Advanced Image Analysis Methods for Automated Segmentation of Subnuclear Chromatin Domains
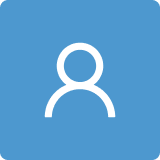
Round 1
Reviewer 1 Report
This manuscript describes two segmentation methods for morphometric analysis of nuclear subdomains. The evaluation of its use by three experimentators gives a nice insight into the possibilities of both methods. The results are very useful and the methods should be available to scientists working in plant nuclear organization, but I guess it can also be to many other scientists interested in analysing 3D-microscopic images. Therefore, this paper should be published. However, several issues can be improved to make this paper easier to read and more accessible to biologists.
General comments
· The introduction is a bit long winded; it would benefit from a stronger focus towards the presented methods.
· What are the motivations to present two different methods? Which one would be most appropriate in which situation
· The methods are applied to 3:1 fixed nuclei. But in formaldehyde-fixed tissue the DAPI stained CCs are often a bit fuzzy. How does the method deal with that?
· It is clear that two users can get different results from manual analysis. But it is not so clear how two users get different results when applying the same iCRAQ automated analysis.
· Explanation of some results (example, fig 4, fig 5) can be improved.
· How does iCRAQ compare to/improve on existing methods developed for a similar type of analysis, like the ImageJ plugin NODeJ (https://pubmed.ncbi.nlm.nih.gov/35668354/)?
· It would be appreciated if the code and data for Nucl.Eye.D was deposited in more suitable repositories (at least GitHub, better Zenodo or similar). That could improve their discoverability and would ensure more permanent accessibility
· Do the presented methods allow for segmentation of individual chromocenters to quantify e.g. their arrangement, relative size, ...?
· Several violin plots are hard to read and show p-values with strange precision
· What other deep learning approaches could be helpful in the future (e.g. contrastive learning, unsupervised methods, ...)? This information could be mentioned in the discussion/conclusion.
· The authors should more discuss about the pros and cons of the two methods. Also discuss a bit more in comparison to the papers by Christoph Tatout and by Philip Andrey.
Specific comments:
· Line 68: Imprecise definition of deep learning. (Deep learning is category of machine learning that characterized by the use of multiple layers of neurons. All machine learning methods aim to estimate model parameters from data, instead of supplying them explicitly.)
· Line 128/Line 646: the link to Nucl.Eye.D is not working, removing the parameters after the questionmarks fixes the problem
· Fig. 4: comparisons between each pair of users are problematic, as they are not fully independent which is an assumption of the applied test. Better would be a measure of inter-rater agreement (https://en.wikipedia.org/wiki/Inter-rater_reliability) that also allows to calculate a summary statistic and an overall comparison between manual/iCRAQ analysis. Perhaps a cohen's kappa test would fit here?
· Fig. 7: similar to Fig. 4
· Line 198: I do not see data supporting this, does the comparison between treatments show a more robust, user independent trend (e.g. first panel fig. 3 user 2)?
· Fig. 6: seems to be missing.
· Line 370: Does this mean that iCRAQ introduces a consistent bias by itself?Line 392: How could these kinds of datasets be obtained? How is inclusion of annotations by multiple users influencing the quality of the trained model?
Author Response
Reviewer 1
- The introduction is a bit long winded; it would benefit from a stronger focus towards the presented methods.
We modified some parts to shorten the introduction and to better focus on both presented methods.
- What are the motivations to present two different methods? Which one would be most appropriate in which situation
We better highlighted the motivation of the 2 presented methods in the introduction and conclusion parts (lines 114-122 and lines 537-539, respectively).
- The methods are applied to 3:1 fixed-nuclei. But in formaldehyde-fixed tissue the DAPI stained CCs are often a bit fuzzy. How does the method deal with that?
The power of Nucl.eye.D is to be (i) ready to use or (ii) flexible and thus de novo trained with different set of tissue samples (such as different fixation methods).
In this manuscript Nucl.eye.D was trained with nuclei prepared from 3:1 (ethanol/acetic acid) fixed tissue originating from a wide range of genotypes with different nuclei and chromocenter shapes.
