A Quantitative Multiparametric MRI Analysis Platform for Estimation of Robust Imaging Biomarkers in Clinical Oncology
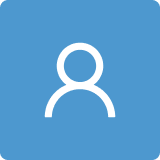
Round 1
Reviewer 1 Report
Comments and Suggestions for AuthorsThis is a very nice work and would be beneficial for the qMRI community.
Comment:
What is the novelty of this platform compared to the previous ones? Could you highlight this point?
Please also discuss the benefits of using your platform against AI-based methods and MR fingerprinting.
Author Response
Please see the attachment
Author Response File: Author Response.pdf
Reviewer 2 Report
Comments and Suggestions for AuthorsThe manuscript describes a comprehensive toolbox for the analysis of
multiparametric MRI to extract oncological biomarkers. The toolbox is
thoroughly benchmarked and discussed in the context of other data
processing tools.
The manuscript is well written and easy to read. Although I do not
have much experience with data processing in the medical space I feel
that the toolbox is well designed, performing above average and
potentially a one-stop shop for the clinician accessing patient MRI
data.
I suggest publication, perhaps after addressing the 2 comments below.
- The arterial input function (AIF) discussed in section 2.1.4 does
not seem to have an equivalent in the workflow model in Fig.2 which
is confusing.
- To a physicist, the Optimal Model Mapping (OMM) is terrifying. It
seems to be based on what works best phenomenologically, not on
first principles. I acknowledge that this may be the only way of
operating, I still like to flag it. Perhaps the limitations of the
approach could be discussed in the manuscript.
Author Response
Please see the attachment
Author Response File: Author Response.pdf
Reviewer 3 Report
Comments and Suggestions for AuthorsThis manuscript gives a useful and important overview of the design, capabilities and merits of the MRI-QAMPER software. MRI-QAMPER is a Matlab toolkit designed to analyse a range of quantitative MRI data; including relaxometry, DCE modelling and diffusion-weighted analyses. The software is both vendor- and organ-agnostic and its efficacy has been tested via a number of challenges. The manuscript is generally well-written and easy to understand. However, there are a few minor corrections and clarifications necessary:
112 remove gap 'CA and tissue'
160 Eq5. The equations for the other models are all in terms of C(t). Either make consistent or briefly explain the reason for the discrepancy.
162 R in R1 should not be subscript.
240 2 in R2 needs superscript.
Fig3 Suggest the use of a linearly perceptual colourmap (see https://www.nature.com/articles/s41467-020-19160-7)
252 DW- MRI remove space
273 Use abbreviation for IVIM
291 Use abbreviation for NCI
332 Madym is a fully-featured multi-model DCE toolkit, so can move to line 334. It can also analyse DWI (ADC, IVIM) and relaxometry (T1 and T2 mapping), similar to MRI-QAMPER. Although the DWI and T2 functionality has yet to be published.
Author Response
Please see the attachment
Author Response File: Author Response.pdf
Reviewer 4 Report
Comments and Suggestions for AuthorsThe paper aims to develop and evaluate a quantitative tool for mpMRI analysis. While it displays clear organization and fluency in language, the absence of related work and a discussion on the current research gap in the introduction is notable. Additionally, the results section lacks quantitative analysis, presenting only a summary without supporting data. Hence, it's difficult to consider this paper as scientifically strong, even though the topic is interesting.
My detailed comments are below:
1. Abstract: You defined some abbreviations, but you didn't define others like DCE, DW, and NCI. To ensure clarity and consistency, please define all abbreviations used in the text.
2. Abstract: Your statement lacks supporting data. For instance, when discussing "MRI-QAMPER routines were validated using digital reference objects (DROs) for DCE-and DW-MRI, serving for approval stages by the NCI/Quantitative Imaging Network (QIN) software benchmark." including specific data or statistics would clarify the validation process and its outcomes. Additionally, please quantitatively define "promising results".
3. Abstract: Could you provide some examples of "wider applications"?
4. Introduction: Please provide a discussion of related work and highlight the current gap in the field to underscore the importance of your developed tool.
5. Introduction: "DRO" was defined twice in the paper.
6. 2.1, Lines 112 & 115: The abbreviation "CA" was introduced and defined after its initial mention.
7. 2.1, Line 115: Figure 2 doesn't have T10. Please include it in the figure.
8. 2. Materials and Methods. Please ensure that all symbols used in equations and text are explicitly defined. For instance, in line 115, "T10" should be defined, in line 120, both "R1(t)" and "ΔR1(t)" should be clarified, and in line 144, "kep" should be defined. Additionally, you showed equation: ∆R1 =(?1− R10) but you actually used ∆R1(t) =(?1(t)− R10). Please be consistent.
9. Results: The results section comprises solely of summary statements and lacks accompanying supportive data. This absence of supporting data significantly weakens the overall substance of the paper. If all the results have been previously published or are intended for future publication, it raises questions about the originality and completeness of this article as an original research contribution.
Author Response
Please see the attachment
Author Response File: Author Response.pdf
Round 2
Reviewer 1 Report
Comments and Suggestions for AuthorsThank you for addressing my comments thoughtfully and now I have no further concerns.
Reviewer 4 Report
Comments and Suggestions for AuthorsI can tell that my questions and comments have been appropriately handled. I want to thank the authors for their hard work in enhancing the paper's quality.