ANN-Based Predictors of ASR Well Recovery Effectiveness in Unconfined Aquifers
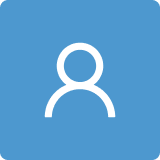
Round 1
Reviewer 1 Report
Comments for author File: Comments.pdf
English writing is difficult to understand because of the very long sentences.
Author Response
Hello Reviewer,
I hope you are doing well.
I attached the revised version of the paper within hydrology-2442663_ANNPaper_Revision_1.docx file.
The responses of your comments are:
---------------------------------------------------------
Comments and Suggestions:
- “English needs to be improved.”
Response 1: We changed words of and shortened 60 sentences in the abstract, body, and conclusions.
- “Introduction needs to be improved, especially for ASR as a tool or man-made interface for interaction of surface water and groundwater, needs more words to stress its importance to manage water resources.”
Response 2: At the beginning of the introduction, we added lines 33-46 to stress the importance of ASR, especially as a tool for integrating surface water and groundwater.
- “ANN as a tool to predict ASR well recovery effectiveness in unconfined aquifers, has many advantages that have been stated in this manuscript. Please add a paragraph to discuss its limitation and short-comings. Especially, the efficiency and usefulness of ANN are determined by not only its structure, but also its training data that must cover the situations/cases that need to be predicted.”
Response 3: We added sentences about limitations of ANN in lines 78-85.
- “The methodology developed in this manuscript seems successful. However, it is based on a hypothetical case with a homogeneous unsaturated aquifer under a simple hydrogeological background. Is there any example can be provided to show how it can be used in real case?”
Response 4: Now, in lines 439-443 we clarify that the ANN is used for reconnaissance evaluation. After the reconnaissance evaluation, if more detailed evaluation is needed for a site having heterogeneous data, one would not use the ANN. Instead, one would calibrate and use a heterogeneous flow and transport model.
- “In MT3DMS modeling, which ion was simulated to use as water quality indicator? And how such a conservative ion was chosen and based on what criteria?”
Response 5: We now mention in lines 254-263 that as commonly done in irrigation water management, chloride can be assumed to be a conservative contaminant. Freshwater, including rainwater, can dissolve halite from soil while recharging or passing through an aquifer. Also, under oxidizing conditions, dissolved nitrate is considered to be nonreactive and conservative (Macpherson and Townsend, 1998). Thus, in MTDMS flow and transport simulations here, the only simulated transport mechanisms are advection and dispersion (Gelhar et. al, 1992).
- “For unsaturated aquifers, how deep is the suitable depth for ASR to avoid evapotranspiration loss of water?”
- “How to assess the water quality for ASR while in wet season surface water may infiltrate into the same aquifer at the same time. This is why ASR is preferred to use saturated aquifer without evaporation loss.”
Responses 6 and 7: We added sentences in lines 203-209. The assumed ground surface elevation of the model study area is a value of 100 m (328.08 ft). That results in: a) zero evapotranspiration loss of water from groundwater. Because capillary fringe does not reach the overlying root zone. Note, the height of the capillary fringe in various soils does not exceed one meter (Health, 1983); and b) zero deep percolation into the same aquifer for this study. Because the unconfined aquifer includes a very thick initial saturated thickness, and deep percolation might cause only a small relative change in transmissivity.
- “In real situation, heterogeneity in aquifers may cause major deviations in predictions from observation. Any consideration for it?”
Response 8: We added sentences in lines 507-510. We believe that the simplicity of the ANN helps water managers to rapidly perform a reconnaissance level evaluation for a candidate ASR well site. At a reconnaissance level there might not be more than one observed hydraulic conductivity or transmissivity value in a candidate injection site.
- “ANN-based methods have a major problem that is also a major strength to attract people to use it. It ignore the physical mechanism that results in the state variable that people want to predict. When we don’t know the mechanism at all or just a little, it is perfect to use ANN. After we have accumulated knowledge and understanding of the system, we should not depend on the ANN too much, but seek physical understanding and also update ANN model based on physical mechanisms.”
Response 9: We added sentences in lines 127-130, 320, 322, 357, and 368 to describe the analytical or logical equations used within Terms 1-3 to estimate plume shape, length, and width for the ASR injection process. The ANN-based predictors assist in estimating the retrieval of injectate, which is not simply the inverse process of the injectate's movement in the down gradient.
Comments on the Quality of English Language:
- “English writing is difficult to understand because of the very long sentences.”
Response 10: We shortened about 60 long sentences to include no more than 22 words.
---------------------------------------------------------
Best regards,
Saeid Masoudiashtiani
Author Response File: Author Response.docx
Reviewer 2 Report
This study provides a rapid method to predict Aquifer Storage and Recovery (ASR) and well recovery effectiveness (REN), using the ANN-based predictors.
The topic is of scientific interest and importance and the manuscript is well presented.
However, this manuscript is recommended for publication if the following issues can be addressed.
General remarks:
- The relevant elements that need to be taken into account to determine the feasibility of an ASR project should be mentioned, such as defining recharge objectives, water demand, availability and storage requirement, hydrogeology, selection of an ASR concept and preliminary design, financial feasibility, environmental feasibility, etc;
- It should be mentioned that ASR implies that the wells are used for both infiltration and withdrawal, and must be referred to as 'dual purpose wells';
- Some general data regarding Applications of ASR, different ASR systems, etc. should be mentioned;
- Some general data regarding design issues and operational issues, etc. should be mentioned.
