Development of a Real-Time Vespa velutina Nest Detection and Notification System Using Artificial Intelligence in Drones
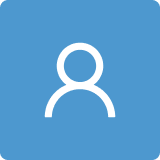
Round 1
Reviewer 1 Report
[Summary of the Study]
This research endeavor aimed to devise a real-time drone-based and AI-driven system for the detection and notification of V. velutina nest locations. The outcomes indicated impressive precision at 100%, a recall rate of 92.5%, an accuracy score of 99.7%, and an F1 score reaching 96.1%.
[Positive Aspects of the Study]
The study addresses the pressing need for real-time V. velutina nest detection, crucial for beekeepers. Furthermore, the inclusion of a real-time notification system enhances the applicability and practicality of the developed system.
[Negative Aspects of the Study]
Despite a commendable conceptual foundation, several research procedures lacked careful consideration, rendering the study incomplete. Weaknesses in the manuscript include a limited literature review, resulting in a lackluster Discussion section. Additionally, the study didn't account for whether V. velutina nests were active or vacant, and numerous grammatical and stylistic errors marred the text.
[Major Comments]
**Introduction**
1. The introduction contains statistics and numbers without proper citations, potentially leading to plagiarism issues. Citations should be added to substantiate these facts.
2. The study should incorporate existing methods for V. velutina nest detection to provide context and relevance. A recent article on V. velutina detection using drones published in "Drone" early this year should be cited and discussed in this manuscript.
3. The authors should consider exploring the feasibility of detecting smaller-sized nests to prevent extensive damage to honeybees, and specify the size threshold for "large" nests.
**Methods/Results**
1. The timing of the study from October to before overwintering may not sufficiently assist beekeepers in protecting honeybees before October when their activity decreases. The authors should clarify how the system can aid beekeepers when they need rather than focusing on when would be the best time for drones and AI to detect V. velutina nests.
2. Line 127: flying the drone at 65-150 m above sea level does not explain how high the drone flew over the canopy of trees; land elevation changes over landscapes. Please add the flight altitude of the drone above the CANOPY so that other scientists can replicate the study.
3. Lines 128-129 “In the apiaries indicated by blue circles in Figure 2, V. velutina nests within 1 km of the surrounding area were detected”: How were the authors sure that they find all V. velutina nests (shown in Figure 2) in 1 km area? Did the authors conduct any ground surveys in 1 km areas? If they did, please explain how they did it because it is quite a difficult task to check individual trees to locate V. velutina nests in hilly or mountainous areas.
4. Figure 2: Blue dots are for apiaries, but I had to read the manuscript to figure out what they mean. Please add the explanation directly in the figure caption.
5. Lines 134-139: this part is quite important to understand what data were used in this study but was so vaguely written. Here are several questions: (1) Were the video images used or were photo images used? I could not imagine that the authors had 10,095 images with V. velutina nests from photo images. So, I think the authors used frames of video images for the analysis, but it is hard to know based on the current manuscript, (2) although there were 10,095 images with V. velutina, there were only 19 V. velutina nests to generate 10,095 images based on what I understand from the manuscript. This means that there were about 500 images generated for each nest. Surely, it is possible in machine learning standpoint, but in biological standpoint, I hoped that the authors had more actual V. velutina nests than 19, (3) again, my big question and concern is how the authors were sure that the “image data without V. velutina nests” actually didn’t have any V. velutina nests unless they census the study areas because some V. velutina nests could be there but not visible from the drone.
6. All FP and FN were calculated for machine learning but not for the detection of actual V. velutina nests. In other words, the authors didn’t test FN for the machine learning capability because in section 3.3, they just tested for FP, not for FN. So, we still don’t know how much the drone and AI capability miss V. velutina nests that cannot be detected by the drone. Please note that there are numerous V. velutina nests that can be seen from the ground but hardly seen from the sky, and this study ignored that.
7. The image size has been increased from 640*480 to 1280*960 but processing speed is the same 30 frames/s while frame/s decreases with increase in image size in other cases in Table 1. This part is very confusing.