Our goal was to show that the Nucl.eye.D “ready to use” version can lead to the expected results although the fixation of the dark/light tissue was performed with formaldehyde. This shows that Nucl.eye.D can deal with different fixative method.
- It is clear that two users can get different results from manual analysis. But it is not so clear how two users get different results when applying the same iCRAQ automated analysis.
As mentioned in line 133-139 (initial version of the manuscript): iCRAQ allows “a semi-automatic segmentation”, and “In case of need, iCRAQ includes options for a potential free-hand correction of the segmentation.”, meaning that it includes some manual intervention that explains why there is inter-user variability as compared to fully automated tools. We modified the text accordingly to make it clearer to the readership:
line 133-139: “iCRAQ segmentation is performed via a variable global thresholding of the median-filtered maximum z-projection, and chromocenters through an interactive H-watershed (Figure 1). In case of need, iCRAQ includes options for a potential free-hand correction of the segmentation.”
is replaced by:
line 155-162: “Depending on the image quality, nucleus segmentation is either done automatically using a minimum cross entropy thresholding method [46], or by manual thresholding, or by drawing the nucleus outline with the ImageJ freehand selection tool. Chromocenter segmentation is done via the H-watershed ImageJ with manual intervention to choose the optimal segmentation (Figure 1; [42]). For both nuclei and chromocenters, wrongly detected objects can be individually removed or manually added with the freehand selection tool."
- Explanation of some results (example, fig 4, fig 5) can be improved.
We improved the explanation of the new Fig. 4 displaying the Dice coefficient. In addition, we attempted to be more precise in the conclusions of Fig. 4 at line 222:
“These observations highlight, that cognitive biases occurring during manual segmentation induce high variability. iCRAQ improves object recognition, demonstrating that segmentation assistance can lead to higher accuracy.”
Is replaced by:
“These observations highlight, that cognitive biases occurring during manual segmentation induce high variability and demonstrate that segmentation assistance, using iCRAQ, can improve the reproducibility of object recognition.”
- How does iCRAQ compare to/improve on existing methods developed for a similar type of analysis, like the ImageJ plugin NODeJ (https://pubmed.ncbi.nlm.nih.gov/35668354/)?
As acknowledge by reviewer 1, other methods have been developed for nucleus and chromocenter analysis: NucleusJ, NucleusJ2, NODeJ and a method by Arpón et al. 2018. We agree with the reviewer that those tools were not properly described in the state-of-the-art section and the rationale of designing a new tool (iCRAQ) not sufficiently explained in the text.
iCRAQ heuristic for nucleus detection uses global thresholding of the Z-projected stack and thus is a simplified version of the method used in NucleusJ and Arpón et al. 2018. Concerning chromocenter segmentation, iCRAQ uses the structure tensor which is similar to the NODeJ method and tends to provide smoother estimation of chromocenter boundaries compare to NucleusJ, NucleusJ2 and Arpón et al. 2018.
NucleusJ, NucleusJ2 and NODeJ were designed to provide a fully-automated detection of nuclei and chromocenters. We encountered issues using fully automated tools due to some of our samples’ specificity: etiolated cotyledons present many etioplasts highly fluorescent accolated to the nuclei, nucleus preparation by tissue squashing leads to the presence of debris and vessels, and our semi-automated confocal acquisition mode (specifying acquisition windows in a navigator module) often leads to the presence of multiple nuclei and other objects in the frame. We have then designed iCRAQ to facilitate the manual segmentation of nuclei and chromocenters. iCRAQ only guides, fastens and eases manual segmentation while requiring manual validation or intervention for each nucleus.
Accordingly, we have modified line 53 as follow:
“Open-source software, web-assisted applications and plugins, are increasingly developed and improved to assist or automatize the detection of nuclear substructures through intensity thresholding, edge detection and mathematical image transformation, including several automated tools developed for plant chromatin architecture (NucleusJ, NucleusJ2.0 (Dubos et al., 2020), and NodeJ (Dubos et al., 2020). However, segmentation of high complexity structures exhibiting irregular shapes or intensities remains challenging and often requires time-consuming human curation or decision.