- Arrangement of equations is necessary;
- The table and Figures in Appendix A must be numbered Table A1, respectively Figures A1 and A2.
- Line 119, renumbered 2.1.1;
Specific remarks:
- There is no definitive formula for determining the optimal number of neurons and hidden layers in an ANN structure, but some details regarding some general guidelines to find the optimal number of neurons and hidden layers in an ANN structure should be mentioned, because it can be challenging and typically involves a combination of experimentation, trial and error, and domain knowledge;
- Regarding the transfer function, why was the Sigmoid function used and not the Rectified Linear Unit function?, the latter being the most popular activation function due to its simplicity and effectiveness and it is computationally efficient and helps alleviate the vanishing gradient problem;
- The architecture of the neural network is improper, it must be entered as input Extraction days, thus more neurons will result on the hidden layer. A neural network must be a model that includes all scenarios. I recommend that the NN input be simpler, so that Term 1, Term 2 and Term 3 be replaced with the data used as input in the programs used, for example MODFLOW2005 and MT3DMS.
Author Response
Hello Reviewer,
I hope you are doing well.
I attached the revised version of the paper within hydrology-2442663_ANNPaper_Revision_1.docx file.
The responses to your comments are:
---------------------------------------------------
Comments and Suggestions:
- “This study provides a rapid method to predict Aquifer Storage and Recovery (ASR) and well recovery effectiveness (REN), using the ANN-based predictors.”
Response: We revised text to make it clear that it is a rapid ‘reconnaissance-level’ method.
General remarks:
- “The relevant elements that need to be taken into account to determine the feasibility of an ASR project should be mentioned, such as defining recharge objectives, water demand, availability and storage requirement, hydrogeology, selection of an ASR concept and preliminary design, financial feasibility, environmental feasibility, etc.;”
Response 1: We added text in lines 16-20, 24-27, 33-46, 141-146, 437-443, 482-486, and 502-510 to define recharge objectives, water demand, availability, and storage requirement, hydrogeology, selection of an ASR concept and preliminary design, and environmental feasibility. Now, manuscript text clarifies that its intent is merely to provide a rapid reconnaissance-level method for predicting injectate recovery (REN), using commonly available hydrogeologic and hydraulic parameters, and assumed surface water availability for injection. Also clarified is that this paper does not provide guidance on: a) determining the minimum acceptable REN for a particular site because that can require evaluating of stochastically varying water availability and rights to use water; and b) evaluating fiscal feasibility because that can further require comparing non-commensurate costs and benefits (requirement to recharge might be legal and cost might be irrelevant).
- “It should be mentioned that ASR implies that the wells are used for both infiltration and withdrawal and must be referred to as 'dual purpose wells';”
Response 2: We added sentences in lines 33-46 to clarify that as one MAR tool, ASR can use both inject water into and extract water from an aquifer using the same well.
- “Some general data regarding Applications of ASR, different ASR systems, etc. should be mentioned;”
- “Some general data regarding design issues and operational issues, etc. should be mentioned.”
Responses 3 and 4: This study uses site hydrogeologic data to develop ANNs that predict REN of one fully penetrating ASR well operating in an unconfined aquifer. We added sentences in lines 33-46 about intended use, and concept of ASR as one of the MAR methods.
- “Arrangement of equations is necessary;”
Response 5: We added sentences in lines 127-130, 320, 322, 357, and 368 to describe the analytical or logical equations used within Terms 1-3 to estimate plume shape, length, and width for the ASR injection process. The ANN-based predictors assist in estimating the retrieval of injectate, which is not simply the inverse process of the injectate's movement in the down gradient.
- “The table and Figures in Appendix A must be numbered Table A1, respectively Figures A1 and A2.”
Response 6: We corrected the numbering of the table and figures relating to such table and figures in the Appendix and body of the paper.
- “Line 119, renumbered 2.1.1;”
Response 7: We corrected the numbering for this part of the paper.
Specific remarks:
- “There is no definitive formula for determining the optimal number of neurons and hidden layers in an ANN structure, but some details regarding some general guidelines to find the optimal number of neurons and hidden layers in an ANN structure should be mentioned, because it can be challenging and typically involves a combination of experimentation, trial and error, and domain knowledge;”
Response 1: We added sentences in lines 78-85, 287-295, 382-389 to explain the employed combination of experimentation, trial and error, and domain knowledge.
- “Regarding the transfer function, why was the Sigmoid function used and not the Rectified Linear Unit function?, the latter being the most popular activation function due to its simplicity and effectiveness and it is computationally efficient and helps alleviate the vanishing gradient problem;”
Response 2: We added sentences in lines 348-351 to explain why we used the Sigmoid function.
- “The architecture of the neural network is improper, it must be entered as input Extraction days, thus more neurons will result on the hidden layer. A neural network must be a model that includes all scenarios. I recommend that the NN input be simpler, so that Term 1, Term 2, and Term 3 be replaced with the data used as input in the programs used, for example, MODFLOW2005 and MT3DMS.”
Response 3: We added sentences in lines 298-300, and 382-389 to explain why we developed Term 1, Term 2, and Term 3.
---------------------------------------------------
Best regards,
Saeid Masoudiashtiani
Author Response File: Author Response.docx