Tropical Dry Forest Dynamics Explained by Topographic and Anthropogenic Factors: A Case Study in Mexico
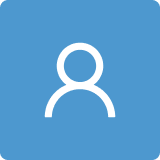
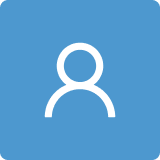
Round 1
Reviewer 1 Report
Tropical dry forests are disappearing rapidly, and this study aims to investigate the dynamics of tropical dry forests and their influencing factors. Machine learning algorithms are mainly used for image classification, and then logistic regression models are used to correlate reasonable factor variables to predict areas of future TDF loss and gain. But there are still some issues that need to be addressed:
(1) Lines 107, 109, 114: Sentinel-2A, DEM, road data and land vegetation and use data can be added to the website link, which is a clearer view of the data set for readers.
(2) Explain how agricultural data can be extracted from land use, land cover and vegetation maps obtained from INEGI?
(3) The author introduces two classification methods, artificial neural networks and random forests, both applying 5-fold cross-validation with 5 repeats, and the author can give a brief introduction to this cross-validation method.
(4) For the sample segmentation ratio mentioned in the two classification algorithms, how did the author determine it?
(5) Can you show the results of the experiment that determines the mtry value?
(6) Give the reason for choosing the best model prediction.
(7) Line 232: An explanation is given for reclassification using 0.5 as a threshold.
(8) Both ANN and RF classification results have high accuracy, why did the authors choose ANN results for analysis?
(9) Line 334: Describe the Wilcox-test.
(10) You can increase the number of study areas or change the classification method to compare experiments again, and finally find that the gain and loss of TDF are balanced.
(11) Whether the area covered by TDF can be selected to compare and analyze the area with large TDF coverage and the area with small TDF coverage, the influencing factors of TDF dynamic change can be obtained.
Author Response
Tropical dry forests are disappearing rapidly, and this study aims to investigate the dynamics of tropical dry forests and their influencing factors. Machine learning algorithms are mainly used for image classification, and then logistic regression models are used to correlate reasonable factor variables to predict areas of future TDF loss and gain. But there are still some issues that need to be addressed:
Thank you. We provided point-to-point response below inside "[ ]".
- Lines 107, 109, 114: Sentinel-2A, DEM, road data and land vegetation and use data can be added to the website link, which is a clearer view of the data set for readers.
[Thank you. We modified table 1 by adding a new column to show the website link for the access of the datasets].
- Explain how agricultural data can be extracted from land use, land cover and vegetation maps obtained from INEGI?
[We added the detail of how we extracted agricultural data from land use land cover and vegetation map of INEGI and how we created the variables of distance to roads, and distance to agriculture, in the lines 117-121].
- The author introduces two classification methods, artificial neural networks and random forests, both applying 5-fold cross-validation with 5 repeats, and the author can give a brief introduction to this cross-validation method.
[Thanks. We provided a brief introduction to the cross-validation method, in lines 152-158].
- For the sample segmentation ratio mentioned in the two classification algorithms, how did the author determine it?
[For the ANN algorithm, the sample segmentation ratio was 0.7, with 70% of the data for training, and 30% of the data for validation. For RF algorithm, the ratio was 0.2, with 20% of the data for training and 80% of the data for validation. The ratio of 0.7 was frequently adopted, while the ratio of 0.2 was determined mainly for practical reasons, since it was the division that the RF algorithm could function. Since we have limited computer power, we used the largest percentage possible of the training data that the computer was capable of processing].
- Can you show the results of the experiment that determines the mtry value?
[We showed the results of the experiment in the Appendix as Table A1 for the mtry in the 2022 classification, and Table A2 for the mtry in the 2019 classification].
- Give the reason for choosing the best model prediction.
[We provided the criterion for choosing the best model prediction, and we modified the text accordingly in lines 242-249].
- Line 232: An explanation is given for reclassification using 0.5 as a threshold.
[We gave the explanation in the text, see lines 265-267].
- Both ANN and RF classification results have high accuracy, why did the authors choose ANN results for analysis?
[We explained this in lines 290-294].
- Line 334: Describe the Wilcox-test.
[We provided the description and reference for the Wilcox-test that it is a non-parametric test that compares the median values of two samples, see lines 273-275].
- You can increase the number of study areas or change the classification method to compare experiments again, and finally find that the gain and loss of TDF are balanced.
[We agree with the observation of the reviewer, and this is also what the confidence intervals of the estimated TDF loss and gain have shown in table 3].
- Whether the area covered by TDF can be selected to compare and analyze the area with large TDF coverage and the area with small TDF coverage, the influencing factors of TDF dynamic change can be obtained.
