A High-Accuracy Mathematical Morphology and Multilayer Perceptron-Based Approach for Melanoma Detection
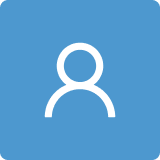
Round 1
Reviewer 1 Report
The manuscript proposed an image feature-based classification method for detection of melanoma from dermatological images. While there exist other convolutional neural network-based methods that can achieve similar (or possibly better) performance for the task, it will be useful to investigate how morphological (e.g. size, symmetry) and texture (e.g. histogram) features are linked to malignancy of the melanoma, through explicit feature extraction-classification methods as in this work.
However, even with a very simple MLP structure being used, the manuscript did not discuss feature importance nor correlation among features at all. It is highly suggested that the relationship between extracted features, the class labels, and prediction performance should be discussed in-detail. How exactly does the symmetry, edge, color and size in dermatological images are helping in detection of melanoma will be the real contribution of this work.
Further, structure of MLP was not described clearly within the manuscript. From Fig. 7 it seemed that the network has only one hidden layer. While that is acceptable (and actually beneficial for feature analysis), the reviewer suggests an additional experiment using a deeper network to investigate performance changes.
Some comments for clarification of reading and scientific soundness:
Review of recent works of dermatological image analysis, especially those utilizing deep learning methods, shall be added. “Edge” feature definition in Algorithm 6 is ambiguous and lacking explanation for its rationale. Size of the dataset, and details of training/testing split shall be provided. In caption of Fig.11, name of the methods for comparison shall be provided along with their reference.Author Response
Reviewer 1
The manuscript proposed an image feature-based classification method for detection of melanoma from dermatological images. While there exist other convolutional neural network-based methods that can achieve similar (or possibly better) performance for the task, it will be useful to investigate how morphological (e.g. size, symmetry) and texture (e.g. histogram) features are linked to malignancy of the melanoma, through explicit feature extraction-classification methods as in this work.
However, even with a very simple MLP structure being used, the manuscript did not discuss feature importance nor correlation among features at all. It is highly suggested that the relationship between extracted features, the class labels, and prediction performance should be discussed in-detail. How exactly does the symmetry, edge, color and size in dermatological images are helping in detection of melanoma will be the real contribution of this work.
Thank you for your suggestions. In the latest version of the manuscript, we showed in Table 3 a comparison of accuracy in classification when different descriptors setup are used. It can be observed that when just a descriptor is used the more relevant descriptor is color followed by symmetry. When two descriptors are used the best combination is symmetry and dimension. Finally, the best setup when three descriptors are used is the edge, color, and dimension.
Further, structure of MLP was not described clearly within the manuscript. From Fig. 7 it seemed that the network has only one hidden layer. While that is acceptable (and actually beneficial for feature analysis), the reviewer suggests an additional experiment using a deeper network to investigate performance changes.
Now, Table 2 shows a comparison of accuracy when different MLP architectures are used, justifying the choice of architecture 4-1024-1.
Some comments for clarification of reading and scientific soundness: Review of recent works of dermatological image analysis, especially those utilizing deep learning methods, shall be added.
As you suggest, we have added recent works to our introduction and comparison.
“Edge” feature definition in Algorithm 6 is ambiguous and lacking explanation for its rationale.
In order to avoid the ambiguity, we have explained more detailed Algorithm 6 by adding comments in each line.
Size of the dataset, and details of training/testing split shall be provided.
Indeed, we updated Section 2.1 to detailed data about the dataset setup.
In caption of Fig.11, name of the methods for comparison shall be provided along with their reference.
Thank you very much for the comments. We have updated the Fig. 11 and now more information is provided.
Reviewer 2 Report
As the Authors state in this paper, the incidence of melanoma is increasing in most developed countries in the word. This has been associated with an increase also in mortality, which is clearly related to the stage at which the melanoma is diagnosed and treated. This paper describes a very technological approach to improve the diagnosis of pigmented skin lesions.
The authors used sophisticated methods, features extraction and refined statistical analysis.
INTRODUCTION
Pag 1 lines 30-31. The Author reports: “In medical centers, the conventional tools used to diagnose melanomas are the dermatoscope and the Fotofinder”.
First of all the authors should be less specific!!! Delete Fotofinder (which is the brand of an instrument and not a method) and substitute with digital dermoscopy analysis. Moreover to introduce the topic he has to make a sentence like:
“In recent years, non-invasive diagnostic techniques for the diagnosis of melanoma have been developing very quickly. These include dermatoscopy, digital dermoscopic analysis, confocal microscopy and LC-OCT (Add these references: Rubegni P, et al. Computer-assisted melanoma diagnosis: a new integrated system. Melanoma Res. 2015 Dec; 25 (6): 537-42 .; Dubois A et al. Line-field confocal optical coherence tomography for high-resolution noninvasive imaging of skin tumors. Journal of Biomedical Optics (23) 10, 1 October 2018, Article number 106007 and Cinotti et al. Dermoscopy vs. reflectance confocal microscopy for the diagnosis of lentigo maligna. Journal of the European Academy of Dermatology and Venereology. Volume 32, Issue 8, August 2018, Pages 1284-1291). Among them, the one most certainly used is dermatoscopy.