And line 114:
“First, we developed a semi-automated ImageJ macro that we called Interactive Chromocenter Recognition and Quantification (iCRAQ; https://github.com/gschivre/iCRAQ) for the purpose of facilitating nuclei and chromocenter segmentation and reducing inter-user variability while retaining visual validation of each segmented object by the user. iCRAQ relies on simple heuristics to guide the user during nucleus and chromocenter segmentation and accepts user input for manual curation of image segmentation. These validation and curation steps are particularly necessary when contaminants are present (debris, vessels or plastids) that can be otherwise misidentified as nuclei by automated tools.”
- It would be appreciated if the code and data for Nucl.Eye.D was deposited in more suitable repositories (at least GitHub, better Zenodo or similar). That could improve their discoverability and would ensure more permanent accessibility
Nucl.Eye.D code and training data set were deposited in https://doi.org/10.5281/zenodo.7075507
- Do the presented methods allow for segmentation of individual chromocenters to quantify e.g. their arrangement, relative size, ...?
Indeed, each method allows retrieving individual chromocenter measures (i.e relative size). However, given that 2D images were analyzed, both tools do not allow measuring chromocenter arrangement within the nucleus.
- Several violin plots are hard to read and show p-values with strange precision
We homogenized the way to show p-values and displayed violin plots in a more visible manner.
- What other deep learning approaches could be helpful in the future (e.g. contrastive learning, unsupervised methods, ...)? This information could be mentioned in the discussion/conclusion.
We added these points in the conclusion (Line 545):
“In recent years unsupervised learning techniques like contrastive learning improved especially for segmentation of medical images [52,53]. Therefore, these have the benefit of needing much less (semi-supervised training) or no annotated images (self-supervised / unsupervised training) [53]. Consequently, these methods also reduce the risk of inducing a bias through the segmentation method used to build training-set. However, to which extent these more recently developed methods can outperform the established CNN models for the segmentation of nuclei and subnuclear structures remains to be evaluated.”
52 : van Voorst et al.: https://doi.org/10.3174/ajnr.A7582
53 : Wang et al. : https://doi.org/10.1016/j.media.2022.102447
Comment to the reviewer:
These improvements are made often using more complex algorithms on the dataset before the training or using more complex model architectures. The scope of this study was to create an easy-to-understand framework allowing non-specialized biologists to start using deep NN in their workflow. Therefore, we used a relatively basic NN technique of CNN models and easy to visualize model like the U-Net model.
- The authors should more discuss about the pros and cons of the two methods. Also discuss a bit more in comparison to the papers by Christoph Tatout and by Philip Andrey.
For the comparisons with the papers by Christophe Tatout and Philippe Andrey, please refer to the answer to question about NodeJ and modifications in the text as stated in that answer.
Concerning the pros and cons of both methods, as answered to the second question: The central motivation was to test and provide a plug-in assisted methods which can be used to facilitate the production of training data-sets to build a fully automated DL tool. We strongly recommend to use DL based tools to enhance reproducibility (in the case the data-set is large enough we further recommend to retrain the DL tool on your own data, which then will be facilitated by iCRAQ).
Specific comments:
- Line 68: Imprecise definition of deep learning. (Deep learning is category of machine learning that characterized by the use of multiple layers of neurons. All machine learning methods aim to estimate model parameters from data, instead of supplying them explicitly.)
We changed the sentence according reviewer’s suggestion.
- Line 128/Line 646: the link to Nucl.Eye.D is not working, removing the parameters after the question marks fixes the problem
We corrected the link and fixed this problem.
- 4: comparisons between each pair of users are problematic, as they are not fully independent which is an assumption of the applied test. Better would be a measure of inter-rater agreement (https://en.wikipedia.org/wiki/Inter-rater_reliability) that also allows to calculate a summary statistic and an overall comparison between manual/iCRAQ analysis. Perhaps a cohen's kappa test would fit here? Fig. 7: similar to Fig. 4.