[We agree with this observation. We also think that by running a set of separate models using data of larger and smaller TDF gain and loss, for example, by using the 25% of either higher or lower percentile of the area distribution of the TDF loss and gain, some interesting results can be found regarding the size and the contributing factors. However, due to the small number of polygons of TDF gain that we have obtained and correctly verified, the 25% of the percentile does not give reliable number of samples for the model. In the future research, we would like to expand the time period of the study, and hopefully can identify a bigger number in TDF change polygons, and therefore can perform this analysis].
Reviewer 2 Report
General comments
The study on land cover dynamics using only 2 images separated by 3 years is not very convincing. For such a study, long-term data covering 10, or more years will be necessary, which will also show the effect of extreme climatic conditions. Climatic information is missing. It is advisable to include some field investigations to verify the obtained results. There is a lot of confusion about the terms used in the land cover classes as well as in the presentation of results.
Detail comments
1. Line 36. Define first what you mean by dry broad-leaved forest. Do you mean that the leaves are dry? Also, explain the process for causing dryness.
2. Lines 89-91. The description of the study area is very scanty. What are the Lat/Long coordinates, the surficial area, geomorphology, topography, etc.?
3. Lines 91-93. How do you prove that your study area is tropical or sub-tropical? Explain with supporting climatic data.
4. Table 1. You have used the Sentinel 2A data obtained on 15 March 2022 and 15 May 2019. How do you prove that there is no seasonal effect on the vegetation cover changes due to 2 months gap between the Sentinel images you have used?
5. Table 2. Some of the land cover classes are very strange e.g. irrigated dry and irrigated wet. If they are irrigated how can they be dry? Also, if crops are harvested why the land is irrigated and so on. It will be better to call them fallow. Also, it will be better to call rain-fed agriculture instead of temporal agriculture.
6. The figure on page 5.
- It is not clear how many samples were taken to represent each land cover class. Are the reflectance curves shown in the figure represent the mean values of the classes? Please explain.
- Some classes show overlap (Temperate forest, irrigated agriculture) in 2022. Is this due to the seasonal effect?
- Figure numbering is wrong.
- Table 3. I
- I suppose that AP(%) is the producer’s accuracy.
- Producer’s accuracy is shown in percentage. What is the unit of user’s accuracy?
- Why there is a big difference between the producer’s and the user’s accuracy? Please explain.
Author Response
The study on land cover dynamics using only 2 images separated by 3 years is not very convincing. For such a study, long-term data covering 10, or more years will be necessary, which will also show the effect of extreme climatic conditions. Climatic information is missing. It is advisable to include some field investigations to verify the obtained results. There is a lot of confusion about the terms used in the land cover classes as well as in the presentation of results.
Thank you. We provide point-to-point response below inside "[ ]".
Detail comments
- Line 36. Define first what you mean by dry broad-leaved forest. Do you mean that the leaves are dry? Also, explain the process for causing dryness.
[Thank you. Tropical dry forest is composed of deciduous and semi-deciduous trees and about 50-80% of the trees lose their leaves during a prolonged dry season. We have now detailed this characteristics in lines 95-97].
- Lines 89-91. The description of the study area is very scanty. What are the Lat/Long coordinates, the surficial area, geomorphology, topography, etc.?
[We agree with this observation. We have added the Lat/Long coordinates in Figure 1 which depicts the study area, and also added the geographic characteristics of the study area, in lines 92-95].
- Lines 91-93. How do you prove that your study area is tropical or sub-tropical? Explain with supporting climatic data.
[We added this information in the description of the study area, mainly in lines 92-95].
- Table 1. You have used the Sentinel 2A data obtained on 15 March 2022 and 15 May 2019. How do you prove that there is no seasonal effect on the vegetation cover changes due to 2 months gap between the Sentinel images you have used?
[Both images are in dry season, during which there is little difference in the spectral reflectance of the land use land cover types. But most important of all is that each image has its own training data (please see the last column of the Table 2, where we included the number of training samples for the classification of 2019 and 2022), and therefore, the classification was not affected by the difference of the 2 months gap].
- Table 2. Some of the land cover classes are very strange e.g. irrigated dry and irrigated wet. If they are irrigated how can they be dry? Also, if crops are harvested why the land is irrigated and so on. It will be better to call them fallow. Also, it will be better to call rain-fed agriculture instead of temporal agriculture.
[Sorry for the confusion and thanks for this comment. We have corrected the names of the land use land cover classes in Table 2, and in Figures A3-A6. ]
- The figure on page 5. - It is not clear how many samples were taken to represent each land cover class. Are the reflectance curves shown in the figure represent the mean values of the classes? Please explain.