8. The description for Figure 7 indicated that the best result was obtained for the higher resolution of 3840 but the figure shows that the resolution of 3840 has the lowest value. Also, the image hasn’t been incorporated properly as the values in the images are cut off.
9. The site for the validation is very less with just one site and only 4 nests being detected. Additional sites for the validation would have made the study more robust and the results more reliable.
**Discussion**The discussion seems to be reluctantly written, and this part needs really intensive major revision. Even, they failed to do a literature review on aerial detection of insects or even V. velutina nest detection published in the same journal early this year. If this manuscript is rejected, the Discussion section would be one of the major reasons for rejection.
[Recommendation]
- The rationale, justification, and objectives of this study are good and very important. I would like to see this type of technology being further studied and actually used for apiaries. However, I notice that the field experiment has some flaws and was not well explained in the manuscript. Also, the authors seemed very reluctant to do literature review based on the lack of references in many sentences and ignored the importance of Discussion section. At least the writing part can be fixable, but it would require serious revision while some missing part of field experiments might not be fixable. Therefore, I suggest the author withdraw the manuscript (I need to reject it as there was no option for withdrawal).
There are numerous grammatical and stylish errors. However, those errors can be readily corrected.
Author Response
please refer to the attachment
Author Response File: Author Response.pdf
Reviewer 2 Report
This is a very interesting paper, exploring the possibilities of combining AI and drone technology. However, the exact aim of the study, and what was accomplished, is a bit unclear, and the methods needs more work.
It is recommended that this study makes it clearer what was developed (a preliminary system that can geolocate wasp nests in-flight?) and conduct more tests of the system to have more results to show. Precision, Recall, etc. of a trained neural network is not enough of a result itself, as it should be verified by extensive application in situ. Determining that photos with a higher resolution is better data for training, than photos with low resolution, is too trivial.
Abstract
This should be more to the point. It is repeated that “four V. velutina nests were detected” (line 26).
Keywords: Please add "YOLOv5"
Introduction
It is interesting reading, but one could question the relevance of many of the details. Keep the aim of the study in mind and make it much more to the point.
The use of references should be much more precise. Several different statements (e.g. line 38-58) are simply combined in [1-5] references, making it difficult to check the individual statement.
Line 59: Does AI really “allow machines to have reasoning and judgment capabilities”?
Line 59-83: lacks several references - this is a general problem in the paper.
The Aim of study should be more concise. Keep in mind past/present tense.
Materials and Methods
Please make it clearer what was directly developed by you, and what was developed by others and then combined by you to make up the “system”. Did you tweak the code of a “generic” version of YOLOv5, or did you simply use it to train a custom neural network for detecting V. velutina nests?
Is the power supply unit relevant? Simply state the air time of the drone.
That the drone is made up of FC, GPS, GCS, etc. seems a bit too trivial. Were these parts developed by you? If not, please add brand/model.
Please elaborate on the Database. Was the obtained data uploaded real-time or manually after the flight? If the latter, then what is the point of the system, instead of just using a generic drone to film/photograph an area, and then analyze the footage afterwards?
Please make it clearer to the reader that 2.2.1. Data Acquisition and Preprocessing is concerning the training data for the neural network, not the object detection and geo-location results (?) of the paper.
Figure 2: Where these nests detected using the system? Then this should be results? Or were these nests simply used to gather training data?
Technical jargon of the machine learning methods should be more precise, e.g. object detection instead of artificial intelligence learning, etc. Maybe consult other experts within the field of computer vision? It is unclear which version of YOLOv5s was used, and the exact parameters chosen for training and detection (it is somewhat mentioned in results). The NVIDIA GeForce is a GPU (which both training and detection relies heavily upon), not a CPU.
Are all the details of the YOLOv5 structure etc. relevant?
Several papers discuss the flight height when obtaining the training data vs. the flight height when detecting, and the uses of YOLOv5 (and newer models). This should be investigated more.
