An Explainable AI System for the Diagnosis of High-Dimensional Biomedical Data
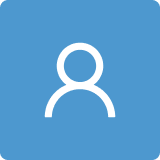
Round 1
Reviewer 1 Report
Comments and Suggestions for AuthorsIn this manuscript, the authors introduced a novel explainable AI (XAI) method, ALPODS, designed for advanced flow cytometry data samples. They assert that ALPODS diagnoses with human-like accuracy while maintaining decision transparency through domain-specific fuzzy reasoning rules. This claim is substantiated by comparing ALPODS to leading XAI systems on benchmark and routine datasets.
Comments and suggestions:
The authors had a comprehensive explanations of the background and methods, and compared multiple test scenarios. Overall, this is an inspiring study with multiple practical applications. I have one suggestion to make this manuscript more comprehensive: the authors compared multiple methods in this manuscript, is it possible for them to have a consistency study for these different methods? For example, have some feature importance ranking and check whether they could generate a similar set of top features or rules on the same dataset.
A suggestion on text editing: can the authors use a "," for a thousand-separator comma instead of “.” to distinguish with a decimal point, for example, in lines 98, 160 “n >= 100.000”?
Author Response
in pdf:
Author Response File: Author Response.pdf
Reviewer 2 Report
Comments and Suggestions for AuthorsReview comments:
1) Table 2 is not well formatted. Kindly reform it.
2) The procedure to divide training and test sets is not clear. Is it by cross-validation or leave one out or other method? Kindly explain.
3) Add statistical significance test (anova 1) during pairwise comparison.
4) Add any noise removal method (say, DBSCAN algorithm or similar) if possible.
5) Add objective in the introduction and future scope in conclusion.
6) Add more comparison between the current study with state-of-the-art methods.
Comments on the Quality of English Language
English writing is more or less fine.
Author Response
in pdf:
Author Response File: Author Response.pdf
Reviewer 3 Report
Comments and Suggestions for Authors1. auto hyphenation can be turned off
2. why LIME is preferred over SHAP model?
3. which classifier is used with ALPODS? can be specified in abstract and other places.
4. Lot of references are cited. good
5. Overall work flow diagram missing
6. sample data/images from datasets can be added for better understanding.
7. graph or charts of comparing ALPODS with other models is missing.
Author Response
in pdf:
Author Response File: Author Response.pdf
Reviewer 4 Report
Comments and Suggestions for AuthorsThe second key contribution is hard to understand: "low number of cases in the of the part if the dataset used for learning"
Fourth key contribution: is there are reason that Interoperability is capitalized?
DAG is introduced in section 3.2 but only written out in section 3.3
Line 252: why is Data Scientist capitalized?
Line 327: if this is the Iris dataset from the UCI Machine Learning Repository, it provides no meaningful information. It is an absolutely trivial classification problem. The Iris dataset has no place in a scientific paper about novel machine learning approaches. In particular, the description of how the data set was modified with noise tells us that a synthetic data set would have been more meaningful. Furthermore, ALPODS performs terribly on the Iris dataset. This paper would be more meaningful if Iris experiments were removed.
In general, this paper seems to try to do too much. It's not clear if this is about visualization, psychology, machine learning, or even XAI. For example, the authors extol the virtues of neural network models in their introduction, but then avoid them in any experimentation. Is the paper then not about choosing the best ML model? The authors discuss putting explanations in the form of the domain expert, not the data scientist, but they don't go into significant detail about what those explanations actually are. Likewise, they insist on 7 rules, based on a psychology paper. Does this number apply to domain experts, who may have spent decades in their domain, and likely have a much higher capacity for understanding in that domain than the average person?
I don't know that I have specific requests for change. I feel that it trying to do too much and so loses focus.
Comments on the Quality of English Languageplease see the required radio button above.
Author Response
in pdf:
Author Response File: Author Response.pdf
Reviewer 5 Report
Comments and Suggestions for AuthorsThe authors present study on the relevant topic - explainable AI. They study it in a particular domain - cytology. I appreciate that authors considers not only performance of the models in terms of the classification accuracy, but also from the point of processing time
Introduction section adequately describes relevance of the research. However other parts of the paper could benefit from improvement:
- manuscript will benefit from diagram, describing workflow of the proposed algorithm, as at the current time is relatively hard to comprehend what is actually happening in the ALPODS despite rather detailed description and prseudocode. I suggest to include figure 6 from the supplementary material in the manuscript itself.
- since on authors focuses on the explainability of the models I think it is of high importance to highlight the process for the description rules generated by ALPODS, which are presented in tables 4-6. I suggest to provide specific step-by-step presentation for description rule for one particular Cell type. And compare explanations provided by different methods
- authors should consider adding explanations why they used Random Forest as a baseline model, and not one of the Gradient Boosting algorithms, which are usually optimized for a faster processing time. Also it is worthy to comment on why Shap explanations were not considered
- some small mistakes / typos that were noticed:
* in table 6 the accuracy of RF-FAUST is the same for all datasets
* in table 6 there is (supposedly) extra mantissa in line average number of events per case (330.000 vs 330 000)
* figures 3 and 4 description implies "red dots", however on figures presented only purple, gray and blue. also it is not explained what are gray and blue dots.
* in lines 371, 373, 374 authors write "100,000", "700.000" and "440.00"
* in some case authors present accuracy not in the percentage form (f.e. in table 2 accuracy for RF-FAUST is 0.92, same goes for line 498)
Author Response
in pdf:
Author Response File: Author Response.pdf
Round 2
Reviewer 1 Report
Comments and Suggestions for AuthorsThank the authors for the detailed explanations and updates on the manuscript. Really appreciate their hard work. They addressed all my comments and suggestions for the previous vision.
Author Response
It seems this reviewer accepted the manuscript. There is no further comment to revise the manuscript.
Reviewer 3 Report
Comments and Suggestions for Authorsauthor made necessary changes based on review comments
Author Response
It seems this reviewer accepted the manuscript. There is no further comment to revise the manuscript.
Reviewer 5 Report
Comments and Suggestions for AuthorsIt appears that authors have adequately reacted to suggestions of several reviewers
Author Response
It seems this reviewer accepted the manuscript. There is no further comment to revise the manuscript.