Following reviewer’s suggestion comparison was performed using the Dice-coefficient between pairs of users.
- Line 198: I do not see data supporting this, does the comparison between treatments show a more robust, user independent trend (e.g. first panel fig. 3 user 2)?
We convey with the reviewer that we did not sufficiently point to the figure supporting this observation. For example, if user 2 in figure 3 had only measured the dark sample, he/she could not have concluded on a low RHF in darkness as his/her median value is close to the usual 15-20% of heterochromatin content in mesophyll nuclei from light-grown young leaves. Only measuring the wild-type originating from control light condition can he/she conclude about a low heterochromatin content in this condition.
We modified the sentence accordingly:
“In addition, these comparative analyses put emphasis on the fact that measures of heterochromatin organization should not only rely on mean chromocenter number, RHI, HF or RHF, but always need to be expressed as relative to an internal control (i.e. wild-type nuclei originating from control growth condition).”
was replaced by (line 202-207):
“In addition, these comparative analyses put emphasis on the fact that measures of heterochromatin organization should always be expressed as relative to an internal control (i.e. wild-type nuclei originating from control growth condition) as absolute values for the different parameters vary between users while the trends are always conserved (Figure 3).”
- 6: seems to be missing.
We are sorry for the bug in our first submission and carefully checked all figures this time.
- Line 370: Does this mean that iCRAQ introduces a consistent bias by itself? Line 392: How could these kinds of datasets be obtained? How is inclusion of annotations by multiple users influencing the quality of the trained model?
Line 373 was modified due to the use of Dice coefficient rather than Overlap and Mean distance.
A completely bias-free data set does not exist. However, we included images with nuclei exhibiting atypical chromocenter structures in the training set. This allows tracking a wide range of phenotypes such as the one observed for ddm1. Generally speaking, the variability of the training set should be representative of the variability of the test set. (Additionally, the variability of training set is enriched when segmentation is performed by multiple users).
Reviewer 2 Report
Berens et al. create two pipelines for image analysis of plant nuclei and chromocenters: a user friendly, semi-automated program called iCRAQ and a deep learning-based program called Nucl.Eye.D. Image analysis is becoming increasingly important as chromatin biology is further requiring more tools to analyze chromatin dynamics and morphology from multiple angles. The authors did a great job creating these resources, which will help multitudes of plant microscopists in the future. This work should be published if the authors make a few minor changes:
· In lines 310 and 311 the authors say, “Ten_Users_iCRAQ model- 309 based predictions recognized a few more chromocenters in Dark nuclei (Figure 6B) and slightly reduced the nuclear perimeter (Figure 6C and Figure S3).” This is unclear from the data since in figure S3 there is no significant change in nuclear area, and at times the one_user and ten_user iCRAQ data look very similar but the ten_user does not. To make these claims about the ten_user vs one_user vs. ten_user iCRAQ the authors need use a one-way anova with multiple comparisons to add statistical evidence to their claims. If not, then the authors cannot make this claim.
· Figure 7 contains multiple comparisons across conditions in what seems like a single experiment. The test used on these comparisons is the Mann Whitney Wilcoxon test between pairs of conditions, but in this figure the authors are no longer comparing only 2 datasets at a time. Instead, this figure compares all data sets at once to each other. Therefore, the authors should again use a one-way anova to do these comparisons with a post hoc multiple comparison’s tests. This will correct for false positives in the data.
· Data in figure 3 is presented as the measurements per user and trends for each user in the manual and iCRAQ methods for light and dark conditions. Did the authors do the same user vs. user comparison for these data sets as was used in Figure 4 for nucleus mean distance and chromocenter mean distance? If so, they should include this in the paper so that we can make conclusions about user variability in determining heterochromatic faction, intensity, and relative heterochromatic fractions.
