An n-Sigmoid Activation Function to Improve the Squeeze-and-Excitation for 2D and 3D Deep Networks
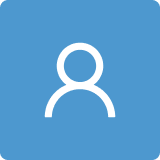
Round 1
Reviewer 1 Report
This paper is about improving the network by introducing a new SE block.
However, is it not targeting any specific issues or domains?
The abstract not clearly showing the results. No contribution list. No Related work, authors have given the title but unfortunately no details. How can section 2 have subsection 3.1??
Proposed methodology is not discussed with a figure. What is the research gap??
Results are not justified with any performance metrics.
No discussion section.
No proper comparison with other works
Author Response
Reviewer 1
As authors, we would like to appreciate the time and effort that you have spent to review this paper. Please accept our gratitude.
This paper is about improving the network by introducing a new SE block.
However, is it not targeting any specific issues or domains?
The target is classification and segmentation for 2D and 3D data. We have outlined it in the abstract and in pages 2, page 12 (twice) and page 16.
The abstract not clearly showing the results. The improvements results have now been added in the abstract.
No contribution list.
The main contributions of this work have now been clearly shown in the introduction with 4 points.
No Related work, authors have given the title but unfortunately no details.
Research involving the modification of the gating mechanism (sigmoid) have not really matured but the paper has highlighted the main works such as Psigmoid.
In the related works, we have now clearly added other works focusing on the Squeeze and Excitation block to improve the neural networks towards the end of section 2.3. Related works have been divided in 3 main blocks such as squeeze and excitation, activation functions and channel and spatial correlations which the main areas of focus for the paper.
How can section 2 have subsection 3.1??
We have made the changes and verify throughout the paper to ensure that such occurrence do not appear again.
Proposed methodology is not discussed with a figure.
We have introduced in the proposed methodology a new figure (Figure 2: schematic of the Resnet module using a SE block with a n-sigmoid) and have explained the role that the n-sigmoid is to play along with a brief discussion of it.
What is the research gap??
The research gap is to improve SE by increasing learning capacity through the reduction of the gradient descent problem as well as retaining more useful features. For this a new activation function to act as gating mechanism was proposed. Please refer to modified Abstract and Proposed method.
Results are not justified with any performance metrics.
Training accuracy is the performance metric chosen for this work as now shown in the experiments results (refer 4.1 Dataset and evaluation metric).
No discussion section.
We have added a discussion section (Refer section 4.5).
No proper comparison with other works
In experimental results, comparison with other works are shown (please refer Table 1, 2, and 3). Only the 2D Unet, 2D Resnet and 2D DenseNet as well as 3D Unet were selected.
Observations:
The paper has been thoroughly modified to meet the remarks of the reviewer.
Reviewer 2 Report
The paper introduces a new sigmoid activation function to improve Neural Network performance by resolving the issues of vanishing gradient and numertical indeterminacy. The paper structure is clear and easy to fellow.
The key point of this paper is the n-sigmoid algorithm (Eq.5,6,7,8). However, the detail is missing. The figures related to the algrithms are too simple and miss important explantions.
1. Line69, change (2) to (3).
2.Figure3, 4, 5 add figure axis. Figure should explain itself, just put a simple function name as figure caption is imprecise.
3.Line312-314, discussion need to be added about why the loss is acceptable for n-sigmoid.
Author Response
Reviewer 2
As authors, we would like to appreciate the time and effort that you have spent to review this paper. Please accept our gratitude.
Comments and Suggestions for Authors
The paper introduces a new sigmoid activation function to improve Neural Network performance by resolving the issues of vanishing gradient and numerical indeterminacy. The paper structure is clear and easy to fellow.
The key point of this paper is the n-sigmoid algorithm (Eq.5,6,7,8). However, the detail is missing. The figures related to the algorithms are too simple and miss important explanations.
All figures have been modified to included legends, titles and axes. Better explanations have been provided.
- Line69, change (2) to (3).
Thank you. We have made the change.
2.Figure3, 4, 5 add figure axis. Figure should explain itself, just put a simple function name as figure caption is imprecise.
We have improved the figures to add axis, legends, and font sizes.
3.Line312-314, discussion need to be added about why the loss is acceptable for n-sigmoid.
A discussion was added to explain that the n-sigmoid validation loss was lower than the others which goes to show that the n-sigmoid performs better.
Reviewer 3 Report
Dear Authors
I satisfied with the new approach presented in the manuscript. I suggest the following minor revisions:
1- Please support abstract with results (numbers).
2- Figures (from 4 to 8) need redrawing by increasing fonts and colors.
3- Please explain the derivation of Eq.(5). This is useful for young researchers
4- While revision process, I hope you can add references published in 2022 and 2023.
Author Response
Reviewer 3
As authors, we would like to appreciate the time and effort that you have spent to review this paper. Please accept our gratitude.
Comments and Suggestions for Authors
Dear Authors
I satisfied with the new approach presented in the manuscript. I suggest the following minor revisions:
1- Please support abstract with results (numbers).
We have added results for all experiments.
2- Figures (from 4 to 8) need redrawing by increasing fonts and colors.
We have made the changes.