2.4. Model Learning Evaluation
Were the True positives/negatives, Precision, Recall, Accuracy etc. checked and calculated manually? If not, how was this obtained?
Results
Large parts of this section belong in the method or discussion section.
Was the false detection assessment made automatically or manually? This can make a big difference and depends highly on the composition of the training data and the verification data, but the assessment of the trained neural network is not robust results. It should be tested in situ in a larger scale.
3.3.2. Demonstration Detection Flight: This should be conducted on a larger scale, to provide solid results of the system. Also, the confidence threshold should be mentioned and elaborated much more. What is the lowest acceptable limit, etc.? The method of testing the system should also be refined: Do the system miss nests (false negatives) or generate false positives? What is the time spent/flight height/precision-ratio? Can it be optimized? It is very promising that the system can automatically provide the location of the nests, but several other methods can do the same, by analyzing “generic” drone footage subsequently.
Discussion
The literature of AI analyzed drone footage (using computer vision and YOLO) should be explored more, several relevant references are missing.
Different font in line 328-330.
Line 332: “… and studies are being exterminator them” - meaning unclear.
As the development of AI is advancing with incredible pace, manual annotation and supervised learning might already be outdated. Please elaborate on why the approach of this paper is still relevant, or give examples of how newer methods can provide the same training data etc.
Conclusion
Did the study really “reduce the damage to the apiary in the following year”?
Did the study test several flight altitudes?
This section should be much more concise, parts of it belongs in discussion, and therefore makes it unclear what the study did exactly.
Line 349: “… enabling them collect more information…” - please add “to”.
The precision, recall, etc. of the neural network should be tested in situ.
Is it really a result that the four nests were detected, when their location were known beforehand?
Please proof-read for grammatical tense.
Author Response
Please see the attachment
Author Response File: Author Response.pdf
Reviewer 3 Report
The article presents a study on the invasive wasp species, Vespa velutina, using drones and their detection capabilities through artificial intelligence. Overall, the article is lucidly written and offers valuable insights. It undoubtedly contributes novel information to the field and is fitting for publication in the "Drones" journal. However, I have several suggestions that could enhance the article's quality. With these minor revisions, I am confident it would be ready for publication.
In the introduction:
The precision of the cited publications could be improved. Specific details are mentioned without appropriate citations. For instance, a source should be provided when stating that "75% of crops are pollinated by pollinators." Additionally, references should be given for the year Vespa velutina was introduced and the extent of damage it has caused. Attention should be paid to this aspect throughout the article. It would be beneficial to avoid clustering all citations at the end of a paragraph.
I recommend incorporating a brief review segment. This should consist of a concise paragraph detailing the amalgamation of fieldwork methods in ecology using drones (e.g., Sikora & Marchowski 2023) and the application of artificial intelligence in ecological studies. It would be enriching to compare works from analogous domains, such as studies on birds, mammals, or reptiles. While I have provided a few examples (e.g., Marchowski et al. 2021, Weinstein et al. 2022), I encourage the authors to delve deeper into relevant literature. This would position the article more effectively within its research context.
Regarding the Discussion section:
It currently feels somewhat constrained. I suggest expanding it to be more comprehensive. The discussion could benefit from referencing publications beyond just those related to insects. It should encompass other domains where drones and artificial intelligence have been employed. Emphasis should be placed on the novelty of the method described by the authors.
Formatting suggestions:
The font type used throughout the article should be consistent.
The referencing style needs standardization. At present, there are inconsistencies in the representation of authors' names—sometimes they are abbreviated, sometimes they are in uppercase, and at other times, they are written out in full.
Sikora A., Marchowski D. 2023. The use of drones to study the
breeding productivity of Whooper Swan Cygnus cygnus. The European
Zoological Journal 90:193-200.
Weinstein et al. 2022. A General Deep Learning Model for Bird Detection in High-Resolution Airborne Imagery. Ecological Applications 32( 8): e2694.