Author Response
Reviewer 2
- In lines 310 and 311 the authors say, “Ten_Users_iCRAQ model-based predictions recognized a few more chromocenters in Dark nuclei (Figure 6B) and slightly reduced the nuclear perimeter (Figure 6C and Figure S3).” This is unclear from the data since in figure S3 there is no significant change in nuclear area, and at times the one_user and ten_user iCRAQ data look very similar but the ten_user does not. To make these claims about the ten_user vs one_user vs. ten_user iCRAQ the authors need use a one-way anova with multiple comparisons to add statistical evidence to their claims. If not, then the authors cannot make this claim.
We agree that for nuclear perimeter, there is no significant difference in the nuclear perimeter between the three methods in Figure S3. We modified the sentence accordingly line 325:
“Interestingly, whereas the One_User and Ten_Users models lead to relatively similar results, the Ten_Users_iCRAQ model recognized a few more chromocenters in Dark nuclei (Figure 6B and Figure S3).”
- Figure 7 contains multiple comparisons across conditions in what seems like a single experiment. The test used on these comparisons is the Mann Whitney Wilcoxon test between pairs of conditions, but in this figure the authors are no longer comparing only 2 datasets at a time. Instead, this figure compares all data sets at once to each other. Therefore, the authors should again use a one-way anova to do these comparisons with a post hoc multiple comparison’s test. This will correct for false positives in the data.
Given that different users performed the experiment and did not segment the same number of objects, our data are not strictly paired (and non-parametric). Thus, it does not allow calculation of one-way Anova for example. Consequently, we decided to use the Mann-Whitney U test that likely overestimates p-values.
- Data in figure 3 is presented as the measurements per user and trends for each user in the manual and iCRAQ methods for light and dark conditions. Did the authors do the same user vs. user comparison for these data sets as was used in Figure 4 for nucleus mean distance and chromocenter mean distance? If so, they should include this in the paper so that we can make conclusions about user variability in determining heterochromatic faction, intensity, and relative heterochromatic fractions.
Segmentation performed for Fig. 3 and 4 are the same. Therefore, the DICE coefficient calculation, now displayed in Fig.4, is based on the same segmentation and parameters shown in Fig. 3
Reviewer 3 Report
The manuscript entitled “Advanced image analysis methods for automated segmentation of subnuclear chromatin domains” by Johann to Berens, Schivre, Theune, Peter, Sall, Mutterer, Barneche, Bourbousse and Molinier reports about the development of two image segmentation methods called iCRAQ and Nucl.Eye.D to define chromatin domains in plant nuclei. These new tools are of interest and promise to be useful for the plant epigenetic community. iCRAQ applies classical imaging methods and is provided as an ImageJ macro while Nucl.Eye.D relies on a U-net deep-learning method. Benchmarking was possible for iCRAQ although there are some difficulties to install the dependencies (more details are required to easily install iCRAQ). Unfortunately it was not possible to benchmark Nucl.Eye.D as the colab's link provided by the authors was not functional. The article is well written but sometime suffers from non-relevant considerations and sometimes questionable choices on the results presented in the main section vs supplementary files of the article (see below). I recommend this publication with some modifications.
Main remarks
As a general remark, the authors should better emphasize their results by comparing the methods for their speed, their performance in respect to the expected results and their variability. As an example, S4 illustrates one main result of the manuscript that I suggest to bring back to the main section showing that whatever the threshold taken for the prediction, dark/light results remain significantly different.
L185-204: While a computer will certainly produce the same outcomes for the exact same image, labelling by biologists lead to variability illustrating the difficulty to segment objects and the different choices made by the users. As a well-known example, chromocenters are not always well defined (they are fuzzy domains of chromatin observed by DAPI staining), very small chromocenters can be discarded by the biologist while the machine can perform its segmentation. A more relevant comparison would have been to compare the model results with the expert annotations using classical metrics such as the Dice index or the Hausdorff distance.
A very important point is to made available all methods and datasets through an open source repository. All weblinks should be clearly mentioned within the manuscript.