3- Please explain the derivation of Eq. (5). This is useful for young researchers.
We have added a line to explain the Calculation.
4- While revision process, I hope you can add references published in 2022 and 2023.
We have used more recent references instead.
Thank you very much.
Reviewer 4 Report
Please find the attached file.
Comments for author File: Comments.pdf
Author Response
Reviewer 4
As authors, we would like to appreciate the time and effort that you have spent to review this paper. Please accept our gratitude.
- There are several acronyms (i.e., RNN, CNN, ELU, Resnet) in the manuscript that lack the proper introduction of their full names when they are first used.
All acronyms have been introduced severally now that remove any ambiguity in the text.
- On page 7, line 267, there is a typo. I believe it should be ”lack”.
The typo “lac=k” have been changed.
- For all Figures, it needs further descriptions. All the details of the Figures should be well explained in the captions below (i.e., in Figure 11, the explanation in the image is blurry), as well as the main paragraphs (i.e., the trend of Figures 7 and 8 are not explained). More importantly, there is no information of axes in the data representing figures. It is hard for the readers to know what X and Y axes stand for.
We have added better explanations in the captions and axes.
- On page 5, the n-sigmoid function was proposed as the new activation function. It is quite abrupt to me for this proposal. Is there any specific reason for proposing this function? How is this function derived? Can the author explain these factors in detail?
A new structure has been adopted where the proposed method starts with a Squeeze and Excitation (SE) block with n-sigmoid where the role, the position in the SE of the proposed n-sigmoid is clearly defined and briefly explained. This is followed by an explanation of the sigmoid, its challenges regarding saturation and vanishing gradients, a reason why the change occurs at the gating mechanism of the SE and finally the proposed n-sigmoid is introduced.
- On page 6, lines 218-220 and 222-223 are repeated.
We have removed lines 222-223.
- On page 11, lines 408-413, the authors claim that the value of α could influence the final result. However, the improvement of the new activation function is small. In some of the cases, the total improvement is only 0.25%. What are the real influences of α? By choosing a different α value, can the result show deterioration instead of improvement? Further discussion is needed to prove that the data supports the claim of the manuscript.
In section 4.4.1.b the authors explain that the change in the value of has revealed that the lesser the value chosen for lesser the accuracy becomes.
Thank you very much.
Round 2
Reviewer 1 Report
A n-sigmoid Activation Function to Improve the Squeeze-and- 2 Excitation for 2D and 3D Deep Networks
The authors have revised but still the paper needs more modifications.
1. Literature review needs a table to summarise the state-of-the-art work with results and limitations
2. Section 4.1 says Datasets and Evaluation metrics. But, no performance evaluation metrics are discussed. Please discuss the performance evaluation metrics in detail.
3. Where is accuracy graph??
4. Discussion section looks very week. Please elaborate it more.
5. Where is comparison study section. Make a separate section with name comparative analysis and insert a table and show how your work is better than at least 3-4 references (latest papers). Compare with all the metrics.
6. Please also add Limitations and Future direction sections.
Author Response
As authors, we would like to really appreciate all your efforts to make our paper an excellent paper. We would like to really express our gratitude.
- Literature review needs a table to summarise the state-of-the-art work with results and limitations. A table (Table 1) has been added that show other works, their results and limitations.
- Section 4.1 says Datasets and Evaluation metrics. But, no performance evaluation metrics are discussed. Please discuss the performance evaluation metrics in detail.
The paper has been modified to respond to the reviewer suggestion. Sections 4.4.1.b and 4.4.2.b have been renamed Comparative analysis where the performance metrics are succinctly discussed and the training Accuracy and Loss graphs have been added (Figure 12, 13 and 15). Tables 3 and 4 have been redesigned to show more clearly the comparison differences between the proposed n-sigmoid SE block and other SOTA methods.
- Where is accuracy graph?? Accuracy graphs have been added (Figure 12, 13 and 15)
- Discussion section looks very week. Please elaborate it more.
Discussion section has been modified and divided in 3 sections (Impact of using other activation functions, The vanishing gradient solution, Future research directions) as well as a brief discussion on the performance metrics results at the beginning.
- Where is comparison study section. Make a separate section with name comparative analysis and insert a table and show how your work is better than at least 3-4 references (latest papers). Compare with all the metrics.
Sections 4.4.1.b and 4.4.2.b have been renamed Comparative analysis where the performance metrics are succinctly discussed and the training Accuracy and Loss graphs have been added (Figure 12, 13 and 15). In both 2D and 3D, SOTA methods have been added as comparison tools.
- Please also add Limitations and Future direction sections.
Sub-section 4.5.3. discusses the future directions after a brief list of the challenges that the n-sigmoid activation encounters. Thank you very much.
Notes:
To improve the method description, the authors have removed the sub-section regarding the sigmoid function and that discussing its challenges. This way the reader can directly face the proposed methodology (use in a convolutional neural network) directly followed by a description of the n-sigmoid.
Reviewer 2 Report
My previous concerns are well addressed in the revised manuscript.
Author Response
The authors highly appreciate your time and positive comments.
Round 3
Reviewer 1 Report
The authors have responded to my previous comments and the paper can be accepted now.