[We added the number of training samples (in pixel) for the classification of 2019 and 2022 in Table 2. Yes, the reflectance curves shown in the figure represent the mean values of the reflectance of the classes. We clarified this in the caption of the Figure 2].
- Some classes show overlap (Temperate forest, irrigated agriculture) in 2022. Is this due to the seasonal effect?
[Yes, this is due to the interannual variation in the land covers of the study area].
- Table 3. I suppose that AP(%) is the producer’s accuracy.
[AP(%) represents the area proportion of the classes. We clarified this in the caption of the table].
- Producer’s accuracy is shown in percentage. What is the unit of user’s accuracy?
[Both producer’s accuracy and user’s accuracy is shown in decimal digits; we have clarified this in the table].
- Why there is a big difference between the producer’s and the user’s accuracy? Please explain.
[The big difference between the producer’s accuracy and the user’s accuracy is because of the relatively large commission error in the classes of change, especially in TDF gain. However, since we only used the verified TDF loss and gain and verified TDF persistence to construct the model for exploring the influencing variables, the modelling of the TDF dynamics is robust. We explained this in the section of 2.6].
Reviewer 3 Report
The manuscript discusses an interesting work and is well-presented. However, the following minor points may be noted for further improvement,
1. Authors need to highlight the novelty of the work in comparison with the state-of-the-art
2. It is also suggested to use more algorithms (state-of-the-art) for the analyses so as to avoid any algorithmic bias.
Author Response
The manuscript discusses an interesting work and is well-presented. However, the following minor points may be noted for further improvement,
Thank you. We provide our response below point by point inside "[ ]".
- Authors need to highlight the novelty of the work in comparison with the state-of-the-art
[Thank you. Our study area, the Ayuquila River watershed has a long history in the practice of shifting cultivation. However, it has not been studied that, especially with a spatially explicit method, whether there is a balance in TDF loss and gain in the tropical dry forest under the scheme of shifting cultivation. We have clarified this in lines 78-80].
- It is also suggested to use more algorithms (state-of-the-art) for the analyses so as to avoid any algorithmic bias.
[We agree that it is important to try more algorithms. In the paper, we presented only two algorithms of machine learning, artificial neural network (ANN) and random forest (RF), however, we actually carried out other machine learning algorithms as well such as decision trees, maximum likelihood classifier, and K-nearest neighbor. We presented the ANN and RF since they gave better results].
Reviewer 4 Report
Comments:
1. Author should enhance the quality of all pictures in the document (2) Figure 1. It is not necessary to show the LULCC, it can be put in the result of the study. The study area should only mention the location of which part of Mexico. (3). Table1: please mention the source of data (4.) Figure 1 is not necessary to show. (5). line 158, why author use 500? have you research on that? (6). Table 2. the class of results and with this table is not consistent. It should use the same class as the result and the table. (7). line 162: in the change analysis only use 5 classes. it is also not consistent. (8.) line 201: This subchapter should be more elaborated because this is the key to study and also mention in the title. better if you explain with a flowchart (9.) Line 326: better use table than boxplot (10). Tabel A1. it is also not consistent with the class mentione in the method
Comments for author File: Comments.pdf
Author Response
- Author should enhance the quality of all pictures in the document
[Thank you. We have enhanced the quality of the pictures].
- Figure 1. It is not necessary to show the LULCC, it can be put in the result of the study. The study area should only mention the location of which part of Mexico.
[We updated Figure 1 by including only the location in Mexico. We reallocated the result of land use land cover change and the distribution of the verification points to the section of accuracy assessment as Figure 3].
- Table1: please mention the source of data.
[We added a new column in Table 1 and explained the sources of the datasets].
- line 158, why author use 500? have you research on that?
[We used 500 trees since it is a recommended number in the application Random Forest algorithm in remote sensing. We added a reference for this choice].
- Table 2. the class of results and with this table is not consistent. It should use the same class as the result and the table.
[Thank you. We updated Table 2 and the captions of Figures A3-A6 to make the sure the same classes were used in the table and in the classifications].
- line 162: in the change analysis only use 5 classes. it is also not consistent.
[Sorry for the confusion. We clarified this by making it clear that the change analysis was carried out after reclassifying the land cover classifications into 5 classes. Please see lines 177-184].
- line 201: This subchapter should be more elaborated because this is the key to study and also mention in the title. better if you explain with a flowchart
[Thank you for the observation. We have clarified this subchapter (lines 196-228). We opt for not including a flowchart since we have already many tables and figures in the manuscript].
- Line 326: better use table than boxplot (10).
[Thank you for the suggestion. We kept the boxplot since we believe it can better depict the distribution of the TDF loss and gain in sizes].
- Table A1. it is also not consistent with the class mentioned in the method
[Thank you for identifying this inconsistence. We corrected the captions for the tables A1-A4 in lines 467-470].