L109: The colab's link provided by the authors is not functional (thus the jupyter notebook to test Nucl.Eye.D was not available). It would suggest to use a Git repository as for iCRAQ. I strongly recommend to provide the pre-trained Nucl.Eye.D model.
Nucl.Eye.D was not compared to available models such as Cellpose, Stardist or ZeroCostDL4Mic which can also be trained for chromocenter segmentation. At least mentioned these available tools in the introduction.
In the Git repository of iCRAQ, the folder architecture must be respected: macros/iCRAQ/iCRAQ_main.txt
Figure 6 and Figure S1 are missing.
Carefully revised the legend of the Supplementary Figures…
Minor remarks
L57-65: The authors should consider that DL techniques are typically based on manually segmented datasets (training and validation datasets) and then are also prone to follow human biases. DL/ML are useful to reduce inter-operator variability and to automate the resolution of certain imaging problems.
L69: “a DL method… is defined by its ability of learning on its own” replace on its own by “on training datasets”.
L136: The authors should describe in more details the basic principle of iCRAQ and explain what is “a variable global thresholding of the median-filtered maximum z-projection”.
L139-142: the authors should indicate the open repository (web link) where the 50 nuclei are stored
L175-76: Figure2 show close results for the 3 users, can the authors explain what do they mean by “divergent conclusions”
L212: As a non-parametric test is performed (Mann Whitney Wilcoxon test) I recommend to indicate the medians rather the means in Figure 2
L239: I would recommend to mention the repository where the code is accessible (do not list it as a reference).
L250-252: the authors should indicate the open repository (web link) where the two datasets of 150 and 300 nuclei are stored
L280: users annotate 10 images manually and after i-CRAQ but are they the same images?
L290: 12 hours of training for 200 2D images seems to be a lot? Also mentioned in the legend fig 5 l271, 12h for 300 and 150 images. Can the author describe their informatic environment (CPU/GPU, docker, conda…)
L338: The authors cannot claim that they have obtained the expected result with the predictions and that differences in preparation, acquisition, analysis are responsible for the differences. This was not demonstrated. Could it be that the observed results are rather due to the reproducibility of the DAPI staining or to the use of 2D images to estimate area of chromocenters?
L392: the authors should reformulate “(i) the bias-free training set” as a training set is never bias free…
L439: specify the numerical aperture of the objective and image calibration. Are the images oversampled (i.e: 0.06x0.06x0.23)?
L479-484: the authors should indicate the open repository (web link) where Nucl.Eye.D is stored. They should specify the technical limits of this method? (time/image, number of images, CPU vs GPU etc...)
L512 : specify a minimum PC configuration in the text would be convenient for the reader
L530-531 : reformulate as this will fixes the biases leading to a more reproducible result but It cannot avoid them.
Author Response
Reviewer 3
Main remarks
- As a general remark, the authors should better emphasize their results by comparing the methods for their speed, their performance in respect to the expected results and their variability. As an example, S4 illustrates one main result of the manuscript that I suggest to bring back to the main section showing that whatever the threshold taken for the prediction, dark/light results remain significantly different.
We thank the reviewer for this suggestion. We introduced Fig S4 as main figure (Fig. 7).
- L185-204: While a computer will certainly produce the same outcomes for the exact same image, labelling by biologists lead to variability illustrating the difficulty to segment objects and the different choices made by the users. As a well-known example, chromocenters are not always well defined (they are fuzzy domains of chromatin observed by DAPI staining), very small chromocenters can be discarded by the biologist while the machine can perform its segmentation. A more relevant comparison would have been to compare the model results with the expert annotations using classical metrics such as the Dice index or the Hausdorff distance.
We thank the reviewer for this suggestion. We calculated the Dice coefficient for all comparisons and displayed the data in Fig. 8
- A very important point is to made available all methods and datasets through an open source repository. All weblinks should be clearly mentioned within the manuscript.
All images used for the training sets are available. We better highlighted the link to the repository (https://zenodo.org/record/7075507)
- L109: The colab's link provided by the authors is not functional (thus the jupyter notebook to test Nucl.Eye.D was not available). It would suggest to use a Git repository as for iCRAQ. I strongly recommend to provide the pre-trained Nucl.Eye.D model.