Pag. 2 lines 56-78 and Table 1. In this part of the introduction the author makes his own and subjective story of the various researches on the topic starting from 2011. In particular research on computer assisted diagnosis techniques start much earlier. In the early 2000s Elbaum published an article that deserves to be mentioned absolutely (Elbaum M, Kopf AW, Rabinovitz HS, Langley RG, Kamino H, Mihm MC Jr, Sober AJ, Peck GL, Bogdan A, Gutkowicz-Krusin D, Greenebaum M, Keem S, Oliviero M, Wang S. Automatic differentiation of melanoma from melanocytic nevi with multispectral digital dermoscopy: a feasibility study. J Am Acad Dermatol. 2001 Feb; 44 (2): 207-1),
The author must add these authors in the bibliography and rebuilt the history of non-invasive diagnosis and melanoma in a slightly more objective way.
MATERIAL AND METHODS
The Author includes in the study lesions obtained from 3 large datasets but we do not know how many and what type of melanoma the included? (superficial spreading, nodular, acral achromic) and control lesions? Are they equivocal benign nevi (dysplastic nevi Reed nevi, Spitz nevi etc etc) and/or are they other benign non melanocytic skin lesions (seborrheic keratoses or pigmented basal cell carcinoma)? Authors should keep in mind that despite the fact that these lesions can be sometimes difficult to distinguish from melanoma from a clinical point of view, thanks to dermoscopy, today they can be easily distinguished in a few seconds. Here comes the second important issue: the Authors in their study obtained a good value of diagnostic accuracy. However, their diagnostic system is not compared to simple clinical/dermoscopic assessment of the skin lesions: in other words if a dermatologist evaluates for comparison the lesion studied in the paper and he obtain a diagnostic accuracy of 100% how would the Authors judge their results? Pag 11 line 206: the Author write benign and malignant melanoma. Please correct probably…. “melanocytic lesions”? Pag 13 line 227 … AGAIN!
All these concept must be reported in the paper in term of limitations of the study or in the discussion section
In conclusion we believe that the methodology used is interesting but the author should collaborate with clinicians in order to address the problem in a rational way. For example, I believe that might be interesting to test their method of amelanotic and or featureless melanomas in order to evaluate if they are able to help the clinician in recognizing this kind of terrible skin neoplasm.
Author Response
Reviewer 2
As the Authors state in this paper, the incidence of melanoma is increasing in most developed countries in the word. This has been associated with an increase also in mortality, which is clearly related to the stage at which the melanoma is diagnosed and treated. This paper describes a very technological approach to improve the diagnosis of pigmented skin lesions.
The authors used sophisticated methods, features extraction and refined statistical analysis.
INTRODUCTION
Pag 1 lines 30-31. The Author reports: “In medical centers, the conventional tools used to diagnose melanomas are the dermatoscope and the Fotofinder”.
First of all the authors should be less specific!!! Delete Fotofinder (which is the brand of an instrument and not a method) and substitute with digital dermoscopy analysis. Moreover to introduce the topic he has to make a sentence like:
“In recent years, non-invasive diagnostic techniques for the diagnosis of melanoma have been developing very quickly. These include dermatoscopy, digital dermoscopic analysis, confocal microscopy and LC-OCT (Add these references: Rubegni P, et al. Computer-assisted melanoma diagnosis: a new integrated system. Melanoma Res. 2015 Dec; 25 (6): 537-42 .; Dubois A et al. Line-field confocal optical coherence tomography for high-resolution noninvasive imaging of skin tumors. Journal of Biomedical Optics (23) 10, 1 October 2018, Article number 106007 and Cinotti et al. Dermoscopy vs. reflectance confocal microscopy for the diagnosis of lentigo maligna. Journal of the European Academy of Dermatology and Venereology. Volume 32, Issue 8, August 2018, Pages 1284-1291). Among them, the one most certainly used is dermatoscopy.
Thank you very much for the comments. We have substituted the word Fotofinder as you suggest. Additionally, we have included the proposed references since we think to contribute to making the manuscript more readable.
Pag. 2 lines 56-78 and Table 1. In this part of the introduction the author makes his own and subjective story of the various researches on the topic starting from 2011. In particular research on computer assisted diagnosis techniques start much earlier. In the early 2000s Elbaum published an article that deserves to be mentioned absolutely (Elbaum M, Kopf AW, Rabinovitz HS, Langley RG, Kamino H, Mihm MC Jr, Sober AJ, Peck GL, Bogdan A, Gutkowicz-Krusin D, Greenebaum M, Keem S, Oliviero M, Wang S. Automatic differentiation of melanoma from melanocytic nevi with multispectral digital dermoscopy: a feasibility study. J Am Acad Dermatol. 2001 Feb; 44 (2): 207-1),
The author must add these authors in the bibliography and rebuilt the history of non-invasive diagnosis and melanoma in a slightly more objective way.
