Performance Evaluation of Feature Matching Techniques for Detecting Reinforced Soil Retaining Wall Displacement
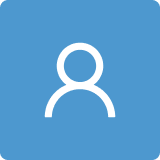
Round 1
Reviewer 1 Report
This study demonstrates that KAZE is the best feature matching method among the five methods through single and multi-block experiments, and proves the feasibility of KAZE method for accurate performance analysis of reinforced soil retaining walls. However, there are issues that should be addressed before the paper could be recommended for publication:
- In line 129-133, “a matching threshold of 10 was applied to binary feature vectors such as MinEigen, ORB, and BRISK, and a matching threshold of 1 was employed for KAZE and SURF”, why do different methods set different matching thresholds.
- In formula 4, why are there two ‘AREh1’? Please correct them
- In figure 1, in the first picture, the two points corresponding to outliner are between A and C, and in the second picture, they are between B and D. Please explain their meanings in detail. If there is a marking error, please correct it.
- In line 255, “KAZE and SURF have relatively high repeatabilities of 0.288–0.875 based on the results of 10 repetitions.” Is the result in Figure 3 one of the ten repeated test results? Is the rule in the other nine results the same as the rule in the figure ?
- In figure 5, the picture is not fully displayed, please pay attention to the modification.
- In line 340, “The KAZE method resulted in an ARE of less than 2 pixels between 50°-80°.” From Figure 8, we can see that ARE is relatively small at 30°-40°, but why only between 50°-80°. In addition, in Figure 3, repeatability is better when the low incident Angle as 5°-15°. What is the priority order of different indicators when determining the optimal incident Angle.
- In line 401-404, “However, in multiblock experiments, it is possible to detect and match relatively smaller or larger inlier matching features depending on the characteristics of the target image including size, position, and feature vector.” What does it mean?
- This study compares the feature matching performance of the five methods to illustrate KAZE's advantages, whether there is such a significant advantage when KAZE is used in actual engineering.
- Some References related should be added.
Improving dynamic soil parameters and advancing the pile signal matching technique. Computers and Geotechnics, 2013
Seismic time-history response and system reliability analysis of slopes considering uncertainty of multi-parameters and earthquake excitations. Computers and Geotechnics, 2021
Machine Learning Techniques for Vehicle Matching with Non-Overlapping Visual Features. IEEE 3rd Connected and Automated Vehicles Symposium, 2020
Author Response
The authors are thankful to anonymous reviewers for their valuable comments which were very useful in bringing the manuscript into its present form. The followings are answers to the referee’s comments. The paper including Tables and Figures was modified according to the referees’ comments.
Please see the attachment for detail.
Author Response File: Author Response.pdf
Reviewer 2 Report
The paper aims to evaluate the feature matching performance and to find an optimal technique for detecting three types of behaviours—facing displacement, settlement, and combined displacement—in reinforced soil retaining walls.
The work is well described and the exposition is quite rigorous. From my point of view, I have no specific comments on the text. I just want to point out that the authors should better highlight that the results are more theoretical than practical because they refer to particular laboratory conditions. The results should be confirmed in real situations.
Author Response
The authors are thankful to anonymous reviewers for their valuable comments which were very useful in bringing the manuscript into its present form. The followings are answers to the referee’s comments. The paper including Tables and Figures was modified according to the referees’ comments.
Please see the attachment for detail.
Author Response File: Author Response.pdf
Reviewer 3 Report
The authors investigate the problem of assessing earth reinforced retaining walls. They are attempting to automate and improve on the manual inspection process. Their research is in two phases. The first, reported in this paper, is to identify feature points in images of the wall and match them through a sequence taken at widely separated times. The second phase is to estimate the development of the 3D structure of the wall as represented by the features.
The present paper reports experiments that use the feature detectors available in Matlab, they investigate these feature detectors’ ability to detect features and subsequently match them across image pairs that differ in illumination and orientation. Natural and artifical targets are employed.
The description of the evaluation matrics is obscure. Perhaps it would be clearer to give a simple list and describe the metrics using equations. Ultimately a metric to quantify the accuracy of the feature matching is derived.
They report a thorough experiment to identify the optimum viewing angle and feature detector for feature matching. This is by capturing images of a single block with controlled displacement between images, and using all of the feature detectors available in Matlab. Whilst the feature matching is performed using the feature detectors, the evaluation metric requires the corners of the block – these are indicated manually at present. At least, that is my understanding of the paper.
There is a further experiment using images of a wall built and imaged under lab conditions. The feature detector identified as the best in the single block experiment was used. Artificial targets were used to estimate block motion, as well as so-called natural targets – the visible patterns on the block. Repeatability measurements under different displacements were computed, the ATs and NTs gave similar results.
Minor corrections
132 A matching threshold of ... The specific value doesn’t mean anything without a definition of the descriptor. Perhaps omot the mention of a specific threshold.
138 The text suggests that a pair of detected features in two images will match if the projection of one into the second image matches the location of the second feature to within two pixels. But this can only work if the wall has not distorted. Any comments?
151 Clarify “through an adjusting type of feature detector”
159 what is the “minimum number of features detected in the image pair”?
And “target image at initial”?
189 Don’t need the brackets around in (Ai)TOD etc in eq 3
190 I don’t understand the statement in this paragraph, why can’t you compute a registration error for the same or the desired points?
One of the ARE subscripts in eq 4 is incorrect, one of them should be h1
198 at the initial after the behavior in the transformed image -> in the initial image, in the subsequent image and in the transformed image
Vertexes -> vertices (and probably elsewhere)
Queries
199 A transformation is estimated using matching features Ei -> En. The transfomation is then applied to the corners of the block, and an error is computed based on the difference between the transformed corners and the corners in the second image. My question is how do you find the block’s corners once you’ve found a target or a feature within a block?
The block displacement seems to be measured using a fixed camera, as far as I can understand. Is this a realistic proposition in the field?
A single transformation is used to map all the features in the wall images. Is this reasonable if we have a wall that is deforming – different portions of the wall will have different transformations?
Author Response
The authors are thankful to anonymous reviewers for their valuable comments which were very useful in bringing the manuscript into its present form. The followings are answers to the referee’s comments. The paper including Tables and Figures was modified according to the referees’ comments.
Please see the attachment for detail.
Author Response File: Author Response.pdf
Round 2
Reviewer 1 Report
English should be revised.
Reviewer 2 Report
The paper has been further improved thanks to the comments of the other authors and can be accepted for publication.
Reviewer 3 Report
The revised paper has addressed all of my concerns apart from point 2 in the authors' response. My comment addressed the problem of the wall being distorted as it's in the process of collapsing, but in their response the authors have interpreted this as the problem of camera distortion. However, I'm happy that this problem is addressed implicitly in the paper.