Author Response
Please see the attachment
Author Response File: Author Response.pdf
Reviewer 4 Report
#611 words
Reviewer 2:
The manuscript is of great interest for the pipeline developed in the detection of Vespa Velutina nests during late autum/winter time (i.e.no leaf, and big nest to be detected)
The authors descibe their hardware and software setup as well as the analyssis of the frames from the camera, unfortunately the level of details is not sufficinet for the manuscript to be accepted.
the english of the manuscipt has to be revised in order to be more fluent
secton 2.3 need revriting to a better form
The paraghraph about discussion is pointless and do not contain reelevan inofrmation for the reader, it just report the content of the reference [23] and other litterautrre without further analysis of the data
The authors are strongly encorage to openly relese their pipeline if they consider it can be of practical use. in this way more resercher but also people in the field can benefit from the authors effort and improve the fight against V.velutina
The authors are encoraged to unifor the use of terminoogy V.velutina is sometimes calle (bee, wasp, hornet)
reference [14]&[22] are not cited in the text of the manuscript
Please find the major comments below referenced by manuscript line number, meanwhile minor comments are marked in the PDF manuscript.
# abstract
L15: would help adding (i.e. ~130 million USD) or equivalent
L18: can be shortened to V.velutina and all the following as well
L19: did you mean 'hornet'?
L19: as for L18
L25: as for L18
L26: to be deleted, repetition below
# introduction
L30: maybe 'needed' suits better the sentence?
L43: as suggested at L15
L43: Please proveide some reference to support this statement
L49: change to 'as'
L50: maybe be relevant to report about the bio-cycle of V.velutina that there are 2 kind of nests: i) primary nest ( smaller where the queen of the year develop, ii) secondary nest ( the massive big one)
In order to control V.velutina would be best to identify the frst rather than the second due to obvius reason that the 300-500 queens don't get mated at all. Please develop further and support with some reference te statements.
L78: ????
L86: ?? m,aybe to the date of last flight is better
L90: change to: the aim of this paper
# materials and methods
L134:139 need clarification
L134: please also add reference to the labelling software used
L134: the training conditions of the 4 models used need to be described in more details and in as clear manner similarly has it has been done in the validation section
L136: what exactly is it meant her for 'pieces of data' ?!!!!
L168: for 'object' detection
L197: the greek letter 'eta' do not appear in any of eq 1 to 6. where should be referring to?
L225: references should be added on the exact model used during training
#discussion
L328: pease avoid copy/pastye and use the same font in all the manuscript
# coclusions
L344: this manuscript describe a detection pipeline not the removal
Figure2: would be helpful to ad a scale bar to one of the sub images
Figure2: the georeferencing of the position of the nest should be located more accuratly in the images since coordinates are known.
Figure3: would be helpful to ad a scale bar to one of the sub images
Figure3: need to be included with better resolution, noting besides a greenish images is visible
Figure7: please increase the resolution of the image in the mauscript, even do the image for the camera has lowert resolution the bounding box should appear crispy and sharp and not pixelated as it is
Figure9: please improve the quality of the image
Comments for author File: Comments.pdf
Author Response
Please see the attachment
Author Response File: Author Response.pdf
Round 2
Reviewer 1 Report
The authors fairly responded to my concerns on this manuscript. However, I am still not sure why the authors are so reluctant to write more to make the discussion section better. There are numerous items they can discuss based on their findings.
Specifically, the authors tried to explain some specific situations of Korea (weather, wasp phenology, apiary management, etc.) in their response letter. If the authors think the Korea-specific situation matters as shown in their response letter, then they should explain them in the manuscript as well (the discussion section might be the best place). At minimum, the authors' responses 3, 4, 9, and 12 need to be added to the Discussion section.
There are a few grammatical and numerous stylish errors in the manuscript. The authors need to check the manuscript very carefully to make sure the manuscript is written without such errors.
Author Response
Please see the attachment
Author Response File: Author Response.pdf
Reviewer 2 Report
No further comments
Please proofread for past/present tense
Line 103: "The drone is equipped with..."
Author Response
I corrected the grammar overall.