We are sorry for the bug in our first submission, we created a unique link to a zenodo repository that host Nucl.Eye.D scripts, training sets, models and macros: https://doi.org/10.5281/zenodo.7075507
- Eye.D was not compared to available models such as Cellpose, Stardist or ZeroCostDL4Mic which can also be trained for chromocenter segmentation. At least mentioned these available tools in the introduction.
As acknowledge by reviewer 3, other methods of segmentations have been developed. We chose to compare our tools to methods specifically developed for nucleus and chromocenter analysis: NucleusJ, NucleusJ2, NODeJ and a method by Arpón et al. 2018. We agree with the reviewer that available tools were not properly described in the state-of-the-art section and the rationale of designing a new tool (iCRAQ) not sufficiently explained in the text.
Accordingly, we have modified line 53 as follow:
“Open-source software, web-assisted applications and plugins, are increasingly developed and improved to assist or automatize the detection of nuclear substructures through intensity thresholding, edge detection and mathematical image transformation, including several automated tools developed for plant chromatin architecture (NucleusJ, NucleusJ2.0 (10.1080/19491034.2020.1845012), and NodeJ (10.1186/s12859-022-04743-6). However, segmentation of high complexity structures exhibiting irregular shapes or intensities remains challenging for samples from peculiar tissues.”
- In the Git repository of iCRAQ, the folder architecture must be respected: macros/iCRAQ/iCRAQ_main.txt
The folder architecture has been modified accordingly on Github.
- Figure 6 and Figure S1 are missing.
We corrected this mistake.
Minor remarks
- L57-65: The authors should consider that DL techniques are typically based on manually segmented datasets (training and validation datasets) and then are also prone to follow human biases. DL/ML are useful to reduce inter-operator variability and to automate the resolution of certain imaging problems.
We added this important point in the introduction (Line 71)
- L69: “a DL method… is defined by its ability of learning on its own” replace on its own by “on training datasets”.
We thank the reviewer to have raised this important point. We corrected the sentence.
- L136: The authors should describe in more details the basic principle of iCRAQ and explain what is “a variable global thresholding of the median-filtered maximum z-projection”.
We agree with the reviewer that this sentence was not clear. It has been modified accordingly as below:
Line 152: “iCRAQ segmentation is performed via a variable global thresholding of the median-filtered maximum z-projection, and chromocenters through an interactive H-watershed (Figure 1). In case of need, iCRAQ includes options for a potential free-hand correction of the segmentation.”
is replaced by:
“Depending on the image quality, nucleus segmentation is either done automatically using a minimum cross entropy thresholding method [44], or by manual thresholding, or by drawing the nucleus outline with the ImageJ freehand selection tool. Chromocenter segmentation is done via the H-watershed ImageJ plugin with manual intervention to choose the optimal segmentation (Figure 1; [40]). For both nuclei and chromocenters, wrongly detected objects can be individually removed or manually added with the freehand selection tool."
44 = Li, C. H., & Tam, P. K. S. (1998). An iterative algorithm for minimum cross entropy thresholding. Pattern Recognition Letters, 19(8), 771–776. doi:10.1016/s0167-8655(98)00057-9
- L139-142: the authors should indicate the open repository (web link) where the 50 nuclei are stored
All images used for the training sets are available. We better highlighted the link to the repository.
https://doi.org/10.5281/zenodo.7075507
- L175-76: Figure2 show close results for the 3 users, can the authors explain what do they mean by “divergent conclusions”
We realized that the term “divergent conclusions” was not appropriate. We removed this term.
- L212: As a non-parametric test is performed (Mann Whitney Wilcoxon test) I recommend to indicate the medians rather the means in Figure 2
We produced new graphs with medians.
- L239: I would recommend to mention the repository where the code is accessible (do not list it as a reference).