Round 2
Reviewer 2 Report
1. Corrections/additions have been carried out based on my detailed comments. However, I would have liked the answers with some supporting climatic data related to my question on 2 months gap between the Sentinel images. You mentioned that both images are in the dry season.
2. I am glad that you have also added the number of training samples and added some equations to explain cross-validation and spatial variables contributing to tropical dry forest dynamics.
3. My main concern is still on the use of 2 images with only 3 years of separation to study land cover dynamics. What is the effect of extreme climatic conditions on the land cover? We all know that climatic conditions do not remain constant in any region; some years can be very dry or very wet. This is the reason that we encounter natural disasters due to flooding or drought, which will have a great impact on land cover. We also know that these disasters do not occur every year.
4. For the study on land cover dynamics, long-term data covering 10, or more years will be necessary.
5. The study will profit if you also include analysis of time series climate data (mainly precipitation and air temperature). Analysis of both data will help you to assess land cover change in relation to climate change patterns.
Author Response
- Corrections/additions have been carried out based on my detailed comments. However, I would have liked the answers with some supporting climatic data related to my question on 2 months gap between the Sentinel images. You mentioned that both images are in the dry season.
[Yes, both images were obtained in dry season, with 2 months difference. Since we didn’t analyze changes by comparing the images directly, instead we classified those two images using independent training samples for each image, and we detected changes by post-classification comparison which we believe could remove the effects of the 2-month gap in the images. Nonetheless, we checked annual climate data for 2018-2022. The temperature was rather stable, but the annual precipitation had variations, especially that the precipitation in 2022 was much higher than 2019. The interannual variation in precipitation could potentially cause false detection in change analysis. However, using post-classification comparison, and using verified changes for analyzing contributing factors, we minimized the effects of interannual climate variations].
- I am glad that you have also added the number of training samples and added some equations to explain cross-validation and spatial variables contributing to tropical dry forest dynamics.
[Thank you].
- My main concern is still on the use of 2 images with only 3 years of separation to study land cover dynamics. What is the effect of extreme climatic conditions on the land cover? We all know that climatic conditions do not remain constant in any region; some years can be very dry or very wet. This is the reason that we encounter natural disasters due to flooding or drought, which will have a great impact on land cover. We also know that these disasters do not occur every year.
[We agree with this observation. Although the 3-year change analysis still allowed us to capture the TDF gain and loss from shifting cultivation, an analysis with a longer time span, e.g., 10-30 years, could potentially allow us to have a more precise estimation of TDF gain (with a smaller confidence interval), and show a more complete picture of the TDF dynamics under the influence of shifting cultivation with crop and fallow cycles. As for the climate effect, in our study area, the extreme climate conditions would be drought and excess rainfall. Drought will possibly cause shortened period of greenness and excess rainfall will have opposite effect, causing elongated period of greenness and even delayed onset of deciduousness. All these seasonal and interannual effects will potentially cause problem and false detection especially in time series change detection, which is based on comparison between the observed values and modelled stable period of e.g., NDVI. However, we considered that this is not the case for our analysis, since we used a two-time classification comparison with independent training samples for each period, to observe changes, and especially we verified the changes based on visual interpretation using independent dataset, e.g., high resolution satellite images from Google Earth].
- For the study on land cover dynamics, long-term data covering 10, or more years will be necessary.
[We agree with this observation. An analysis with a longer time span, e.g., 10-30 years, could potentially allow us to have a more precise area estimation of TDF gain (with a smaller confidence interval), and show a more complete picture of the TDF dynamics under the influence of shifting cultivation with crop and fallow cycles. For future research, we would like to extend the study period to cover at least 10 years of time span using perhaps other data sources such as Landsat. For this research, since we used the surface reflectance collection of Sentinel-2A which only allowed us to study the forest cover change from 2019].
- The study will profit if you also include analysis of time series climate data (mainly precipitation and air temperature). Analysis of both data will help you to assess land cover change in relation to climate change patterns.
[We thank the reviewer for this suggestion. We analyzed time series precipitation data from CHIRPS “Climate Hazards Group InfraRed Precipitation with Station Data” and temperature data from “Land surface temperature MODIS” and observed a rather constant annual average temperature and some interannual variations in annual precipitation. We added annual climate data from 2018-2022 as a table in the discussion section and explained that although there is rather large difference in precipitation between 2019 and 2022, using our change analysis methods, we minimized the effects of climate data in the contributing factor modelling].
Reviewer 4 Report
minor revision to proofread this paper
Author Response
Thank you.
We went through the manuscript multiple times to identify and correct the possible mistakes and made extensive revision accordingly. We hope that this revised version can finally meet the standard to be accepted for publication.