich and the director of RiskLab Switzerland.
Background: Systemic risk is the possibility that contagion and negative feedback effects could exacerbate a problem originating in parts of a system and, as a result, trigger a collapse of the entire system. Systemic risk was one of the main contributors to the financial crisis of 2007–2008 and the ensuing European debt crisis. As a consequence, new regulatory guidelines have put a special focus on macroprudential regulation and systemically important financial institutions.
Introduction: The paper proposes a practical way of measuring, allocating, and managing systemic risk. The approach measures total systemic risk by quantifying the a priori cost to society for providing tail-risk insurance to the financial system and allocates it to individual financial institutions according to their size-shifted marginal contributions. Different possibilities of implementing macroprudential regulation, such as systemic risk limits, systemic risk charges, and a cap and trade system for systemic risk, are discussed.
Second Award: “Predicting Motor Insurance Claims Using Telematics Data—XGBoost versus Logistic Regression”
by Jessica Pesantez-Narvaez, Montserrat Guillen and Manuela Alcañiz
Affiliation: Riskcenter-IREA, Universtat de Barcelona
Background: Predicting the occurrence of accident claims in motor insurance lies at the heart of premium calculation, but with the development of new artificial intelligence methods, the question of choosing a suitable model has yet to be completely resolved. In this article, the recently proposed methods of XGBoost (Chen and Guestrin, 2016) and logistic regression are considered and compared regarding their predictive performance in a sample of insured drivers, along with their telematic information.
Introduction: When a logistic regression and XGBoost compete to predict the occurrence of accident claims without model-tuning procedures, the predictive performance of the XGBoost (tree booster) was much higher than the logistic regression in the training sample but considerably poorer in the testing sample. Additional efforts have to be taken to tune the XGBoost model to obtain a higher predictive performance without overfitting the data. Based on our results, the classical logistic regression model could predict accident claims using telematics data and provided a straightforward interpretation of the coefficient estimates. Moreover, logistic regression offered a relatively high predictive performance considering that only two coefficients were significant at the 90% confidence level. These results are not bettered by the XGBoost method.
Third Award: “Pricing Options and Computing Implied Volatilities Using Neural Networks”
by Shuaiqiang Liu, Cornelis W. Oosterlee and Sander M. Bohte
Affiliation: Centrum Wiskunde & Informatica (the National Research Institute for Mathematics and Computer Science), Amsterdam, the Netherlands
Background: Mathematical modeling and numerical methods play a key role in the field of quantitative finance, for example, for financial derivative pricing and risk management purposes. Asset models of increasing complexity, like stochastic volatility models, require advanced, efficient numerical techniques to bring them successfully into practice. In the big data era, typical basic computational tasks in the financial industry are becoming computationally intensive due to the large volumes of financial data that are being generated. Recently, deep learning techniques have emerged as powerful numerical approximation techniques to deal with these computation challenges.
Introduction: This paper proposes a data-driven approach, utilizing an artificial neural network, to evaluate financing options and to calculate implied volatilities, in a fast and efficient way. The research work has attracted the attention of students and prominent researchers in computational finance, as well as that of financial institutions. The article published in the journal Risks has been cited many times already, and the relevant presentation has received the Best Presentation Award in the 2nd International Symposium on PDEs & Stochastic Analysis in Mathematical Finance, January 2020.