We better mentioned the link to the repository, both as reference and in full in the abstract. https://doi.org/10.5281/zenodo.7075507
- L250-252: the authors should indicate the open repository (web link) where the two datasets of 150 and 300 nuclei are stored
All images used for the training sets are available. We better highlighted the link to the repository.
https://doi.org/10.5281/zenodo.7075507
- L280: users annotate 10 images manually and after i-CRAQ but are they the same images?
Indeed, that is correct. We modified the sentence in the methods:
“For the training set #2, 10 different users each segmented 10% of the total set of images. For training set #3, 10 different users segmented 10% of the total set using the iCRAQ tool.”
By
Line 485: “For the training sets #2 and #3, 10 users each segmented 10% of the total set of images either manually (set #2) or using the iCRAQ tool (set #3).”
- L290: 12 hours of training for 200 2D images seems to be a lot? Also mentioned in the legend fig 5 l271, 12h for 300 and 150 images. Can the author describe their informatic environment (CPU/GPU, docker, conda…)
To be more specifics, 12 hours of training considering 192 000 images [Batch size (data-augmented images from training set) * Epochs]
Model 1: 64 * 1000 = 64 000
Model 2: 128 * 500 = 64 000
Model 3: 128 * 500 = 64 000
= 192 000 images
We specified this number in the text and we added the following features in the method section Lone 507:
(Google colab allocated a Cuda v 11.2 ; Tesla P100 - 16 Go HBM2 GPU and Intel(R) Xeon(R) CPU @ 2.20GHz CPU)
- L338: The authors cannot claim that they have obtained the expected result with the predictions and that differences in preparation, acquisition, analyses are responsible for the differences. This was not demonstrated. Could it be that the observed results are rather due to the reproducibility of the DAPI staining or to the use of 2D images to estimate area of chromocenters?
We suggested the possibility of the potential reason why the Nucl.eye.D method displayed a globally lower RHF compared to manual segmentations on identical images. Indeed, the reproducibility of DAPI staining between training set and Dark/light set may be due to “sample preparation” (3:1 ethanol/acetic acid vs formaldehyde).
- L392: the authors should reformulate “(i) the bias-free training set” as a training set is never bias free…
We removed the sentence Line 392.
- L439: specify the numerical aperture of the objective and image calibration. Are the images oversampled (i.e: 0.06x0.06x0.23)?
Features of confocal pictures have been added in the methods section. All our confocal pictures used in the article are 0.05x0.05x0.35um. The objective 63X with a numerical aperture of 1.40 (1.30 for the most recent ones) was used, with a zoom factor of 4.8, acquired in 16bit, 1024x1024.
- L479-484: the authors should indicate the open repository (web link) where Nucl.Eye.D is stored. They should specify the technical limits of this method? (time/image, number of images, CPU vs GPU etc...)
We better highlighted the web link to the Nucl.Eye.D script in the main text: https://doi.org/10.5281/zenodo.7075507
- L512: specify a minimum PC configuration in the text would be convenient for the reader
As Nucl.eye.D is available using external hardware, for example using GoogleColab, we did not specify any minimal PC configuration in the text, however configuration of the CPU and GPU allocated for the training were specified in the method part (Line 506).
- L530-531: reformulate as this will fixes the biases leading to a more reproducible result but It cannot avoid them.
We fully agree with the reviewer on that point but the sentence line 530 does not imply that efforts will avoid bias. We tried to modify it for clarity:
“Additionally, according to our thoughts, the growing trend of automatizing image segmentation and analysis should be accompanied by substantial efforts in verifying the efficiency and inter-user variability of the segmentation method.”
Replaced by:
Line 571: “Additionally, according to our thoughts, the growing trend of automatizing image segmentation and analysis should be accompanied by substantial efforts in assessing inter-user variability of the segmentation method.”
Round 2
Reviewer 1 Report
The authors have adequately replied on the review comments. This paper is ready for publication
Reviewer 3 Report
Dear authors,
Many thanks for having followed our recommendations and for all the editings that have been introduced into the manuscript. These have nicely improved the previous version.
Best wishes.