Thank you for your suggestions. Based on your comment we have included the references you suggest.
MATERIAL AND METHODS
The Author includes in the study lesions obtained from 3 large datasets but we do not know how many and what type of melanoma the included? (superficial spreading, nodular, acral achromic) and control lesions? Are they equivocal benign nevi (dysplastic nevi Reed nevi, Spitz nevi etc etc) and/or are they other benign non melanocytic skin lesions (seborrheic keratoses or pigmented basal cell carcinoma)? Authors should keep in mind that despite the fact that these lesions can be sometimes difficult to distinguish from melanoma from a clinical point of view, thanks to dermoscopy, today they can be easily distinguished in a few seconds.
We really appreciate your comments. The authors of the used databases do not provide additional data about the melanocytic lesions, however, due to the outstanding results in the future, we pretend to obtain data with more detailed labels.
Here comes the second important issue: the Authors in their study obtained a good value of diagnostic accuracy. However, their diagnostic system is not compared to simple clinical/dermoscopic assessment of the skin lesions: in other words if a dermatologist evaluates for comparison the lesion studied in the paper and he obtain a diagnostic accuracy of 100% how would the Authors judge their results?
Since the used datasets are supported by histopathology tests and dermatologists specialized in melanoma, and the datasets are widely accepted by the literature, we think are results gives a reasonable performance reference.
Pag 11 line 206: the Author write benign and malignant melanoma. Please correct probably…. “melanocytic lesions”? Pag 13 line 227 … AGAIN!
Thank you for your suggestion. We have corrected our mistake.
In conclusion we believe that the methodology used is interesting but the author should collaborate with clinicians in order to address the problem in a rational way. For example, I believe that might be interesting to test their method of amelanotic and or featureless melanomas in order to evaluate if they are able to help the clinician in recognizing this kind of terrible skin neoplasm.
We really appreciate your valuable comments. Although our vision with this work is to propose a novel method and we have validated our results with public datasets, we support your comment. Therefore, in our future work, we will work with clinicians to realize the experiments you suggest.
Reviewer 3 Report
In the paper, the authors proposed a method that combines mathematical morphology with a multilayer perceptron for melanoma detection. And the final test results also show that the method has a high accuracy rate. Their research provides a certain computer-aided method for the accurate detection of melanoma, which is of great significance. However, I still have some suggestions for the authors to improve the manuscript:
When four descriptors are input to a multilayer perceptron, the input form of the descriptors and the role of the hidden layer should be described in detail. In fig (5), the mentioned m should give an explanation Please briefly introduce the intent of converting RGB images into GRAY in the paper On line 219 of the paper, the author should unify the number format and change 1 to one. On line 220 of the paper, when the malignant melanoma severity level is divided by different colors, both level one and level three indicate less than 0.85, resulting in the blurred division. Please modify it. On line 178 of the paper, the activation function used in the last layer should correspond to the following content.Author Response
Reviewer3
In the paper, the authors proposed a method that combines mathematical morphology with a multilayer perceptron for melanoma detection. And the final test results also show that the method has a high accuracy rate. Their research provides a certain computer-aided method for the accurate detection of melanoma, which is of great significance. However, I still have some suggestions for the authors to improve the manuscript:
When four descriptors are input to a multilayer perceptron, the input form of the descriptors and the role of the hidden layer should be described in detail.
Thank you for your suggestion. Now we have included two new sections to describe more detailed the information about the multilayer perceptron and descriptors as you suggest.
In fig (5), the mentioned m should give an explanation
Thank you for your feedback. In the latest version of the manuscript, we gave an explanation of Fig. 5.
Please briefly introduce the intent of converting RGB images into GRAY in the paper
A brief explanation of conversion from RGB to GRAY scale is described in the Introduction section by taking into account the reference 31.
On line 219 of the paper, the author should unify the number format and change 1 to one.
It has been corrected.
On line 220 of the paper, when the malignant melanoma severity level is divided by different colors, both level one and level three indicate less than 0.85, resulting in the blurred division. Please modify it.
It has been corrected the mistake.
On line 178 of the paper, the activation function used in the last layer should correspond to the following content.
Thank you for your comment. We have corrected it.
Round 2
Reviewer 1 Report
All of my concerns have been addressed by the response. In addition to further polishment of the language, image quality of Figure 3 and Figure 7 (the two illustrative figures) needs to be improved. It is highly suggested that authors should refer to previous literature in deep learning field of how to illustrate their analytics pipeline and network structure.
Author Response
Thank you for your feedback. A native English Speaker has checked the language. Images have been improved. Certain references have been added regarding deep learning.
Reviewer 2 Report
perfect
Author Response
thank you for your comments.